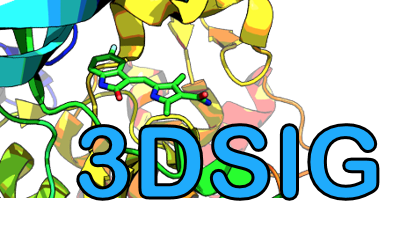
3DSIG
On the reliability and the limits of inference of amino acid sequence alignments
- Dinithi Sumanaweera, Wellcome Sanger Institute, United Kingdom
- Lloyd Allison, Monash University, Australia
- Arun Konagurthu, Monash University, Australia
- Sandun Rajapaksa, Monash University, Australia
- Arthur Lesk, Pennsylvania State University, United States
- Peter Stuckey, Monash University, Australia
- Maria Garcia de la Banda, Monash University, Australia
- David Abramson, University of Queensland, Australia
Presentation Overview: Show
Motivation: Alignments are correspondences between sequences. How reliable are alignments of amino-acid sequences of proteins, and what inferences about protein relationships can be drawn? Using techniques not previously applied to these questions, by weighting every possible sequence alignment by its posterior probability we derive a formal mathematical expectation, and develop an efficient algorithm for computation of the distance between alternative alignments allowing quantitative comparisons of sequence-based alignments with corresponding reference structure alignments.
Results: By analyzing the sequences and structures of one million protein domain pairs, we report the variation of the expected distance between sequence-based and structure-based alignments, as a function of (Markov time of) sequence divergence. Our results clearly demarcate the `daylight', `twilight' and `midnight' zones for interpreting residue-residue correspondences from sequence information alone.
Topsy-Turvy: integrating a global view into sequence-based PPI prediction
- Rohit Singh, Massachusetts Institute of Technology, United States
- Kapil Devkota, Tufts University, United States
- Samuel Sledzieski, Massachusetts Institute of Technology, United States
- Bonnie Berger, Massachusetts Institute of Technology, United States
- Lenore Cowen, Tufts University, United States
Presentation Overview: Show
Computational methods to predict protein-protein interaction (PPI) typically segregate into sequence-based ""bottom-up"" methods that infer properties from the characteristics of the individual protein sequences, or global ""top-down"" methods that infer properties from the pattern of already known PPIs in the species of interest. However, a way to incorporate top-down insights into sequence-based bottom-up PPI prediction methods has been elusive. We thus introduce Topsy-Turvy, a method that newly synthesizes both views in a sequence-based, multi-scale, deep-learning model for PPI prediction. While Topsy-Turvy makes predictions using only sequence data, during the training phase it takes a transfer-learning approach by incorporating patterns from both global and molecular-level views of protein interaction. In a cross-species context, we show it achieves state-of-the-art performance, offering the ability to perform genome-scale, interpretable PPI prediction for non-model organisms with no existing experimental PPI data. In species with available experimental PPI data, we further present a Topsy-Turvy hybrid (TT-Hybrid) model which integrates Topsy-Turvy with a purely network-based model for link prediction that provides information about species-specific network rewiring. TT-Hybrid makes accurate predictions for both well- and sparsely-characterized proteins, outperforming both its constituent components as well as other state-of-the-art PPI prediction methods. Furthermore, running Topsy-Turvy and TT-Hybrid screens is feasible for whole genomes, and thus these methods scale to settings where other methods (e.g., AlphaFold-Multimer) might be infeasible. The generalizability, accuracy and genome-level scalability of Topsy-Turvy and TT-Hybrid unlocks a more comprehensive map of protein interaction and organization in both model and non-model organisms.
Software availability: https://topsyturvy.csail.mit.edu
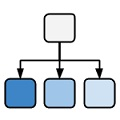
Bio-Ontologies
DeepGOZero: Improving protein function prediction from sequence and zero-shot learning based on ontology axioms
- Maxat Kulmanov, King Abdullah University of Science and Technology, Saudi Arabia
- Robert Hoehndorf, King Abdullah University of Science and Technology, Saudi Arabia
Presentation Overview: Show
Motivation: Protein functions are often described
using the Gene Ontology (GO) which is an ontology consisting of over
50,000 classes and a large set of formal axioms. Predicting the
functions of proteins is one of the key challenges in computational
biology and a variety of machine learning methods have been
developed for this purpose. However, these methods usually require
significant amount of training data and cannot make predictions for
GO classes which
have only few or no experimental annotations.
Results: We developed DeepGOZero, a machine learning model
which improves predictions for functions with no or only a small
number of annotations. To achieve this goal, we rely on a
model-theoretic approach for learning ontology embeddings and
combine it with neural networks for protein function
prediction. DeepGOZero can exploit formal axioms in the GO to make
zero-shot predictions, i.e., predict protein functions even if not a
single protein in the training phase was associated with that
function. Furthermore, the zero-shot prediction method employed by
DeepGOZero is generic and can be applied whenever associations with
ontology classes need to be predicted.
Exploring Automatic Inconsistency Detection for Literature-based Gene Ontology Annotation
- Jiyu Chen, The University of Melbourne, Australia
- Benjamin Goudey, The University of Melbourne, Australia
- Justin Zobel, The University of Melbourne, Australia
- Nicholas Geard, The University of Melbourne, Australia
- Karin Verspoor, RMIT University, Australia
Presentation Overview: Show
Motivation: Literature-based Gene Ontology Annotations (GOA) are biological database records that use controlled vocabulary to uniformly represent gene function information that is described in primary literature. Assurance of the quality of GOA is crucial for supporting biological research. However, a range of different kinds of inconsistencies in between literature as evidence and annotated GO terms can be identified; these have not been systematically studied at record level. The existing manual-curation approach to GOA consistency assurance is inefficient and is unable to keep pace with the rate of updates to gene function knowledge. Automatic tools are therefore needed to assist with GOA consistency assurance. This paper presents an exploration of different GOA inconsistencies and an early feasibility study of automatic inconsistency detection.
Results: We have created a reliable synthetic dataset to simulate four realistic types of GOA inconsistency in biological databases. Three automatic approaches are proposed. They provide reasonable performance on the task of distinguishing the four types of inconsistency and are directly applicable to detect inconsistencies in real-world GOA database records. Major challenges resulting from such inconsistencies in the context of several specific application settings are reported.
Conclusion: This is the first study to introduce automatic approaches that are designed to address the challenges in current GOA quality assurance workflows.
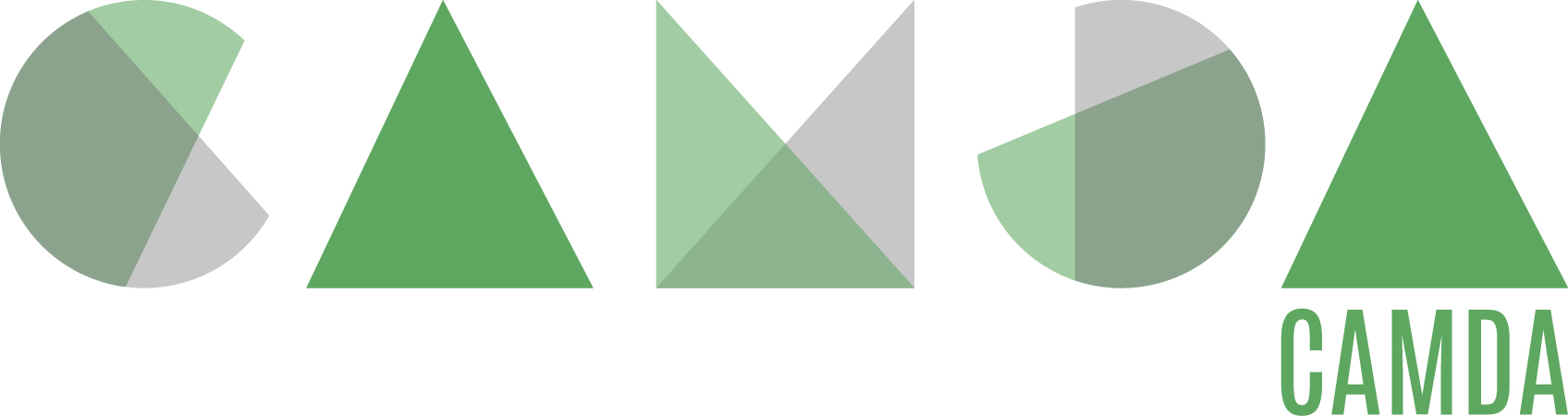
CAMDA
High-sensitivity pattern discovery in large, paired multi-omic datasets
- Andrew Ghazi, Broad Institute, United States
- Kathleen Sucipto, Harvard T.H. Chan School of Public Health, United States
- Ali Rahnavard, Harvard T.H. Chan School of Public Health, United States
- Eric Franzosa, Harvard T.H. Chan School of Public Health, United States
- Lauren McIver, Harvard T.H. Chan School of Public Health, United States
- Jason Lloyd-Price, Harvard T.H. Chan School of Public Health, United States
- Emma Schwager, Harvard T.H. Chan School of Public Health, United States
- George Weingart, Harvard T.H. Chan School of Public Health, United States
- Yo Sup Moon, Harvard T.H. Chan School of Public Health, United States
- Xochitl Morgan, University of Otago, United States
- Levi Waldron, City University of New York Graduate School of Public Health and Health Policy, United States
- Curtis Huttenhower, Harvard T.H. Chan School of Public Health, United States
Presentation Overview: Show
Motivation: Modern biological screens yield enormous numbers of measurements, and identifying and interpreting statistically significant associations among features is essential. In experiments featuring multiple high-dimensional datasets collected from the same set of samples, it is useful to identify groups of associated features between the datasets in a way that provides high statistical power and false discovery rate control.
Results: Here, we present a novel hierarchical framework, HAllA (Hierarchical All-against-All associa-tion testing), for structured association discovery between paired high-dimensional datasets. HAllA efficiently integrates hierarchical hypothesis testing with false discovery rate correction to reveal signif-icant linear and non-linear block-wise relationships among continuous and/or categorical data. We optimized and evaluated HAllA using heterogeneous synthetic datasets of known association struc-ture, where HAllA outperformed all-against-all and other block testing approaches across a range of common similarity measures. We then applied HAllA to a series of real-world multi-omics datasets, revealing new associations between gene expression and host immune activity, the microbiome and host transcriptome, metabolomic profiling, and human health phenotypes.
Availability: An open-source implementation of HAllA is freely available at http://huttenhower.sph.harvard.edu/halla along with documentation, demo datasets, and a user group.
Phage-bacteria contig association prediction with a convolutional neural network
- Tianqi Tang, Department of Quantitative and Computational Biology, University of Southern California, United States
- Shengwei Hou, Department of Ocean Science and Engineering, Southern University of Science and Technology, China
- Jed Fuhrman, Marine and Environmental Biology, Department of Biological Sciences, University of Southern California, United States
- Fengzhu Sun, Department of Quantitative and Computational Biology, University of Southern California, United States
Presentation Overview: Show
Motivation: Phage-host associations play important roles in microbial communities.
But in natural communities, as opposed to culture-based lab studies where phages are discovered and characterized metagenomically, their hosts are generally not known. Several programs have been developed for predicting which phage infects which host based on various sequence similarity measures or machine learning approaches.
These are often based on whole viral and host genomes, but in metagenomics-based studies we rarely have whole genomes but rather must rely on contigs that are sometimes as short as hundreds of bp long.
Therefore, we need programs that predict hosts of phage contigs on the basis of these short contigs.
Although most existing programs can be applied to metagenomic datasets for these predictions, their accuracies are generally low. Here we develop ContigNet, a convolutional neural network based model capable of predicting phage-host matches based on relatively short contigs,and compare to previously published VirHostMatcher and WIsH.
Results: On the validation set, ContigNet achieves 72-85% area under the receiver operating characteristic curve (AUROC) scores, compared to the maximum of 68\% by VirHostMatcher or WIsH for contigs of lengths between 200 bps to 50 kbps.
