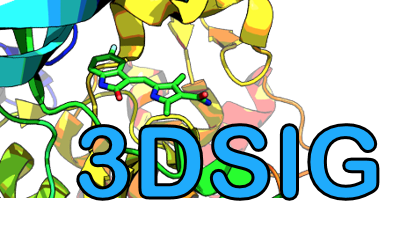
3DSIG
DDAffinity: Predicting the changes in binding affinity of multiple point mutations using protein three-dimensional structure
- Guanglei Yu, Central South University, China
- Qichang Zhao, Central South University, China
- Xuehua Bi, Central South University, China
- Jianxin Wang, Central South University, China
Presentation Overview: Show
Motivation: Mutations are the crucial driving force for biological evolution as they can disrupt protein stability and protein-protein interactions which have notable impacts on protein structure, function, and expression. And the progressive accumulation of multiple point mutations would lead to cancer. However, existing computational methods for protein mutation effects prediction are generally limited to single point mutations with global dependencies, and do not systematically take into account the local and global synergistic epistasis inherent in multiple point mutations.
Results: To this end, we propose a novel spatial and sequential message passing neural network, named DDAffinity, to predict the changes in binding affinity caused by multiple point mutations based on protein three-dimensional (3D) structures. Specifically, instead of being on the whole protein, we perform message passing on the k-nearest neighbour residue graphs to extract pocket features of the protein 3D structures. Furthermore, to learn global topological features, a two-step additive Gaussian noising strategy during training is applied to blur out local details of protein geometry. We evaluate DDAffinity on benchmark datasets and external validation datasets. Overall, the predictive performance of DDAffinity is significantly improved compared with state-of-the-art baselines on multiple point mutations, including end-to-end and pre-training based methods. The ablation studies indicate the reasonable design of all components of DDAffinity. In addition, applications in non-redundant blind testing, predicting mutation effects of SARS-CoV-2 RBD variants, and optimizing human antibody against SARS-CoV-2 illustrate the
effectiveness of DDAffinity. Availability and implementation: DDAffinity is available at https://github.com/ak422/DDAffinity.
Enhancing Generalizability and Performance in Drug-Target Interaction Identification by Integrating Pharmacophore and Pre-trained Models
- Zuolong Zhang, Henan University, China
- Gang Luo, Nanchang University, China
- Shengbo Chen, Henan University, China
- Xin He, Henan University, China
- Dazhi Long, Ji'an Third People's Hospital, China
Presentation Overview: Show
In drug discovery, it is crucial to assess the drug-target binding affinity. Although molecular docking is widely used, computational efficiency limits its application in large-scale virtual screening. Deep learning-based methods learn virtual scoring functions from labeled datasets and can quickly predict affinity. However, there are three limitations. First, existing methods only consider the atom-bond graph or one-dimensional sequence representations of compounds, ignoring the information about functional groups (pharmacophores) with specific biological activities. Second, relying on limited labeled datasets fails to learn comprehensive embedding representations of compounds and proteins, resulting in poor generalization performance in complex scenarios. Third, existing feature fusion methods cannot adequately capture contextual interaction information. Therefore, we propose a novel drug-target binding affinity prediction method named HeteroDTA. Specifically, a multi-view compound feature extraction module is constructed to model the atom-bond graph and pharmacophore graph. The residue concat graph and protein sequence are also utilized to model protein structure and function. Moreover, to enhance the generalization capability and reduce the dependence on task-specific labeled data, pre-trained models are utilized to initialize the atomic features of the compounds and the embedding representations of the protein sequence. A context-aware nonlinear feature fusion method is also proposed to learn interaction patterns between compounds and proteins. Experimental results on public benchmark datasets show that HeteroDTA significantly outperforms existing methods. In addition, HeteroDTA shows excellent generalization performance in cold-start experiments and superiority in the representation learning ability of drug-target pairs. Finally, the effectiveness of HeteroDTA is demonstrated in a real-world drug discovery study.
RiboDiffusion: Tertiary Structure-based RNA Inverse Folding with Generative Diffusion Models
- Han Huang, The Chinese University of Hong Kong, Hong Kong
- Ziqian Lin, Nanjing University, China
- Dongchen He, The Chinese University of Hong Kong, Hong Kong
- Liang Hong, The Chinese University of Hong Kong, Hong Kong
- Yu Li, The Chinese University of Hong Kong, Hong Kong
Presentation Overview: Show
RNA design shows growing applications in synthetic biology and therapeutics, driven by the crucial role of RNA in various biological processes. A fundamental challenge is to find functional RNA sequences that satisfy given structural constraints, known as the inverse folding problem. Computational approaches have emerged to address this problem based on secondary structures. However, designing RNA sequences directly from 3D structures is still challenging, due to the scarcity of data, the non-unique structure-sequence mapping, and the flexibility of RNA conformation. In this study, we propose RiboDiffusion, a generative diffusion model for RNA inverse folding that can learn the conditional distribution of RNA sequences given 3D backbone structures. Our model consists of a graph neural network-based structure module and a Transformer-based sequence module, which iteratively transforms random sequences into desired sequences. By tuning the sampling weight, our model allows for a trade-off between sequence recovery and diversity to explore more candidates. We split test sets based on RNA clustering with different cut-offs for sequence or structure similarity. Our model outperforms baselines in sequence recovery, with an average relative improvement of 11% for sequence similarity splits and 16% for structure similarity splits. Moreover, RiboDiffusion performs consistently well across various RNA length categories and RNA types. We also apply in-silico folding to validate whether the generated sequences can fold into the given 3D RNA backbones. Our method could be a powerful tool for RNA design that explores the vast sequence space and finds novel solutions to 3D structural constraints.
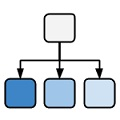
Bio-Ontologies
Integration of Background Knowledge for Automatic Detection of Inconsistencies in Gene Ontology Annotation
- Jiyu Chen, The Commonwealth Scientific and Industrial Research Organisation (CSIRO), Australia
- Benjamin Goudey, The Florey Institute of Neuroscience and Mental Health, Australia
- Nicholas Geard, School of Computing and Information Systems, The University of Melbourne, Australia
- Karin Verspoor, The RMIT University, Australia
Presentation Overview: Show
Biological background knowledge plays an important role in the manual quality assurance (QA) of biological database records. One such QA task is the detection of inconsistencies in literature-based Gene Ontology Annotation (GOA). This manual verification ensures the accuracy of the GOA based on a comprehensive review of the literature used as evidence, Gene Ontology (GO) terms, and annotated genes in GOA records. While automatic approaches for the detection of semantic inconsistencies in GOA have been developed, they operate within predetermined contexts, lacking the ability to leverage broader evidence, especially relevant domain-specific background knowledge. This paper investigates various types of background knowledge that could improve the detection of prevalent inconsistencies in GOA. Additionally, the paper proposes several approaches to integrate background knowledge into the automatic GOA inconsistency detection process.
We extended a previously developed GOA inconsistency dataset with several kinds of GOA-related background knowledge, including GeneRIF statements, biological concepts mentioned within evidence texts, GO hierarchy and existing GO annotations of the specific gene. We proposed several effective approaches to integrate background knowledge as part of the automatic GOA inconsistency detection process. The proposed approaches can improve automatic detection of self-consistency and several of the most prevalent types of inconsistencies.
This is the first study to explore the advantages of utilizing background knowledge and to propose a practical approach to incorporate knowledge in automatic GOA inconsistency detection. We established a new benchmark for performance on this task. Our methods may be applicable to various tasks that involve incorporating biological background knowledge.