We also apply the model to the Metagenomic Gut Virus (MGV) catalogue, a dataset containing a wide range of draft genomes from metagenomic samples and achieved 60-70% AUROC scores while VirHostMatcher and WIsH fulfilled 52%.
Surprisingly, ContigNet can also be used to predict plasmid-host contig associations with high accuracy, indicating a similar genetic exchange between mobile genetic elements and their hosts.
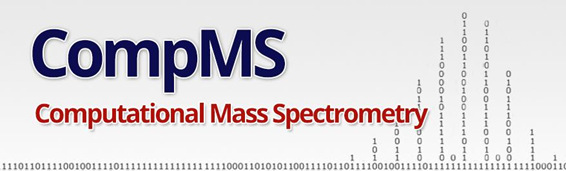
CompMS
Deep kernel learning improves molecular fingerprint prediction from tandem mass spectra
- Kai Dührkop, Friedrich-Schiller-University Jena, Germany
Presentation Overview: Show
Untargeted metabolomics experiments rely on spectral libraries for structure
annotation, but these libraries are vastly incomplete; in-silico methods
search in structure databases, allowing us to overcome this limitation. The best-performing in-silico methods
use machine learning to predict a molecular fingerprint from tandem mass spectra, then use the predicted fingerprint to search in a molecular structure database. Predicted molecular fingerprints are also of great interest for compound class annotation, de novo structure elucidation, and other tasks. So far, Kernel support vector machines are the best tool for fingerprint prediction. However, they cannot be trained on all publicly available reference spectra because their training time scales cubically with the number of training data.
Here, we use the Nystrom approximation to transform the kernel into a linear feature map. We evaluate two methods that use this feature map as input: a linear SVM and a deep neural network.
For evaluation we use a cross-validated dataset of 156,017 compounds and three independent datasets with 1,734 compounds. We show that the combination of kernel method and deep neural network outperforms the kernel support vector machine, which is the current gold-standard, as well as a deep neural network on tandem mass spectra on all evaluation datasets.
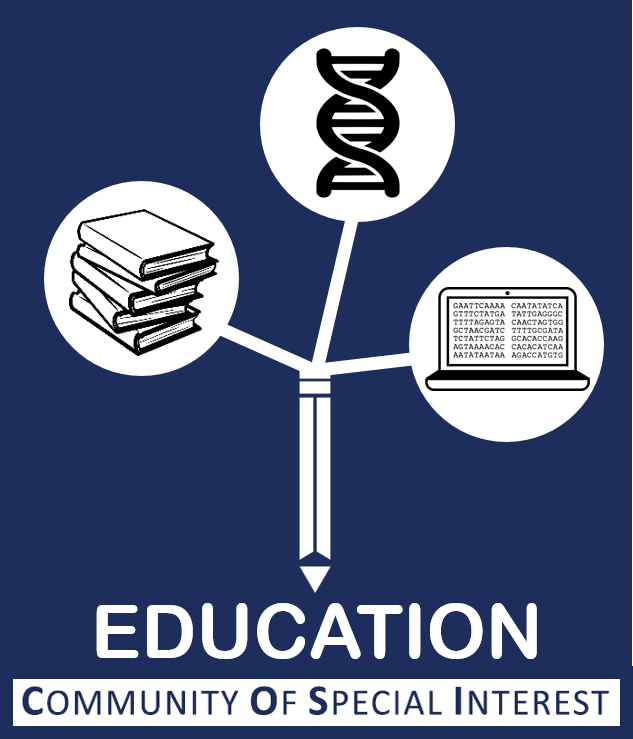
Education
An approachable, flexible, and practical machine learning workshop for biologists
- Fangzhou Mu, University of Wisconsin-Madison, United States
- Rosemary Russ, University of Wisconsin-Madison, United States
- Milica Cvetkovic, University of Wisconsin-Madison, United States
- Debora Treu, University of Wisconsin-Madison, United States
- Anthony Gitter, University of Wisconsin-Madison, United States
- Christopher Magnano, University of Madison-Wisconsin, United States
Presentation Overview: Show
The increasing prevalence and importance of machine learning in biological research has created a need for machine learning training resources tailored towards biological researchers.
However, existing resources are often inaccessible, infeasible, or inappropriate for biologists because they require significant computational and mathematical knowledge, demand an unrealistic time-investment, or teach skills primarily for computational researchers.
We created the Machine Learning for Biologists (ML4Bio) workshop, a short, intensive workshop that empowers biological researchers to comprehend machine learning applications and pursue machine learning collaborations in their own research.
The ML4Bio workshop focuses on classification and was designed around 3 principles: (a) focusing on preparedness over fluency or expertise, (b) necessitating minimal coding and mathematical background, and (c) requiring low time investment.
It incorporates active learning methods and custom open source software that allows participants to explore machine learning workflows.
After multiple sessions to improve workshop design, we performed a study on 3 workshop sessions.
Despite some confusion around identifying subtle methodological flaws in machine learning workflows, participants generally reported that the workshop met their goals, provided them with valuable skills and knowledge, and greatly increased their beliefs that they could engage in research that uses machine learning.
ML4Bio is an educational tool for biological researchers, and its creation and evaluation provides valuable insight into tailoring educational resources for active researchers in different domains.
Characterizing domain-specific open educational resources by linking ISCB Communities of Special Interest to Wikipedia
- Alastair M. Kilpatrick, Centre for Regenerative Medicine, University of Edinburgh, UK, United Kingdom
- Farzana Rahman, School of Mathematics, Computer Science and Engineering, City, University of London, UK, United Kingdom
- Audra Anjum, Office of Instructional Innovation, Ohio University, USA, United States
- Sayane Shome, Department of Anesthesiology, Perioperative and Pain Medicine, Stanford School of Medicine, Stanford University, USA, United States
- K.M. Salim Andalib, Biotechnology and Genetic Engineering Discipline, Khulna University, Khulna, Bangladesh, Bangladesh
- Shrabonti Banik, Faculty of Veterinary, Animal and Biomedical Sciences, Sylhet Agricultural University, Sylhet, Bangladesh, Bangladesh
- Sanjana F. Chowdhury, Department of Genetic Engineering and Biotechnology, Shahjalal University of Science and Technology, Sylhet, Bangladesh, Bangladesh
- Peter Coombe, Wikipedia volunteer, United Kingdom
- Yesid Cuesta Astroz, Colombian Institute of Tropical Medicine, CES University, Medellín, Colombia, Colombia
- J. Maxwell Douglas, Department of Molecular Oncology, BC Cancer Agency, Vancouver, BC, Canada, Canada
- Pradeep Eranti, UMRS-1124, INSERM, Université de Paris, Paris, France, France
- Aleyna D. Kıran, Department of Bioengineering, Ege University, Turkey, Turkey
- Sachendra Kumar, IISc Mathematics Initiative, Indian Institute of Science, Bengaluru, India, India
- Hyeri Lim, Department of Biomedical Data Intelligence, Graduate School of Medicine, Kyoto University, Kyoto, Japan, Japan
- Valentina Lorenzi, Wellcome Sanger Institute, Hinxton, Cambridge, UK; European Bioinformatics Institute (EMBL-EBI), Hinxton, UK, United Kingdom
- Tiago Lubiana, School of Pharmaceutical Sciences, University of São Paulo, São Paulo, Brazil, Brazil
- Sakib Mahmud, Biotechnology and Genetic Engineering Discipline, Khulna University, Khulna, Bangladesh, Bangladesh
- Rafael Puche, Genetics and Forensic Studies Unit (UEGF), Venezuelan Institute of Scientific Research (IVIC), Venezuela, Venezuela
- Agnieszka Rybarczyk, Institute of Computing Science, Poznan University of Technology, Poznan, Poland, Poland
- Syed Muktadir Al Sium, Institute of Epidemiology, Disease Control And Research, Dhaka, Bangladesh, Bangladesh
- David Twesigomwe, Sydney Brenner Institute for Molecular Bioscience (SBIMB), University of the Witwatersrand, Johannesburg, South Africa, South Africa
- Tomasz Zok, Institute of Computing Science, Poznan University of Technology, Poznan, Poland, Poland
- Christine A. Orengo, Institute of Structural and Molecular Biology, University College London, London WC1E 6BT, UK, United Kingdom
- Iddo Friedberg, Program in Bioinformatics and Computational Biology, Iowa State University, Ames, IA, USA, United States
- Janet F. Kelso, Max Planck Institute for Evolutionary Anthropology, Leipzig, Germany, Germany
- Lonnie Welch, School of Electrical Engineering and Computer Science, Ohio University, USA, United States
Presentation Overview: Show
Motivation: Wikipedia is one of the most important channels for the public communication of science and is frequently accessed as an educational resource in computational biology. Joint efforts between the International Society for Computational Biology (ISCB) and the Computational Biology taskforce of WikiProject Molecular Biology (a group of expert Wikipedia editors) have considerably improved computational biology representation on Wikipedia in recent years. However, there is still an urgent need for further improvement in quality, especially when compared to related scientific fields such as genetics and medicine. Facilitating involvement of members from ISCB Communities of Special Interest (COSIs) would improve a vital open education resource in computational biology, additionally allowing COSIs to provide a quality educational resource highly specific to their subfield.
Results: We generate a list of around 1,500 English Wikipedia articles relating to computational biology and describe the development of a binary COSI-Article matrix, linking COSIs to relevant articles and thereby defining domain-specific open educational resources. Our analysis of the COSI-Article matrix data provides a quantitative assessment of computational biology representation on Wikipedia against other fields and at a COSI-specific level. Furthermore, we conducted similarity analysis and subsequent clustering of COSI-Article data to provide insight into potential relationships between COSIs. Finally, based on our analysis, we suggest courses of action to improve the quality of computational biology representation on Wikipedia, enhancing this educational resource for all parties.
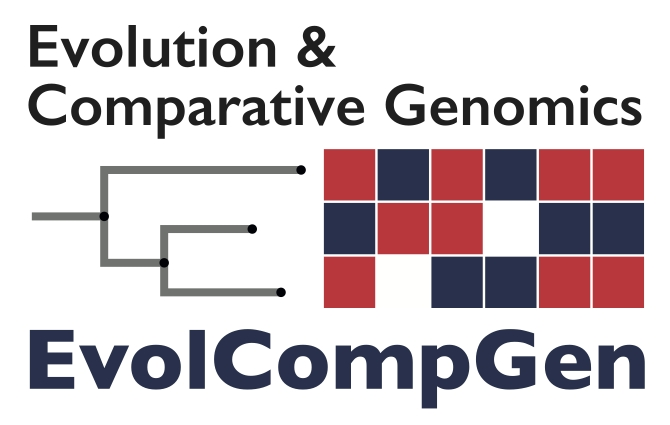
EvolCompGen
A LASSO-based approach to sample sites for phylogenetic tree search
- Noa Ecker, Tel Aviv University, Israel
- Dana Azouri, Tel Aviv University, Israel
- Ben Bettisworth, Heidelberg Institute for Theoretical Studies, Germany
- Alexandros Stamatakis, Heidelberg Institute for Theoretical Studies, Germany
- Yishay Mansour, Tel Aviv University, Israel
- Itay Mayrose, Tel Aviv University, Israel
- Tal Pupko, Tel Aviv University, Israel
Presentation Overview: Show
In recent years, full-genome sequences have become increasingly available and as a result many modern phylogenetic analyses are based on very long sequences, often with over 100,000 sites. Phylogenetic reconstructions of large-scale alignments are challenging for likeli-hood based phylogenetic inference programs and usually require using a powerful computer clus-ter. Current tools for alignment trimming prior to phylogenetic analysis do not promise a signifi-cant reduction in the alignment size and are claimed to have a negative effect on the accuracy of the obtained tree.
Here, we propose an artificial-intelligence based approach, which provides means to select the optimal subset of sites and a formula by which one can compute the log-likelihood of the entire data based on this subset. Our approach is based on training a regularized Lasso-regression model that optimizes the log-likelihood prediction accuracy while putting a constraint on the number of sites used for the approximation. We show that computing the likelihood based on 5% of the sites already provides accurate approximation of the tree likelihood based on the entire data. Furthermore, we show that using this Lasso-based approximation during a tree search decreased running-time substantially while retaining the same tree-search performance.