Predicting protein functions using positive-unlabeled ranking with ontology-based priors
- Fernando Zhapa-Camacho, King Abdullah University of Science and Technology, Saudi Arabia
- Zhenwei Tang, University of Toronto, Canada
- Maxat Kulmanov, King Abdullah University of Science and Technology, Saudi Arabia
- Robert Hoehndorf, King Abdullah University of Science and Technology, Saudi Arabia
Presentation Overview: Show
Automated protein function prediction is a crucial and widely studied problem in bioinformatics. Computationally, protein function is a multilabel classification problem where only positive samples are defined and there is a large number of unlabeled annotations. Most existing methods rely on the assumption that the unlabeled set of protein function annotations are negatives, inducing the false negative issue, where potential positive samples are trained as negatives. We introduce a novel approach named PU-GO, wherein we address function prediction as a positive-unlabeled ranking problem. We apply empirical risk minimization, i.e., we minimize the classification risk of a classifier where class priors are obtained from the Gene Ontology hierarchical structure. We show that our approach is more robust than other state-of-the-art methods on similarity-based and time-based benchmark datasets. Data and code are available at https://github.com/bio-ontology-research-group/PU-GO.
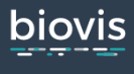
BioVis
Unveil Cis-acting Combinatorial mRNA Motifs by Interpreting Deep Neural Network
- Xiaocheng Zeng, Dept. of Automation, Tsinghua University, China
- Zheng Wei, Tsinghua Univ., China
- Qixiu Du, Department of Automation, Tsinghua University, China
- Jiaqi Li, Tsinghua University, China
- Zhen Xie, Tsinghua University, China
- Xiaowo Wang, Tsinghua University, China
Presentation Overview: Show
Cis-acting mRNA elements play a key role in the regulation of mRNA stability and translation efficiency. Revealing the interactions of these elements and their impact plays a crucial role in understanding the regulation of the mRNA translation process, which supports the development of mRNA-based medicine or vaccines. Deep neural networks (DNN) can learn complex cis-regulatory codes from RNA sequences. However, extracting these cis-regulatory codes efficiently from DNN remains a significant challenge. Here we propose a method based on our toolkit NeuronMotif and motif mutagenesis, which not only enables the discovery of diverse and high-quality motifs but also efficiently reveals motif interactions. By interpreting deep-learning models, we have discovered several crucial motifs that impact mRNA translation efficiency and stability, as well as some unknown motifs or motif syntax, offering novel insights for biologists. Furthermore, we note that it is challenging to enrich motif syntax in datasets composed of randomly generated sequences, and they may not contain sufficient biological signals.
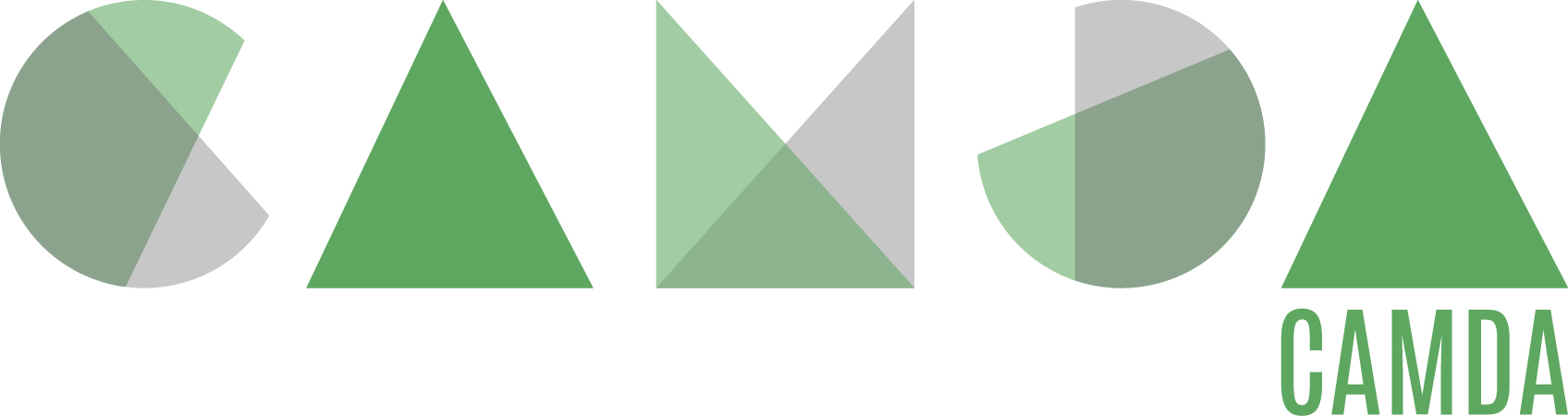
CAMDA
Biomarker identification by interpretable Maximum Mean Discrepancy
- Michael Adamer, ETH Zürich, Switzerland
- Sarah Brüningk, ETH Zürich, Switzerland
- Dexiong Chen, Max Planck Institue of Biochemistry, Germany
- Karsten Borgwardt, Max Planck Institue of Biochemistry, Germany
Presentation Overview: Show
Motivation:In many biomedical applications, we are confronted with paired groups of samples, such as treated vs. control. The aim is to detect discriminating features, i.e. biomarkers, based on high-dimensional (omics-) data. This problem can be phrased more generally as a two-sample problem requiring statistical significance testing to establish differences, and interpretations to identify distinguishing features. The multivariate maximum mean discrepancy (MMD) test quantifies group-level differences, whereas statistically significantly associated features are usually found by univariate feature selection. Currently, there are few general-purpose methods that simultaneously perform multivariate feature selection and two-sample testing.\newline
Results: We introduce a sparse, interpretable, and optimised MMD test (SpInOpt-MMD) that enables two-sample testing and feature selection in the same experiment. SpInOpt-MMD is a versatile method and we demonstrate its application to a variety of synthetic and real-world data types including images, gene expression, and text data. SpInOpt-MMD is effective in identifying relevant features in small sample sizes and outperforms other feature selection methods such as SHapley Additive exPlanations (SHAP) and univariate association analysis in several experiments.
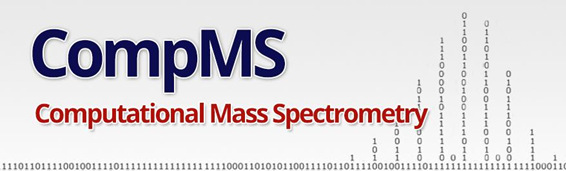
CompMS
A learned score function improves the power of mass spectrometry database search
- Varun Ananth, University of Washington, United States
- Justin Sanders, University of Washington, United States
- Melih Yilmaz, University of Washington, United States
- Bo Wen, University of Washington, United States
- Sewoong Oh, University of Washington, United States
- William Stafford Noble, University of Washington, United States
Presentation Overview: Show
One of the core problems in the analysis of protein tandem mass spectrometry data is the peptide assignment problem: determining, for each observed spectrum, the peptide sequence that was responsible for generating the spectrum. Two primary classes of methods are used to solve this problem: database search and de novo peptide sequencing. State-of-the-art methods for de novo sequencing employ machine learning methods, whereas most database search engines use hand-designed score functions to evaluate the quality of a match between an observed spectrum and a candidate peptide from the database. We hypothesize that machine learning models for de novo sequencing implicitly learn a score function that captures the relationship between peptides and spectra, and thus may be re-purposed as a score function for database search. Because this score function is trained from massive amounts of mass spectrometry data, it could potentially outperform existing, hand-designed database search tools. To test this hypothesis, we re-engineered Casanovo, which has been shown to provide state-of-the-art de novo sequencing capabilities, to assign scores to given peptide-spectrum pairs. We then evaluated the statistical power of this Casanovo score function, dubbed Casanovo-DB, to detect peptides on a benchmark of three mass spectrometry runs from three different species. Our results show that, at a 1% peptide-level false discovery rate threshold, Casanovo-DB outperforms existing hand-designed score functions by 35% to 88%. In addition, we show that re-scoring with the Percolator post-processor benefits Casanovo-DB more than other score functions, further increasing the number of detected peptides.