Bridging the gaps in statistical models of protein alignment
- Dinithi Sumanaweera, Wellcome Sanger Institute, United Kingdom
- Lloyd Allison, Monash University, Australia
- Arun Konagurthu, Monash University, Australia
Presentation Overview: Show
Sequences of proteins evolve by accumulating substitutions together with insertions and deletions (indels) of amino acids. However, it remains a common practice to disconnect substitutions and indels, and infer approximate models for each of them separately, to quantify sequence relationships. Although this approach brings with it computational convenience (which remains its primary motivation), there is a dearth of attempts to unify and model them systematically and together. To overcome this gap, this paper demonstrates how a complete statistical model quantifying the evolution of pairs of aligned proteins can be constructed using a time-parameterised substitution matrix and a time-parameterised alignment state machine. Methods to derive all parameters of such a model from any benchmark collection of aligned protein sequences are described here. This has not only allowed us to generate a unified statistical model for each of the nine widely-used substitution matrices (PAM, JTT, BLOSUM, JO, WAG, VTML, LG, MIQS, and PFASUM), but also resulted in a new unified model, MMLSUM. Our underlying methodology measures the Shannon information content using each model to explain losslessly any given collection of alignments, which has allowed us to quantify the performance of all the above models on six comprehensive alignment benchmarks. Our results show that MMLSUM results in a new and clear overall best performance, followed by PFASUM, VTML, BLOSUM and MIQS, respectively, amongst the top five. We further analyse the statistical properties of MMLSUM model and contrast it with others.
Phylovar: Towards scalable phylogeny-aware inference of single-nucleotide variations from single-cell DNA sequencing data
- Mohammadamin Edrisi, Rice University, United States
- Monica Valecha, University of Vigo, Spain
- Sunkara B. V. Chowdary, Indian Institute of Technology Kanpur, India
- Sergio Robledo, University of Houston, United States
- Huw Ogilvie, Rice University, United States
- David Posada, University of Vigo, Spain
- Hamim Zafar, Indian Institute of Technology Kanpur, United States
- Luay Nakhleh, Rice University, United States
Presentation Overview: Show
Single-nucleotide variants (SNVs) are the most common variations in the human genome. Recently developed methods for SNV detection from single-cell DNA sequencing (scDNAseq) data, such as SCIΦ and scVILP, leverage the evolutionary history of the cells to overcome the technical errors associated with single-cell sequencing protocols. Despite being accurate, these methods are not scalable to the extensive genomic breadth of single-cell whole-genome (scWGS) and whole-exome sequencing (scWES) data.
Here we report on a new scalable method, Phylovar, which extends the phylogeny-guided variant calling approach to sequencing datasets containing millions of loci. Through benchmarking on simulated datasets under different settings, we show that, Phylovar outperforms SCIΦ in terms of running time while being more accurate than Monovar (which is not phylogeny-aware) in terms of SNV detection. Furthermore, we applied Phylovar to two real biological datasets: an scWES triple-negative breast cancer data consisting of 32 cells and 3375 loci as well as an scWGS data of neuron cells from a normal human brain containing 16 cells and approximately 2.5 million loci. For the cancer data, Phylovar detected somatic SNVs with high or moderate functional impact that were also supported by bulk sequencing dataset and for the neuron dataset, Phylovar identified 5745 SNVs with non-synonymous effects some of which were associated with neurodegenerative diseases. We implemented Phylovar and made it publicly available at https://github.com/mae6/Phylovar.git.
QuCo: Quartet-based Co-estimation of Species Trees and Gene Trees
- Siavash Mirarab, University of California San Diego, United States
- Maryam Rabiee, University of California San Diego, United States
Presentation Overview: Show
Motivation: Phylogenomics faces a dilemma: on the one hand, most accurate species and gene tree estimation methods are those that co-estimate them; on the other hand, these co-estimation methods do not scale to moderately large numbers of species. The summary-based methods, which first infer gene trees independently and then combine them, are much more scalable but are prone to gene tree estimation error, which is inevitable when inferring trees from limited-length data. Gene tree estimation error is not just random noise and can create biases such as long-branch attraction. Co-estimating gene trees and the species tree is known to reduce the gene tree error.
Results: We introduce a scalable likelihood-based approach to co-estimation under the multi-species coalescent model. The method, called Quartet Coestimation (QuCo), takes as input independently inferred distributions over gene trees and computes the most likely species tree topology and internal branch length for each quartet, marginalizing over gene tree topologies. It then updates the gene tree posterior probabilities based on the species tree. The focus on gene tree topologies and the heuristic division to quartets enables fast likelihood calculations.
We benchmark our method with extensive simulations for quartet trees in zones known to produce biased species trees and further with larger trees. We also run QuCo on a biological dataset of bees. Our results show better accuracy than the summary-based approach ASTRAL run on estimated gene trees.
Quintet Rooting: Rooting Species Trees under the Multi-Species Coalescent Model
- Yasamin Tabatabaee, University of Illinois at Urbana-Champaign, United States
- Kowshika Sarker, University of Illinois at Urbana-Champaign, United States
- Tandy Warnow, University of Illinois at Urbana-Champaign, United States
Presentation Overview: Show
Rooted species trees are a basic model with multiple applications throughout biology,
including understanding adaptation, biodiversity, phylogeography, and co-evolution. Because most species tree estimation methods produce unrooted trees, methods for rooting these trees have been developed. However, most rooting methods either rely on prior biological knowledge or assume that evolution is close to clock-like, which is not usually the case. Furthermore, most prior rooting methods do not account for biological processes that create discordance between gene trees and species trees.
Results: We present Quintet Rooting, a polynomial time method for rooting species trees from multi-locus datasets, which is based on a proof of identifiability of the rooted species tree under the multi-species coalescent (MSC) model established by Allman, Degnan, and Rhodes (J Math Biol, 2011). Our simulation study shows that Quintet Rooting is generally more accurate than other rooting methods, except under extreme levels of gene tree estimation error or when the number of genes is very small.
Availability and implementation: Quintet Rooting is available in open source form at https://github.com/ytabatabaee/Quintet-Rooting.
Links to datasets used in this study are available at https://tandy.cs.illinois.edu/datasets.html
Reconstructing tumor clonal lineage trees incorporating single nucleotide variants, copy number alterations, and structural variations
- Xuecong Fu, Carnegie Mellon University, United States
- Haoyun Lei, Carnegie Mellon University, United States
- Yifeng Tao, Carnegie Mellon University, United States
- Russell Schwartz, Carnegie Mellon University, United States
Presentation Overview: Show
Cancer develops through a process of clonal evolution in which an initially healthy cell gives rise to progeny gradually differentiating through the accumulation of genetic and epigenetic mutations. These mutations can take various forms, including single nucleotide variants (SNVs), copy number alterations (CNAs), or structural variations (SVs), with each variant type providing complementary insights into tumor evolution as well as offering distinct challenges to phylogenetic inference. In the present work, we develop a tumor phylogeny method, TUSV-ext, that incorporates SNVs, CNAs, and SVs into a single inference framework. We demonstrate on simulated data that the method produces accurate tree inferences in the presence of all three variant types. We further demonstrate the method through application to real prostate tumor data, showing how our approach to coordinated phylogeny inference and clonal construction with all three variant types can reveal a more complicated clonal structure than is suggested by prior work, consistent with extensive polyclonal seeding or migration.
Simulating Domain Architecture Evolution
- Xiaoyue Cui, Carnegie Mellon University, United States
- Yifan Xue, Carnegie Mellon University, United States
- Collin McCormack, Carnegie Mellon University, United States
- Alejandro Garces, Carnegie Mellon University, United States
- Thomas Rachman, Carnegie Mellon University, United States
- Yang Yi, Carnegie Mellon University, United States
- Maureen Stolzer, Carnegie Mellon University, United States
- Dannie Durand, Carnegie Mellon University, United States
Presentation Overview: Show
Simulation is an essential technique for generating biomolecular data with a “known” history for use in validating phylogenetic inference and other evolutionary methods. On longer time scales, simulation supports investigations of equilibrium behavior and provides a formal framework for testing competing evolutionary hypotheses. Twenty years of molecular evolution research have produced a rich repertoire of simulation methods. However, current models do not capture the stringent constraints acting on the domain insertions, duplications, and deletions by which multidomain architectures evolve. Although these processes have the potential to generate any combination of domains, only a tiny fraction of possible domain combinations are observed in nature. Modeling these stringent constraints on domain order and co- occurrence is a fundamental challenge in domain architecture simulation that does not arise with sequence and gene family simulation. Here we introduce a stochastic model of domain architecture evolution to simulate evolutionary trajectories that reflect the constraints on domain order and co-occurrence observed in nature. This framework is implemented in a novel domain architecture simulator, DomArchov, using the Metropolis Hastings algorithm with data-driven transition probabilities. The use of a data-driven event module enables quick and easy redeployment of the simulator for use in different taxonomic and protein function contexts. Using empirical evaluation with metazoan datasets, we demonstrate that domain architectures simulated by DomArchov recapitulate properties of genuine domain architectures that reflect the constraints on domain order and adjacency seen in nature. This work expands the realm of evolutionary processes that are amenable to simulation.
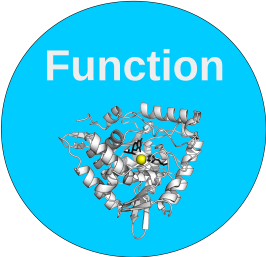
Function
DeepMHCII: A Novel Binding Core-Aware Deep Interaction Model for Accurate MHC II-peptide Binding Affinity Prediction
- Ronghui You, Fudan University, China
- Wei Qu, Fudan University, China
- Hiroshi Mamitsuka, Kyoto University / Aalto University, Japan
- Shanfeng Zhu, Fudan University, China
Presentation Overview: Show
Motivation: Computationally predicting MHC-peptide binding affinity is an important problem in immunological bioinformatics. Recent cutting-edge deep learning-based methods for this problem are unable to achieve satisfactory performance for MHC class II molecules. This is because such methods generate the input by simply concatenating the two given sequences: (the estimated binding core of) a peptide and (the pseudo sequence of) an MHC class II molecule, ignoring biological knowledge behind the interactions of the two molecules. We thus propose a binding core-aware deep learning-based model, DeepMHCII, with binding interaction convolution layer (BICL), which allows to integrate all potential binding cores (in a given peptide) with the MHC pseudo (binding) sequence, through modeling the interaction with multiple convolutional kernels.
Results: Extensive empirical experiments with four large-scale datasets demonstrate that DeepMHCII significantly outperformed four state-of-the-art methods under numerous settings, such as five-fold cross-validation, leave one molecule out, validation with independent testing sets, and binding core prediction. All these results and visualization of the predicted binding cores indicate the effectiveness of our model, DeepMHCII, and importance of properly modeling biological facts in deep learning for high predictive performance and efficient knowledge discovery.