An algorithm for decoy-free false discovery rate estimation in XL-MS/MS
- Yisu Peng, Northeastern University, United States
- Shantanu Jain, Northeastern University, United States
- Predrag Radivojac, Northeastern University, United States
Presentation Overview: Show
Motivation: Cross-linking tandem mass spectrometry (XL-MS/MS) proteomics is an established technique that determines distance constraints between residues within a protein or between interacting proteins, thus improving our understanding of protein structure and function under native cellular conditions. To aid biological discovery, it is essential that pairs of chemically linked peptides be accurately identified, a process that requires: (i) database search, that creates a ranked list of candidate peptide pairs for each experimental spectrum, and (ii) false discovery rate (FDR) estimation, that determines the probability of false identification of the top-ranked peptide pairs for a given score threshold. Currently, the only available FDR estimation mechanism in XL-MS/MS is the target-decoy approach (TDA). However, despite its simplicity, TDA has both theoretical and practical drawbacks.
Results: We introduce a novel decoy-free framework for FDR estimation in XL-MS/MS. Our approach relies on multi-sample mixtures of skew normal distributions, where the latent components correspond to the scores of correct peptide pairs (both peptides identified correctly), partially incorrect peptide pairs (one peptide identified correctly, the other incorrectly), and incorrect peptide pairs (both peptides identified incorrectly). To learn these components, we exploit the score distributions of first- and second-ranked peptide-spectrum matches (PSMs) for each experimental spectrum and subsequently estimate FDR using a novel expectation-maximization (EM) algorithm with constraints. We evaluate the method on ten datasets and provide evidence that the proposed DFA is theoretically sound and a viable alternative to TDA owing to its good performance in terms of accuracy, variance of estimation, and run time.
SpecEncoder: Deep Metric Learning for Accurate Peptide Identification in Proteomics
- Kaiyuan Liu, Indiana University Bloomington, United States
- Chenghua Tao, Indiana University Bloomington, United States
- Yuzhen Ye, Indiana University Bloomington, United States
- Haixu Tang, Indiana University Bloomington, United States
Presentation Overview: Show
Tandem mass spectrometry (MS/MS) is a crucial technology for large-scale proteomic analysis. The protein database search or the spectral library search are commonly used for peptide identification from MS/MS spectra, which, however, may face challenges due to experimental variations between replicated spectra and similar fragmentation patterns among distinct peptides. To address this challenge, we present SpecEncoder, a deep metric learning approach to address these challenges by transforming MS/MS spectra into robust and sensitive embedding vectors in a latent space. The SpecEncoder model can also embed predicted MS/MS spectra of peptides, enabling a hybrid search approach that combines spectral library and protein database searches for peptide identification. We evaluated SpecEncoder on three large human proteomics datasets, and the results showed a consistent improvement in peptide identification. For spectral library search, SpecEncoder identifies ~1-2% more unique peptides (and PSMs) than SpectraST. For protein database search, it identifies 6-15% more unique peptides than MSGF+ enhanced by Percolator, Furthermore, SpecEncoder identified 6-12% additional unique peptides when utilizing a combined library of experimental and predicted spectra. SpecEncoder can also identify more peptides when compared to deep-learning enhanced methods (MSFragger boosted by MSBooster). These results demonstrate SpecEncoder's potential to enhance peptide identification for proteomic data analyses.