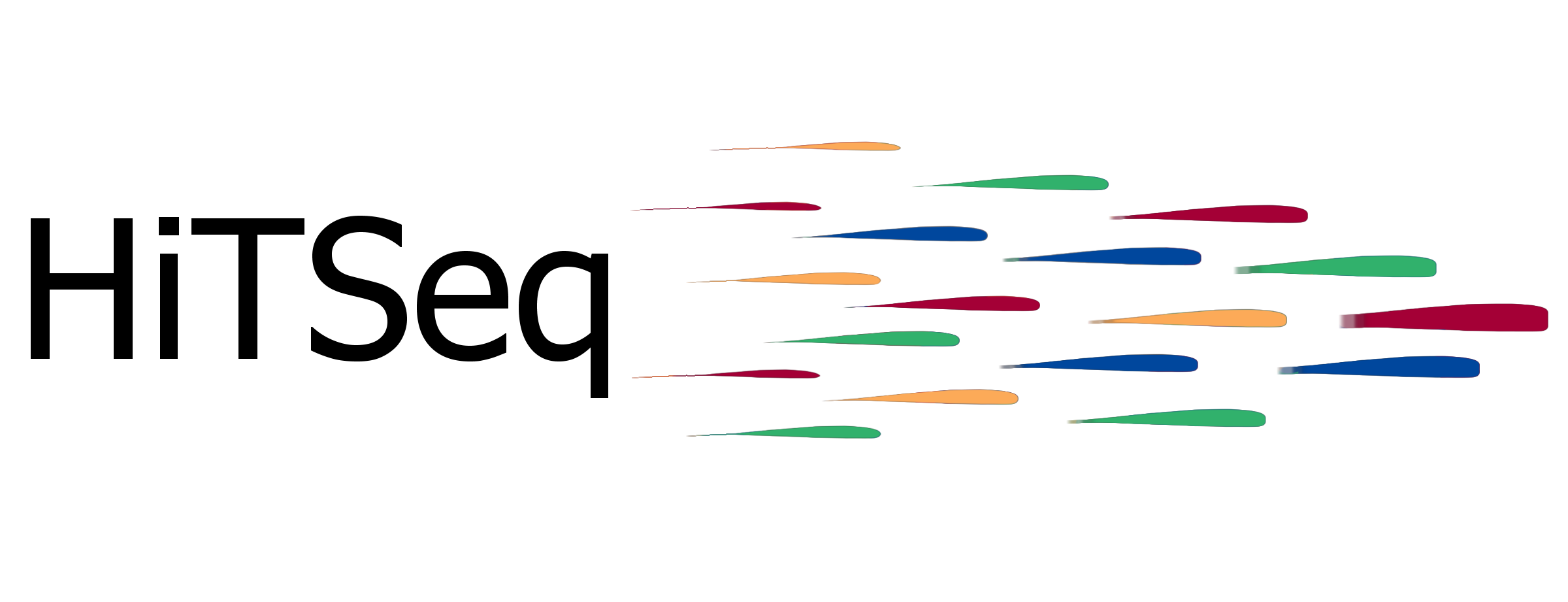
HiTSeq
CALDERA: Finding all significant de Bruijn subgraphs for bacterial GWAS
- Hector Roux de bezieux, Pendulum Therapeutics, United States
- Leandro Lima, LBBE, UCBL1, INRIA, France
- Fanny Perraudeau, Pendulum Therapeutics, San Francisco, United States
- Arnaud Mary, LBBE, France
- Sandrine Dudoit, Associate Professor, Division of Biostatistics, University of California, Berkeley, United States
- Laurent Jacob, CNRS, France
Presentation Overview: Show
Motivation: Genome wide association studies (GWAS), aiming to find genetic variants associated with a trait, have widely been used on bacteria to identify genetic determinants of drug resistance or hypervirulence. Recent bacterial GWAS methods usually rely on $k$-mers, whose presence in a genome can denote variants ranging from single nucleotide polymorphisms to mobile genetic elements. This approach does not require a reference genome, making it easier to account for accessory genes. However, a same gene can exist in slightly different versions across different strains, leading to diluted effects.
Results: Here we overcome this issue by testing covariates built from closed connected subgraphs of the De Bruijn graph defined over genomic $k$-mers. These covariates capture polymorphic genes as a single entity, improving $k$-mer based GWAS both in terms of power and interpretability. However, a method naively testing all possible subgraphs would be powerless due to multiple testing corrections, and the mere exploration of these subgraphs would quickly become computationally intractable. The concept of testable hypothesis has successfully been used to address both problems in similar contexts. We leverage this concept to test all closed connected subgraphs by proposing a novel enumeration scheme for these objects which fully exploits the pruning opportunity offered by testability, resulting in drastic improvements in computational efficiency. Our method integrates with existing visual tools to facilitate interpretation.\\
Availability: We provide an implementation of our method, as well as code to reproduce all results at https://github.com/HectorRDB/Caldera_ISMB.
Markov chains improve the significance computation of overlapping genome annotations
- Askar Gafurov, Comenius University in Bratislava, Slovakia
- Broňa Brejová, Comenius University in Bratislava, Slovakia
- Paul Medvedev, Penn State University, United States
Presentation Overview: Show
Genome annotations are a common way to represent genomic features such as genes, regulatory elements or epigenetic modifications. The amount of overlap between two annotations is often used to ascertain if there is an underlying biological connection between them. In order to distinguish between true biological association and overlap by pure chance, a robust measure of significance is required. One common way to do this is to determine if the number of intervals in the reference annotation that intersect the query annotation is statistically significant. However, currently employed statistical frameworks are often either inefficient or inaccurate when computing p-values on the scale of the whole human genome. We show that finding the p-values under the typically used ''gold'' null hypothesis is NP-hard. This motivates us to reformulate the null hypothesis using Markov chains. To be able to measure the fidelity of our Markovian null hypothesis, we develop a fast direct sampling algorithm to estimate the p-value under the gold null hypothesis. We then present an open-source software tool MCDP that computes the p-values under the Markovian null hypothesis in O(m^2+n) time and O(m) memory, where m and n are the numbers of intervals in the reference and query annotations, respectively. Notably, MCDP runtime and memory usage are independent from the genome length, allowing it to outperform previous approaches in runtime and memory usage by orders of magnitude on human genome annotations, while maintaining the same level of accuracy.
MeConcord: a new metric to quantitatively characterize DNA methylation heterogeneity across reads and CpG sites
- Xianglin Zhang, Tsinghua University, China
- Xiaowo Wang, Tsinghua University, China
Presentation Overview: Show
Motivation: Intermediately methylated regions occupy a significant fraction of the human genome and are markedly associated with epigenetic regulations or cell-type deconvolution of bulk data. However, these regions show distinct methylation patterns, corresponding to different biological mechanisms. Although there have been some metrics developed for investigating these regions, the high sensitivity to noise limits the utility for distinguishing distinct methylation patterns.
Results: We proposed a method named MeConcord to measure local methylation concordance across reads and CpG sites, respectively. MeConcord showed the most stable performance in distinguishing distinct methylation patterns (‘identical’, ‘uniform’, and ‘disordered’) compared with other metrics. Applying MeConcord to the whole genome across 25 cell lines or primary cells or tissues, we found that distinct methylation patterns were associated with different genomic characteristics, such as CTCF binding or imprinted genes. Further, we showed the differences of CpG island’s hypermethylation patterns between senescence and tumorigenesis by using MeConcord. MeConcord is a powerful method to study local read-level methylation patterns for both the whole genome and specific regions of interest.
Availability: MeConcord is available at https://github.com/vhang072/MeConcord.
ReadBouncer: Precise and Scalable Adaptive Sampling for Nanopore Sequencing
- Jens-Uwe Ulrich, Hasso Plattner Institute, Germany
- Ahmad Lutfi, Hasso Plattner Institute, Germany
- Kilian Rutzen, Robert Koch Institute, Germany
- Bernhard Renard, Hasso Plattner Institute, Germany
Presentation Overview: Show
Motivation: Nanopore sequencers allow targeted sequencing of interesting nucleotide sequences by rejecting other sequences from individual pores. This feature facilitates the enrichment of low-abundant sequences by depleting overrepresented ones in-silico. Existing tools for adaptive sampling either apply signal alignment, which cannot handle human-sized reference sequences, or apply read mapping in sequence space relying on fast GPU base callers for real-time read rejection. Using nanopore long-read mapping tools is also not optimal when mapping shorter reads as usually analyzed in adaptive sampling applications.
Results: Here we present a new approach for nanopore adaptive sampling that combines fast CPU and GPU base calling with read classification based on Interleaved Bloom Filters (IBF). ReadBouncer improves the potential enrichment of low abundance sequences by its high read classification sensitivity and specificity, outperforming existing tools in the field. It robustly removes even reads belonging to large reference sequences while running on commodity hardware without graphical processing units (GPUs), making adaptive sampling accessible for in-field researchers. Readbouncer also provides a user-friendly interface and installer files for end-users without a bioinformatics background.
Robust Fingerprinting of Genomic Databases
- Erman Ayday, Case Western Reserve University, United States
- Tianxi Ji, Case Western Reserve Univeristy, United States
- Emre Yilmaz, University of Houston-Downtown, United States
- Pan Li, Case Western Reserve University, United States
Presentation Overview: Show
Database fingerprinting has been widely used to discourage unauthorized redistribution of data by providing means to identify the source of data leakages. However, there is no fingerprinting scheme aiming at achieving liability guarantees when sharing genomic databases. Thus, we are motivated to fill in this gap by devising a vanilla fingerprinting scheme specifically for genomic databases. Moreover, since malicious genomic database recipients may compromise the embedded fingerprint by launching effective correlation attacks which leverage the intrinsic correlations among genomic data (e.g., Mendel’s law and linkage disequilibrium), we also augment the vanilla scheme by developing mitigation techniques to achieve robust fingerprinting of genomic databases against correlation attacks.
We first show that correlation attacks against fingerprinting schemes for genomic databases are very powerful. In particular, the correlation attacks can distort more than half of the fingerprint bits by causing a small utility loss (e.g.,database accuracy and consistency of SNP-phenotype associations measured via p-values). Next, we experimentally show that the correlation attacks can be effectively mitigated by our proposed mitigation techniques. We validate that the attacker can hardly compromise a large portion of the fingerprint bits even if it pays a higher cost in terms of degradation of the database utility. For example, with around 24% loss in accuracy and 20% loss in the consistency of SNP-phenotype associations, the attacker can only distort about 30% fingerprint bits, which is insufficient for it to avoid being accused. We also show that the proposed mitigation techniques also preserve the utility of the shared genomic databases.
Semi-deconvolution of bulk and single-cell RNA-seq data with application to metastatic progression in breast cancer
- Haoyun Lei, Carnegie Mellon University, United States
- Xiaoyan Guo, Carnegie Mellon University, United States
- Yifeng Tao, Carnegie Mellon University, United States
- Kai Ding, UPMC Hillman Cancer Center, Magee-Womens Research Institute, United States
- Xuecong Fu, Carnegie Mellon University, United States
- Steffi Oesterreich, UPMC Hillman Cancer Center, Magee-Womens Research Institute, United States
- Adrian Lee, UPMC Hillman Cancer Center, Magee-Womens Research Institute, United States
- Russell Schwartz, Carnegie Mellon University, United States
Presentation Overview: Show
Identifying cell types and their abundances and how these evolve during tumor progression is critical to understanding the mechanisms of metastasis and identifying predictors of metastatic potential that can guide the development of new diagnostics or therapeutics. Single-cell RNA sequencing (scRNA-seq) has been especially promising in resolving heterogeneity of expression programs at the single-cell level, but is not always feasible, for example for large cohort studies or longitudinal analysis of archived samples. In such cases, clonal subpopulations may still be inferred via genomic deconvolution, but deconvolution methods have limited ability to resolve fine clonal structure and may require reference cell type profiles that are missing or imprecise. Prior methods can eliminate the need for reference profiles but show unstable performance when few bulk samples are available. In this work, we develop a new method using reference scRNA-seq to interpret sample collections for which only bulk RNA-seq is available for some samples, e.g., clonally resolving archived primary (PRM) tissues using scRNA-seq from metastases (METs). By integrating such information in a Quadratic Programming (QP) framework, our method can recover more accurate cell types and corresponding cell type abundances in bulk samples. Application to a breast tumor bone metastases dataset confirms the power of scRNA-seq data to improve cell-type inference and quantification in same-patient bulk samples.
Sparse and Skew Hashing of K-Mers
- Giulio Ermanno Pibiri, ISTI-CNR, Italy
Presentation Overview: Show
Motivation: A dictionary of k-mers is a data structure that stores a set of n distinct k-mers and supports exact membership queries. This data structure is at the hearth of many important tasks in computational biology. High-throughput sequencing of DNA can produce very large k-mer sets, in the size of several billions of strings – in such cases, the memory consumption and query efficiency of the data structure is a concrete challenge.
Results: To tackle this problem, we describe a compressed and associative dictionary for k-mers, that is: a data structure where strings are represented in compact form and each of them is associated to a unique integer identifier in the range [0,n). We show that some statistical properties of k-mer minimizers can be exploited by minimal perfect hashing to substantially improve the space/time trade-off of the dictionary compared to the best-known solutions.
Availability: The C++ implementation of the dictionary is available at https://github.com/jermp/sshash.
The Effect of Genome Graph Expressiveness on the Discrepancy Between Genome Graph Distance and String Set Distance
- Yutong Qiu, Carnegie Mellon University, United States
- Carl Kingsford, Carnegie Mellon University, United States
Presentation Overview: Show
Motivation: Intra-sample heterogeneity describes the phenomenon where a genomic sample contains a diverse set of genomic sequences. In practice, these true string sets are often unknown due to limitations in sequencing technology. In order to compare heterogeneous samples, genome graphs are often used to represent such sets of strings. However, a genome graph is generally more expressive than string sets and is able to represent a string set universe that contains multiple sets of strings in addition to the true string set. This difference between genome graphs and string sets is not well characterized. As a result, a distance metric between genome graphs may not closely model the distance between true string sets.
Results: We extend a genome graph distance metric, Graph Traversal Edit Distance (GTED) proposed by Ebrahimpour Boroojeny et al., to FGTED to model the distance between heterogeneous string sets and show that FGTED always underestimates the Earth Mover’s Edit Distance (EMED) between string sets. We introduce the notion of string set universe diameter of a genome graph. Using the diameter, we are able to upper-bound the deviation of FGTED from EMED and improve FGTED so that it reduces the expected error in empirically estimating the similarity between true string sets. On simulated T-cell receptor sequences and Hepatitis B virus genomes, we show that the diameter-corrected FGTED reduces the deviation of the estimated distance from the true string set distances.
The minimizer Jaccard estimator is biased and inconsistent
- Mahdi Belbasi, The Pennsylvania State University, United States
- Antonio Blanca, Penn State, United States
- Robert S. Harris, The Pennsylvania State University, United States
- David Koslicki, Penn State University, United States
- Paul Medvedev, The Pennsylvania State University, United States
Presentation Overview: Show
Sketching is now widely used in bioinformatics to reduce data size and increase data processing speed. Sketching approaches entice with improved scalability but also carry the danger of decreased accuracy and added bias. In this paper, we investigate the minimizer sketch and its use to estimate the Jaccard similarity between two sequences. We show that the minimizer Jaccard estimator is biased and inconsistent, which means that the expected difference (i.e., the bias) between the estimator and the true value is not zero, even in the limit as the lengths of the sequences grow. We derive an analytical formula for the bias as a function of how the shared k-mers are laid out along the sequences. We show both theoretically and empirically that there are families of sequences where the bias can be substantial (e.g. the true Jaccard can be more than double the estimate). Finally, we demonstrate that this bias affects the accuracy of the widely used mashmap read mapping tool.
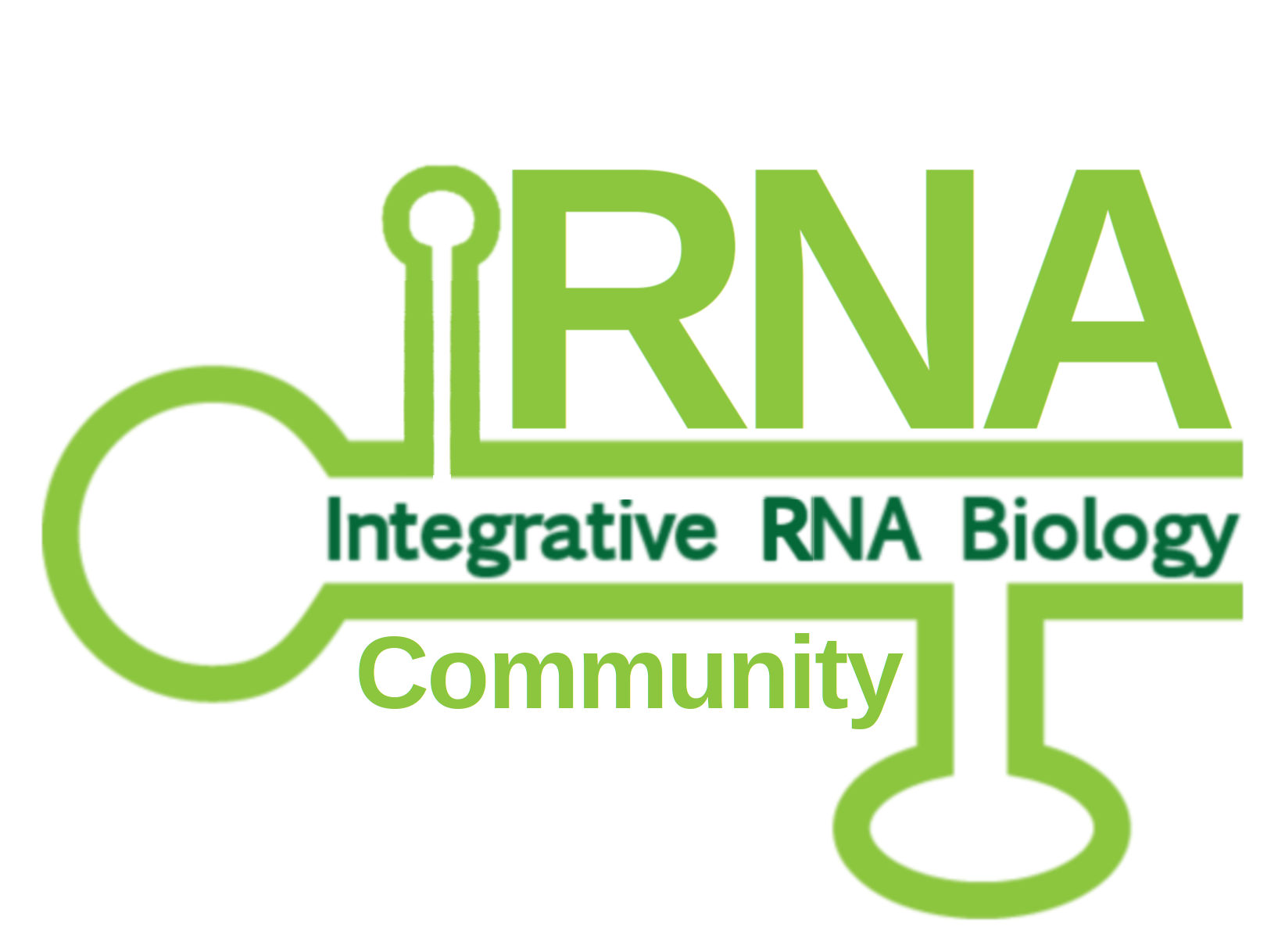
iRNA
DeepCRISTL: Deep transfer learning to predictCRISPR/Cas9 functional and endogenous on-targetediting efficiency
- Shai Elkayam, Ben-Gurion University, Israel
- Yaron Orenstein, Ben-Gurion University, Israel
Presentation Overview: Show
Motivation: In the last few years, computational methods have been used to predict the editing efficiency of CRISPR/Cas9 gene editing for any guide RNA (gRNA) of interest. High-throughput datasets were collected to train machine-learning models for this task, but they have a low correlation with functional or endogenous editing.
Results: To better utilize high-throughput datasets of CRISPR/Cas9 editing for functional and endogenous editing predictions, we developed DeepCRISTL, a deep learning model to predict the on-target efficiency given a gRNA sequence. The DeepCRISTL model is based on pre-training on more than 150,000 gRNAs over three enzymes, and improving prediction performance by multi-task and ensemble techniques. Our new model achieves state-of-the-art results over all three enzymes: up to 0.89 in Spearman correlation between predicted and measured on-target efficiencies. To fine-tune the model for functional or endogenous prediction tasks, we tested several transfer-learning (TL) approaches, with gradual-learning being the overall best performer. Our final model DeepCRISTL has been evaluated and compared versus popular extant methods, and achieved state-of-the-art results over all datasets.
Availability: DeepCRISTL is publicly available at github.com/OrensteinLab/DeepCRISTL/.
PhyloPGM: Boosting Regulatory Function Prediction Accuracy Using Evolutionary Information
- Faizy Ahsan, McGill University, Canada
- Zichao Yan, McGill University, Canada
- Doina Precup, McGill University, Canada
- Mathieu Blanchette, McGill University, Canada
Presentation Overview: Show
Motivation: The computational prediction of regulatory function associated with a genomic sequence is of utter importance in -omics study, which facilitates our understanding of the underlying mechanisms underpinning the vast gene regulatory network. Prominent examples in this area include the binding prediction of transcription factors in DNA regulatory regions, and predicting RNA-protein interaction in the context of post-transcriptional gene expression. However, existing computational methods have suffered from high false positive rates and have seldom used any evolutionary information, despite the vast amount of available orthologous data across multitudes of extant and ancestral genomes, which readily present an opportunity to improve the accuracy of existing computational methods.
Results: In this study, we present a novel probabilistic approach called PhyloPGM that leverages previously trained TFBS or RNA-RBP binding predictors by aggregating their predictions from various orthologous regions, in order to boost the overall prediction accuracy on human sequences. Throughout our experiments, PhyloPGM has shown significant improvement over baselines such as the sequence- based RNA-RBP binding predictor RNATracker and the sequence-based TFBS predictor that is known as FactorNet. PhyloPGM is simple in principle, easy to implement and yet, yields impressive results.
Visualizing hierarchies in scRNA-seq data using a density tree-biased autoencoder
- Manfred Claassen, University of Tübingen, Germany
- Quentin Garrido, Université Gustave Eiffel, ESIEE Paris, LIGM, France
- Sebastian Damrich, Heidelberg University, Germany
- Alexander Jäger, Heidelberg University, Germany
- Dario Cerletti, ETH Zurich, Switzerland
- Laurent Najman, Université Gustave Eiffel, ESIEE Paris, LIGM, France
- Fred Hamprecht, Heidelberg University, Germany
Presentation Overview: Show
Motivation: Single cell RNA sequencing (scRNA-seq) data makes studying the development of cells possible at unparalleled resolution. Given that many cellular differentiation processes are hierarchical, their scRNA-seq data is expected to be approximately tree-shaped in gene expression space. Inference and representation of this tree-structure in two dimensions is highly desirable for biological interpretation and exploratory analysis.
Results: Our two contributions are an approach for identifying a meaningful tree structure from high-dimensional scRNA-seq data, and a visualization method respecting the tree-structure. We extract the tree structure by means of a density based minimum spanning tree on a vector quantization of the data and show that it captures biological information well. We then introduce DTAE, a tree-biased autoencoder that emphasizes the tree structure of the data in low dimensional space. We compare to other dimension reduction methods and demonstrate the success of our method both qualitatively and quantitatively on real and toy data.
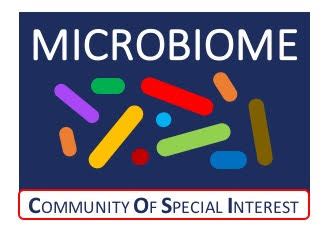
MICROBIOME
CMash: fast, multi-resolution estimation of k-mer-based Jaccard and containment indices
- Shaopeng Liu, Pennsylvania State University, United States
- David Koslicki, Pennsylvania State University, United States
Presentation Overview: Show
K-mer based methods are used ubiquitously in the field of computational biology. However, determining the optimal value of k for a specific application often remains heuristic. Simply reconstructing a new k-mer set with another k-mer size is computationally expensive, especially in metagenomic analysis where data sets are large. Here, we introduce a hashing-based technique that leverages a kind of bottom-m sketch as well as a k-mer ternary search tree (KTST) to obtain k-mer based similarity estimates for a range of k values. By truncating k-mers stored in a pre-built KTST with a large k = k_max value, we can simultaneously obtain k-mer based estimates for all k values up to k_max. This truncation approach circumvents the reconstruction of new k-mer sets when changing k values, making analysis more time and space-efficient. For example, we show that when using a KTST to estimate the containment index between a RefSeq-based microbial reference database and simulated metagenome data for 10 values of k, the running time is close to 10x faster compared to a classic MinHash approach while using less than one-fifth the space to store the data structure. A python implementation of this method, CMash, is available at https://github.com/dkoslicki/CMash. The reproduction of all experiments presented herein can be accessed via https://github.com/KoslickiLab/CMASH-reproducibles.
Syotti: Scalable Bait Design for DNA Enrichment
- Jarno Alanko, University of Helsinki, Finland
- Ilya Slizovskiy, Department of Veterinary Population Medicine, College of Veterinary Medicine, University of Minnesota, United States
- Daniel Lokshtanov, University of California, Santa Barbara, United States
- Travis Gagie, Diego Portales University, Finland
- Noelle Noyes, University of Minnesota, United States
- Christina Boucher, University of Florida, United States
Presentation Overview: Show
Motivation: Bait enrichment is a relatively new protocol that is becoming increasingly ubiquitous as it has
been shown to successfully amplify regions of interest in metagenomic samples. In this method, a set of
synthetic probes (“baits”) are designed, manufactured, and applied to fragmented metagenomic DNA. The
probes bind to the fragmented DNA and any unbound DNA is rinsed away, leaving the bound fragments to
be amplified for sequencing. Most recently, Metsky et al. (Nature Biotech 2019) demonstrated that bait-enrichment
is capable of detecting a large number of human viral pathogens within metagenomic samples.
Results: We formalize the problem of designing baits by defining the Minimum Bait Cover problem,
and show that the problem is NP-hard even under very restrictive assumptions, and design an efficient heuristic that takes advantage of
succinct data structures. We refer to our method as Syotti. The running time of Syotti shows linear scaling
in practice, running at least an order of magnitude faster than state-of-the-art methods, including the recent
method of Metsky et al. At the same time, our method produces bait sets that are smaller than the ones
produced by the competing methods, while also leaving fewer positions uncovered. Lastly, we show that
Syotti requires only 25 minutes to design baits for a dataset comprised of 3 billion nucleotides from 1000
related bacterial substrains, whereas the method of Metsky et al. shows clearly super-linear running time
and fails to process even a subset of 8% of the data in 24 hours.
Availability: https://github.com/jnalanko/syotti.
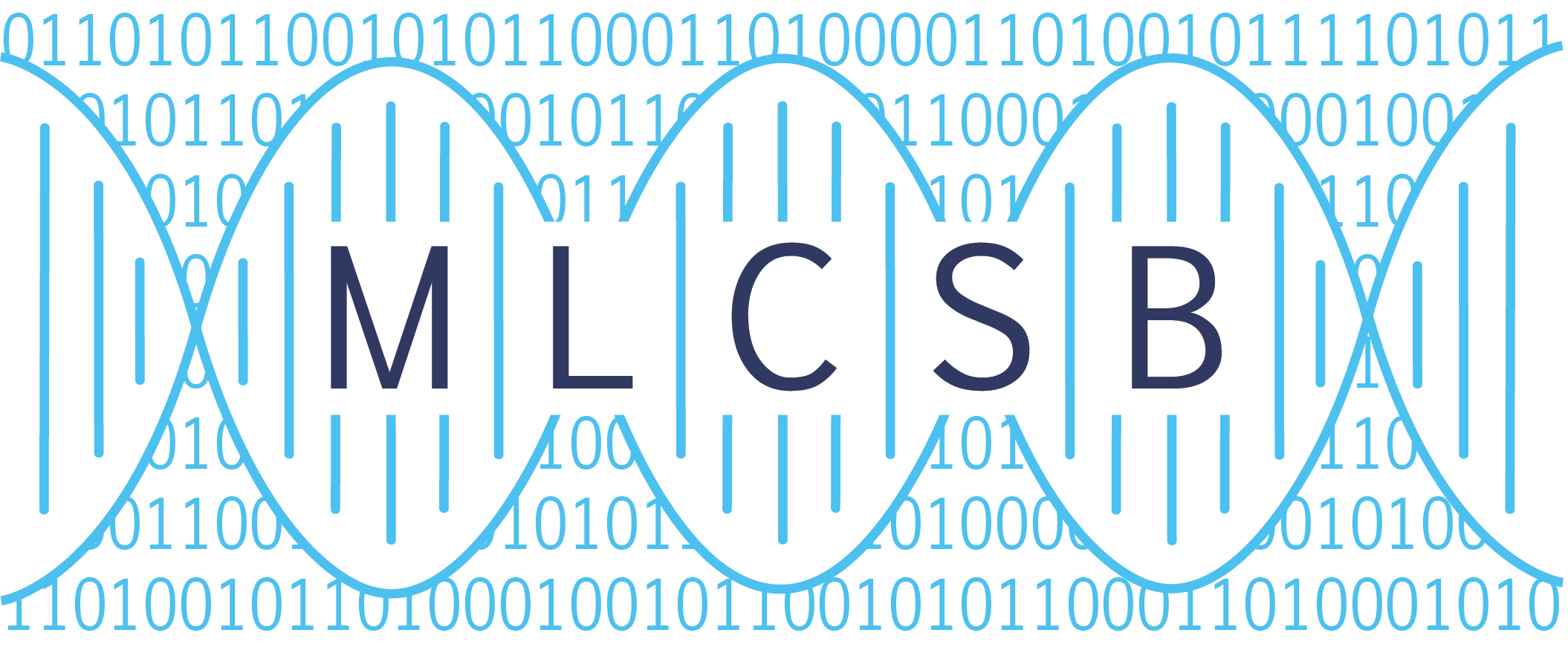
MLCSB
A Graph Neural Network Approach for Molecule Carcinogenicity Prediction
- Philip Fradkin, Univeristy of Toronto, Vector Institute, Canada
- Adamo Young, Univeristy of Toronto, Vector Institute, Canada
- Lazar Atanackovic, Univeristy of Toronto, Vector Institute, Canada
- Brendan Frey, Univeristy of Toronto, Vector Institute, Canada
- Leo Lee, Univeristy of Toronto, Vector Institute, Canada
- Bo Wang, Univeristy of Toronto, Vector Institute, Canada
Presentation Overview: Show
Molecular carcinogenicity is a preventable cause of cancer, but systematically identifying carcinogenic compounds, which involves performing experiments on animal models, is expensive, time consuming, and low throughput. As a result, carcinogenicity information is fairly limited and building data-driven models with good prediction accuracy remains a major challenge. In this work, we propose CONCERTO, a deep learning model that uses a graph transformer in conjunction with a molecular fingerprint representation for carcinogenicity prediction from molecular structure. Special efforts have been made to overcome the data size constraint, such as enriching the training data with more informative labels, multi-round pre-training on related but lower quality mutagenicity data, and transfer learning from a large self-supervised model. Extensive experiments demonstrate that our model performs well and can generalize to external validation sets. CONCERTO could be useful for guiding future carcinogenicity experiments and provide insight into the molecular basis of carcinogenicity.
BITES: Balanced Individual Treatment Effect for Survival data
- Stefan Schrod, University Medical Center Göttingen, Germany
- Andreas Schäfer, Universität Regensburg, Germany
- Stefan Solbrig, Universität Regensburg, Germany
- Robert Lohmayer, Leibniz Institute for Immunotherapy Regensburg, Germany
- Wolfram Gronwald, Universität Regensburg, Germany
- Peter J. Oefner, Universität Regensburg, Germany
- Tim Beißbarth, University Medical Center Göttingen, Germany
- Rainer Spang, Universität Regensburg, Germany
- Helena Zacharias, Universität Kiel, Germany
- Michael Altenbuchinger, University Medical Center Göttingen, Germany
- Stefan Schrod, University Medical Center Göttingen, Germany
- Andreas Schäfer, Universität Regensburg, Germany
- Stefan Solbrig, Universität Regensburg, Germany
- Robert Lohmayer, Leibniz Institute for Immunotherapy Regensburg, Germany
- Wolfram Gronwald, Universität Regensburg, Germany
- Peter J. Oefner, Universität Regensburg, Germany
- Tim Beißbarth, University Medical Center Göttingen, Germany
- Rainer Spang, Universität Regensburg, Germany
- Helena Zacharias, Universität Kiel, Germany
- Michael Altenbuchinger, University Medical Center Göttingen, Germany
Presentation Overview: Show
Estimating the effects of interventions on patient outcome is one of the key aspects of personalized medicine. Their inference is often challenged by the fact that the training data comprises only the outcome for the administered treatment, and not for alternative treatments (the so-called counterfactual outcomes). Several methods were suggested for this scenario based on observational data, i.e.~data where the intervention was not applied randomly, for both continuous and binary outcome variables. However, patient outcome is often recorded in terms of time-to-event data, comprising right-censored event times if an event does not occur within the observation period. Albeit their enormous importance, time-to-event data is rarely used for treatment optimization.
We suggest an approach named BITES (Balanced Individual Treatment Effect for Survival data), which combines a treatment-specific semi-parametric Cox loss with a treatment-balanced deep neural network; i.e.~we regularize differences between treated and non-treated patients using Integral Probability Metrics (IPM). We show in simulation studies that this approach outperforms the state of the art. Further, we demonstrate in an application to a cohort of breast cancer patients that hormone treatment can be optimized based on six routine parameters. We successfully validated this finding in an independent cohort. We provide BITES as an easy-to-use python implementation including scheduled hyper-parameter optimization.
DECODE: a computational pipeline to discover T-cell receptor binding rules
- An-Phi Nguyen, IBM Research Europe, ETH Zurich, Switzerland
- Maria Rodriguez Martinez, IBM Research Europe, Switzerland
- Iliana Papadopoulou, IBM Research Europe, ETH Zurich, Switzerland
- Anna Weber, IBM Research Europe, ETH Zurich, Switzerland
Presentation Overview: Show
Motivation: Understanding the mechanisms underlying T cell receptor (TCR) binding is of fundamental importance to understanding adaptive immune responses. A better understanding of the biochemical rules governing TCR binding can be used, for example, to guide the design of more powerful and safer T cell-based therapies. Advances in repertoire sequencing technologies have made available millions of TCR sequences. Data abundance has, in turn, fueled the development of many computational models to predict the binding properties of TCRs from their sequences. Unfortunately, while many of these works have made great strides towards predicting TCR specificity using machine learning, the black-box nature of these models has resulted in a limited understanding of the rules that govern the binding of a TCR and an epitope. Results: We present an easy-to-use and customizable pipeline, DECODE, to extract the binding rules from any black-box model designed to predict the TCR-epitope binding. DECODE offers a range of analytical and visualization tools to guide the user in the extraction of such rules. We demonstrate our pipeline on a recently published TCR binding prediction model, TITAN, and show how to use the provided metrics to assess the quality of the computed rules.
In conclusion, DECODE can lead to a better understanding of the sequence motifs that underlie TCR binding. Our pipeline can facilitate the investigation of current immunotherapeutic challenges, such as cross-reactive events due to off-target TCR binding.
Fast and interpretable genomic data analysis using multiple approximate kernel learning
- Ayyüce Begüm Bektaş, Koç University, Turkey
- Çiğdem Ak, Oregon Health & Science University, United States
- Mehmet Gönen, Koç University, Turkey
Presentation Overview: Show
Motivation: Dataset sizes in computational biology have been increased drastically with the help of improved data collection tools and increasing size of patient cohorts. Previous kernel-based machine learning algorithms proposed for increased interpretability started to fail with large sample sizes, owing to their lack of scalability. To overcome this problem, we proposed a fast and efficient multiple kernel learning (MKL) algorithm to be particularly used with large-scale data that integrates kernel approximation and group Lasso formulations into a conjoint model. Our method extracts significant and meaningful information from the genomic data while conjointly learning a model for out-of-sample prediction. It is scalable with increasing sample size by approximating instead of calculating distinct kernel matrices.
Results: To test our computational framework, namely, Multiple Approximate Kernel Learning (MAKL), we demonstrated our experiments on three cancer datasets and showed that MAKL is capable to outperform the baseline algorithm while using only a small fraction of the input features. We also reported selection frequencies of approximated kernel matrices associated with feature subsets (i.e. gene sets/pathways), which helps to see their relevance for the given classification task. Our fast and interpretable MKL algorithm producing sparse solutions is promising for computational biology applications considering its scalability and highly correlated structure of genomic datasets, and it can be used to discover new biomarkers and new therapeutic guidelines.
psupertime: supervised pseudotime analysis for time-series single cell RNA-seq data
- Will Macnair, ETH, Switzerland
- Revant Gupta, University of Tübingen, Germany
- Manfred Claassen, University of Tübingen, Germany
Presentation Overview: Show
Improvements in single cell RNA-seq technologies mean that studies measuring multiple experimental conditions, such as time series, have become more common. At present, few computational methods exist to infer time series-specific transcriptome changes, and such studies have therefore typically used unsupervised pseudotime methods. While these methods identify cell subpopulations and the transitions between them, they are not appropriate for identifying the genes which vary coherently along the time series. In addition, the orderings they estimate are based only on the major sources of variation in the data, which may not correspond to the processes related to the time labels.
We introduce psupertime, a supervised pseudotime approach based on a regression model, which explicitly uses time series labels as input. It identifies genes that vary coherently along a time series, in addition to pseudotime values for individual cells, and a classifier which can be used to estimate labels for new data with unknown or differing labels. We show that psupertime outperforms benchmark classifiers in terms of identifying time-varying genes, and provides better individual cell orderings than popular unsupervised pseudotime techniques. psupertime is applicable to any single cell RNA-seq dataset with sequential labels (principally time series but also drug dosage and disease progression, for example), derived from either experimental design and provides a fast, interpretable tool for targeted identification of genes varying along with specific biological processes.
Scaling Multi-Instance Support Vector Machine to Breast Cancer Detection on the BreaKHis Dataset
- Hoon Seo, Colorado School of Mines, United States
- Lodewijk Brand, Colorado School of Mines, United States
- Lucia Saldana Barco, Colorado School of Mines, United States
- Hua Wang, Colorado School of Mines, United States
Presentation Overview: Show
Breast cancer is a type of cancer that develops in breast tissue, and, after skin cancer, it is the most commonly diagnosed cancer in women in the United States. Given that an early diagnosis is imperative to prevent breast cancer progression, many machine learning models have automated the histopathological classification of the different types of carcinomas. However, many of them are not scalable to the large dataset. In this study, we propose the novel Primal-Dual Multi-Instance Support Vector Machine (pdMISVM) to determine which tissue segments in an image exhibit an indication of an abnormality. We also derive the efficient optimization approach for the proposed method by bypassing the quadratic programming and least-squares problems, which are commonly employed to optimize Support Vector Machine (SVM) models in multi-instance learning. The proposed method is scalable to large datasets, and it is computationally efficient. We applied our method to the public BreaKHis dataset and achieved promising prediction performance and scalability for histopathological classification.
SPARSE: a sparse hypergraph neural network for learning multiple types of latent combinations to accurately predict drug-drug interactions
- Duc Anh Nguyen, Kyoto University, Japan
- Canh Hao Nguyen, Kyoto University, Japan
- Peter Petschner, Kyoto University, Japan
- Hiroshi Mamitsuka, Kyoto University, Japan
Presentation Overview: Show
Motivation: Predicting side effects of drug-drug interactions (DDIs) is an important task in pharmacology. The state-of-the-art methods for DDI prediction use hypergraph neural networks to learn latent representations of drugs and side effects to express high-order relationships among two interacting drugs and a side effect. The idea of these methods is that each side effect is caused by a unique combination of latent features of the corresponding interacting drugs. However, in reality, a side effect might have multiple, different mechanisms that cannot be represented by a single combination of latent features of drugs. Moreover, DDI data is sparse, suggesting that using a sparsity regularization would help to learn better latent representations to improve prediction performances.
Result: We propose SPARSE, which encodes the DDI hypergraph and drug features to latent spaces to learn multiple types of combinations of latent features of drugs and side effects, controlling the model sparsity by a sparse prior. Our extensive experiments using both synthetic and three real-world DDI datasets showed the clear predictive performance advantage of SPARSE over cutting-edge competing methods. Also latent feature analysis over unknown top predictions by SPARSE demonstrated the interpretability advantage contributed by the model sparsity.
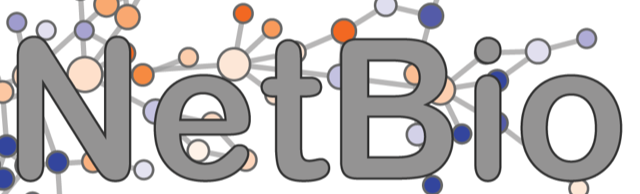
NetBio
Computing optimal factories in metabolic networks with negative regulation
- Spencer Krieger, University of Arizona, United States
- John Kececioglu, University of Arizona, United States
Presentation Overview: Show
Motivation: A factory in a metabolic network specifies how to produce target molecules from source compounds through biochemical reactions, properly accounting for reaction stoichiometry to conserve or not deplete intermediate metabolites. While finding factories is a fundamental problem in systems biology, available methods do not consider the number of reactions used, nor address negative regulation.
Methods: We introduce the new problem of finding optimal factories that use the fewest reactions, for the first time incorporating both first- and second-order negative regulation. We model this problem with directed hypergraphs, prove it is NP-complete, solve it via mixed-integer linear programming, and accommodate second-order negative regulation by an iterative approach that generates next-best factories.
Results: This optimization-based approach is remarkably fast in practice, typically finding optimal factories in a few seconds, even for metabolic networks involving tens of thousands of reactions and metabolites, as demonstrated through comprehensive experiments across all instances from standard reaction databases.
Availability and implementation: Source code for an implementation of our new method for optimal factories with negative regulation in a new tool called Odinn, together with all datasets, is available free for non-commercial use at http://odinn.cs.arizona.edu.
DEMA: a distance-bounded energy-field mini-mization algorithm to model and layout bio-molecular networks with quantitative features
- Zhenyu Weng, Institute of Big Data Technologies, Shenzhen Graduate School, Peking University, China, China
- Zongliang Yue, Informatics Institute, School of Medicine, University of Alabama at Birmingham, USA, United States
- Yuesheng Zhu, Institute of Big Data Technologies, Shenzhen Graduate School, Peking University, China, China
- Jake Chen, Informatics Institute, School of Medicine, University of Alabama at Birmingham, United States
Presentation Overview: Show
In biology, graph layout algorithms can reveal comprehensive biological contexts by visually position-ing graph nodes in their relevant neighborhoods. A layout software algorithm/engine commonly takes a set of nodes and edges and produces layout coordinates of nodes according to edge constraints. However, current layout engines normally do not consider node, edge, or node-set properties during layout and only curate these properties after layout is created. Here, we propose a new layout algo-rithm, distance-bounded energy-field minimization algorithm (DEMA), to natively consider various biological factors, i.e., the strength of gene-to-gene association, the gene’s relative contribution weight, and the functional groups of genes, to enhance the interpretation of complex network graphs. In DEMA, we introduce a parameterized energy model where nodes are repelled by the network to-pology and attracted by a few biological factors, i.e., interaction coefficient (IC), effect coefficient (EC), and fold change (FC) of gene expression. We generalize these factors as gene weights, PPI weights, gene-to-gene correlations, and the gene set annotations—four parameterized functional properties used in DEMA. Moreover, DEMA considers further attraction/repulsion/grouping coefficient to enable different preferences in generating network views. Applying DEMA, we performed two case studies using genetics data in Autism Spectrum Disorder (ASD) and Alzheimer’s disease (AD), re-spectively, for gene candidate discovery. Furthermore, we implement our algorithm as a plugin to Cytoscape, an open-source software platform for visualizing networks; hence, it is convenient. Our software and demo can be freely accessed at http://discovery.informatics.uab.edu/dema.
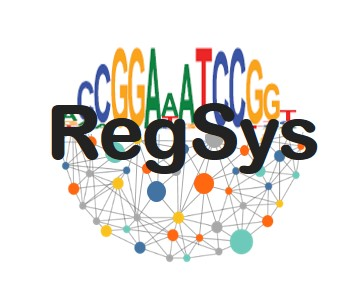
RegSys
Do-calculus enables estimation of causal effects in partially observed biomolecular pathways
- Sara Mohammad Taheri, Northeastern Univerusity, United States
- Jeremy Zucker, Pacific Northwest National Laboratory, United States
- Charles Tapley Hoyt, Laboratory of Systems Pharmacology, Harvard Medical School, United States
- Karen Sachs, Next Generation Analytics, United States
- Vartika Tewari, Northeastern Univerusity, United States
- Robert Ness, Microsoft Research, United States
- Olga Vitek, Northeastern Univerusity, United States
Presentation Overview: Show
Estimating causal queries, such as changes in protein abundance in response to a perturbation, is a fundamental task in the analysis of biomolecular pathways.
The estimation requires experimental measurements on the pathway components. However, in practice, many pathway components are left unobserved (latent) because they are either unknown or difficult to measure. Latent variable models (LVMs) are well-suited for such estimation. Unfortunately, LVM-based estimation of causal queries can be inaccurate when parameters of the latent variables are not uniquely identified, or when the number of latent variables is misspecified. This has limited the use of LVMs for causal inference in biomolecular pathways. In this manuscript, we propose a general and practical approach for LVM-based estimation of causal queries.
We prove that, despite the challenges above, LVM-based estimators of causal queries are accurate if the queries are identifiable according to Pearl's do-calculus, and describe an algorithm for its estimation. We illustrate the breadth and the practical utility of this approach for estimating causal queries in four synthetic and two experimental case studies, where structures of biomolecular pathways challenge the existing methods for causal query estimation.
MOJITOO: a fast and universal method for integration of multimodal single cell data
- Mingbo Cheng, RWTH Aachen, Germany
- Zhijian Li, RWTH Aachen, Germany
- Ivan G. Costa, RWTH Aachen, Germany
Presentation Overview: Show
Motivation: The advent of multi-modal single cell sequencing techniques have shed new light on molecular mechanisms by simultaneously inspecting transcriptomes, epigenomes and proteomes of the same cell. However, to date, the existing computational approaches for integration of multimodal single cell data are either computationally expensive, require the delineation of parameters or can only be applied to particular modalities.
Results: Here we present a single cell multi-modal integration method, named MOJITOO (Multi-mOdal Joint IntegraTion of cOmpOnents). MOJITOO uses canonical correlation analysis for a fast and parameter free detection of a shared representation of cells from multimodal single cell data. Moreover, estimated canonical components can be used for interpretation, i.e. association of modality specific molecular features with the latent space. We evaluate MOJITOO using bi- and tri-modal single cell data sets and show that MOJITOO outperforms existing methods regarding computational requirements, preservation of original latent spaces and clustering.
Availability: The software is available at https://github.com/CostaLab/MOJITOO
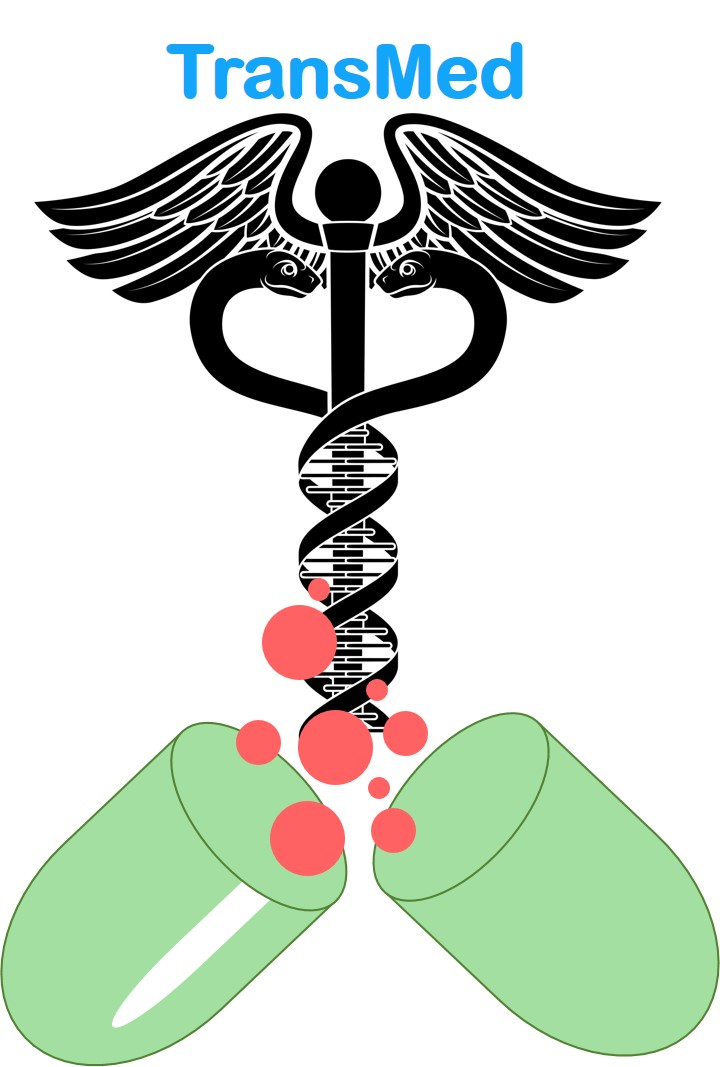
TransMed
From drug repositioning to target repositioning: prediction of therapeutic targets using genetically perturbed transcriptomic signatures
- Satoko Namba, Kyushu Institute of Technology, Japan
- Michio Iwata, Kyushu Institute of Technology, Japan
- Yoshihiro Yamanishi, Kyushu Institute of Technology, Japan
Presentation Overview: Show
Motivation: A critical element of drug development is the identification of therapeutic targets for diseases. However, the depletion of therapeutic targets is a serious problem.
Results: In this study, we propose the novel concept of target repositioning, an extension of the concept of drug repositioning, to predict new therapeutic targets of various diseases. Predictions were performed by a trans-disease analysis which integrated genetically perturbed transcriptomic signatures (knock-down of 4,345 genes and over-expression of 3,114 genes) and disease-specific gene transcriptomic signatures of 79 diseases. The trans-disease method, which takes into account similarities among diseases, enabled us to distinguish the inhibitory from activatory targets, and to predict the therapeutic targetability of not only proteins with known target–disease associations, but also orphan proteins without known associations. Our proposed method is expected to be useful for understanding the commonality of mechanisms among diseases and for therapeutic target identification in drug discovery.
Availability: Supplemental information and software are available at the following website [http://labo.bio.kyutech.ac.jp/~yamani/target_repositioning/].
Contact: yamani@bio.kyutech.ac.jp
Supplementary information: Supplementary data are available at Bioinformatics online.
MLGL-MP: A Multi-Label Graph Learning Framework Enhanced by Pathway Interdependence for Metabolic Pathway Prediction
- Bing-Xue Du, School of Life Sciences, Northwestern Polytechnical University, China
- Peng-Cheng Zhao, School of Life Sciences, Northwestern Polytechnical University, China
- Bei Zhu, School of Life Sciences, Northwestern Polytechnical University, China
- Siu-Ming Yiu, Department of Computer Science, The University of Hong Kong, Hong Kong, China
- Arnold K Nyamabo, School of Computer Science, Northwestern Polytechnical University, China
- Hui Yu, School of Computer Science, Northwestern Polytechnical University, China
- Jian-Yu Shi, School of Life Sciences, Northwestern Polytechnical University, China
Presentation Overview: Show
Motivation: During lead compound optimization, it is crucial to identify pathways where a drug-like compound is metabolized. Recently, machine learning-based methods have achieved inspiring progress to predict potential metabolic pathways for drug-like compounds. However, they neglect the knowledge that metabolic pathways are dependent on each other. Moreover, they are inadequate to elucidate why compounds participate in specific pathways.
Results: To address these issues, we propose a novel multi-label graph learning framework of metabolic pathway prediction boosted by pathway inter-dependence, called MLGL-MP, which contains a compound encoder, a pathway encoder, and a multi-label predictor. The compound encoder learns compound embedding representations by graph neural networks (GNNs). After constructing a pathway dependence graph by re-trained word embeddings and pathway co-occurrences, the pathway encoder learns pathway embeddings by graph convolutional networks (GCNs). Moreover, after adapting the compound embedding space into the pathway embedding space, the multi-label predictor measures the proximity of two spaces to discriminate which pathways a compound participates in. The comparison with state-of-the-art methods on KEGG pathways demonstrates the superiority of our MLGL-MP. Also, the ablation studies reveal how its three components contribute to the model, including the pathway dependence, the adapter between compound embeddings and pathway embeddings, as well as the pre-training strategy. Furthermore, a case study illustrates the interpretability of MLGL-MP by indicating crucial substructures in a compound, which are significantly associated with the attending metabolic pathways. It’s anticipated that this work can boost metabolic pathway predictions in drug discovery.
Prediction of Recovery from Multiple Organ Dysfunction Syndrome in Pediatric Sepsis Patients
- Swiss Pediatric Sepsis Study, Switzerland
- Karsten Borgwardt, ETH Zurich, Switzerland
- Bowen Fan, ETH Zurich, Switzerland
- Juliane Klatt, ETH Zurich, Switzerland
- Michael Moor, ETH Zurich, Switzerland
- Latasha Daniels, Ann & Robert H. Lurie Children's Hospital of Chicago, United States
- Lazaro Sanchez-Pinto, Ann & Robert H. Lurie Children's Hospital of Chicago, United States
- Philipp Agyeman, University Hospital of Bern, Switzerland
- Luregn Schlapbach, University Children’s Hospital Zurich, Switzerland
Presentation Overview: Show
Sepsis is a leading cause of death and disability in children globally, accounting for approximately three million childhood deaths per year. In pediatric sepsis patients, the multiple organ dysfunction syndrome (MODS) is considered a significant risk factor for adverse clinical outcomes characterized by high mortality and morbidity in the pediatric intensive care unit (PICU). The recent rapidly growing availability of electronic health records (EHRs) has allowed researchers to vastly develop data-driven approaches like machine learning in healthcare and achieved great successes. However, effective machine learning models which could make the accurate early prediction of the recovery in pediatric sepsis patients from MODS to a mild state and thus assist the clinicians in the decision-making process is still lacking.
This study develops a machine learning-based approach to predict the recovery from MODS to zero or single organ dysfunction~(Z/SOD) by one week in advance in the Swiss Pediatric Sepsis Study (SPSS) cohort of children with blood-culture confirmed bacteremia. Our model achieves internal validation performance on the SPSS cohort with an AUROC of 79.1 and AUPRC of 73.6, and it was also externally validated on another pediatric sepsis patients cohort collected in the U.S., yielding an AUROC of 76.4 and AUPRC of 72.4. These results indicate that our model has the potential to be included into the EHRs system and contribute to patient assessment and triage in pediatric sepsis patient care.
Self-supervised learning of cell type specificity from immunohistochemical images
- Michael Murphy, Massachusetts Institute of Technology, United States
- Stefanie Jegelka, Massachusetts Institute of Technology, United States
- Ernest Fraenkel, Massachusetts Institute of Technology, United States
Presentation Overview: Show
Motivation: Advances in bioimaging now permit in-situ proteomic characterization of cell-cell interactions in complex tissues, with important applications across a spectrum of biological problems from development to disease. These methods depend on selection of antibodies targeting proteins that are expressed specifically in particular cell types. Candidate marker proteins are often identified from single-cell transcriptomic data, with variable rates of success, in part due to divergence between expression levels of proteins and the genes that encode them. In principle, marker identification could be improved by using existing databases of immunohistochemistry for thousands of antibodies in human tissue, such as the Human Protein Atlas. However, these data lack detailed annotations of the types of cells in each image.
Results: We develop a method to predict cell type specificity of protein markers from unlabeled images. We train a convolutional neural network with a self-supervised objective to generate embeddings of the images. Using nonlinear dimensionality reduction, we observe that the model clusters images according to cell types and anatomical regions for which the stained proteins are specific. We then use estimates of cell type specificity derived from an independent single-cell transcriptomics dataset to train an image classifier, without requiring any human labelling of images. Our scheme demonstrates superior classification of known proteomic markers in kidney compared to differential expression in single-cell transcriptomics.
Synthetic-to-Real: Instance Segmentation of Clinical Cluster Cells with Unlabelled Synthetic Training
- Meng Zhao, Tianjin University of Technology, China
- Siyu Wang, Tianjin University of Technology, China
- Fan Shi, Tianjin University of Technology, China
- Chen Jia, Tianjin University of Technology, China
- Xuguo Sun, Tianjin Medical University, China
- Shengyong Chen, Tianjin University of Technology, China
Presentation Overview: Show
The presence of tumor cell clusters in pleural effusion may be a signal of cancer metastasis. The instance segmentation of single cell from cell clusters plays a pivotal role for cluster cell analysis. However, current cell segmentation methods perform poorly for cluster cells due to the overlapping/ touching characters of clusters, multiple instance properties of cells, and the poor generalization ability of the models. In the paper, we propose a contour constraint instance segmentation framework (CC framework) for cluster cells based on a cluster cell combination enhancement module. The framework can accurately locate each instance from cluster cells and realize highprecision contour segmentation under a few samples. Specifically, we propose the contour attention constraint (CAC) module to alleviate over-segmentation and under-segmentation among individual cell-instance boundaries. In addition, to evaluate the framework, we construct a pleural effusion cluster cell dataset including 197 high-quality samples. The quantitative results show that the numeric result of AP mask is greater than 90%, a more than 10% increase compared with state-of-the-art semantic segmentation algorithms. From the qualitative results, we can observe that our method rarely has segmentation errors.
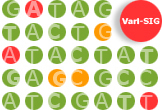
VarI
PolarMorphism enables discovery of shared genetic variants across multiple traits from GWAS summary statistics
- Joanna von Berg, University Medical Center Utrecht, Netherlands
- Michelle ten Dam, University Medical Center Utrecht, Netherlands
- Sander van der Laan, University Medical Center Utrecht, Netherlands
- Jeroen de Ridder, University Medical Center Utrecht, Netherlands
Presentation Overview: Show
Pleiotropic SNPs are associated with multiple traits. Such SNPs can help pinpoint biological processes with an effect on multiple traits or point to a shared etiology between traits. We present PolarMorphism, a new method for the identification of pleiotropic SNPs from GWAS summary statistics. PolarMorphism can be readily applied to more than two traits or whole trait domains. PolarMorphism makes use of the fact that trait-specific SNP effect sizes can be seen as Cartesian coordinates and can thus be converted to polar coordinates r (distance from the origin) and theta (angle with the Cartesian x-axis). r describes the overall effect of a SNP, while theta describes the extent to which a SNP is shared. r and theta are used to determine the significance of SNP sharedness, resulting in a p-value per SNP that can be used for further analysis. We apply PolarMorphism to a large collection of publicly available GWAS summary statistics enabling the construction of a pleiotropy network that shows the extent to which traits share SNPs. This network shows how PolarMorphism can be used to gain insight into relationships between traits and trait domains. Furthermore, pathway analysis of the newly discovered pleiotropic SNPs demonstrates that analysis of more than two traits simultaneously yields more biologically relevant results than the combined results of pairwise analysis of the same traits. Finally, we show that PolarMorphism is more efficient and more powerful than previously published methods.