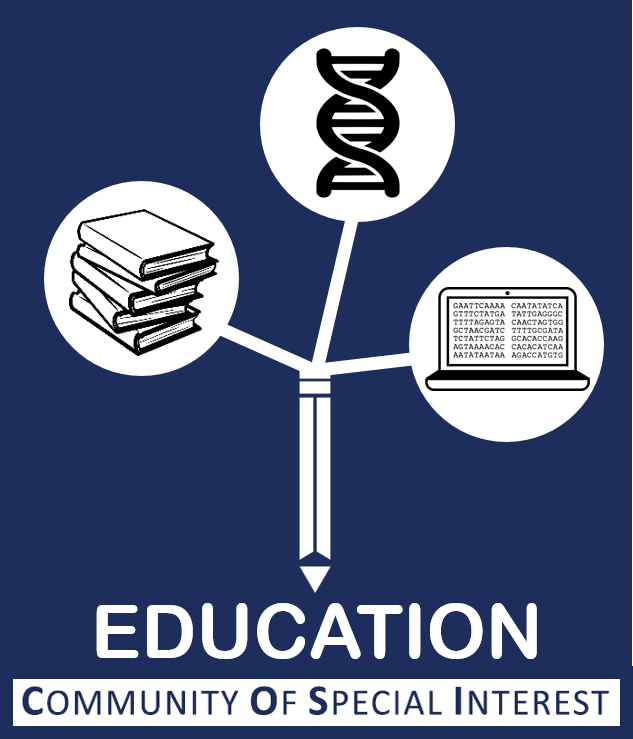
Education
Closing the computational biology “knowledge gap”: Spanish Wikipedia as a case study
- Nelly Sélem-Mojica, Centro de Ciencias Matemáticas, Universidad Nacional Autónoma de México, Mexico
- Tülay Karakulak, Department of Molecular Life Sciences and Swiss Institute of Bioinformatics, University of Zurich, Switzerland
- Audra Anjum, Office of Instructional Design, Ohio University, United States
- Antón Pashkov, Escuela Nacional de Estudios Superiores (ENES) Unidad Morelia, Universidad Nacional Autónoma de México, Mexico
- Rafael Pérez Estrada, Escuela Nacional de Estudios Superiores (ENES) Unidad Morelia, Universidad Nacional Autónoma de México, Mexico
- Karina Enriquez-Guillén, Escuela Nacional de Estudios Superiores (ENES) Unidad Morelia, Universidad Nacional Autónoma de México, Mexico
- Dan DeBlasio, Ray and Stephanie Lane Computational Biology Department, Carnegie Mellon University, United States
- Sofia Ferreira-Gonzalez, Centre for Inflammation Research, Institute for Regeneration and Repair, The University of Edinburgh, United Kingdom
- Alejandra Medina-Rivera, Laboratorio Internacional de Investigación sobre el Genoma Humano, Universidad Nacional Autónoma de México, Mexico
- Daniel Rodrigo-Torres, Centre for Regenerative Medicine, Institute for Regeneration and Repair, The University of Edinburgh, United Kingdom
- Alastair M. Kilpatrick, Centre for Regenerative Medicine, Institute for Regeneration and Repair, The University of Edinburgh, United Kingdom
- Lonnie R. Welch, School of Electrical Engineering and Computer Science, Ohio University, United States
- Farzana Rahman, School of Computing and Mathematics, Faculty of Engineering, Computing, and Environment, Kingston University London, United Kingdom
Presentation Overview: Show
Motivation: Wikipedia is a vital open educational resource in computational biology. The quality of computational biology coverage in English Wikipedia has improved steadily in recent years. However, there is an increasingly large ‘knowledge gap’ between computational biology resources in English Wikipedia, and Wikipedias in non-English languages. Reducing this knowledge gap by providing educational resources in non-English languages would reduce language barriers which disadvantage non-native English speaking learners across multiple dimensions in computational biology.
Results: Here, we provide a comprehensive assessment of computational biology coverage in Spanish Wikipedia, the second most accessed Wikipedia worldwide. Using Spanish Wikipedia as a case study, we generate quantitative and qualitative data before and after a targeted educational event, specifically, a Spanish-focused student editing competition. Our data demonstrates how such events and activities can narrow the knowledge gap between English and non-English educational resources, by improving existing articles and creating new articles. Finally, based on our analysis, we suggest ways to prioritise future initiatives to improve open educational resources in other languages.
Teaching Bioinformatics through the Analysis of SARS-CoV-2: Project-Based Training for Computer Science Students
- Pavlin G. Poličar, University of Ljubljana, Faculty of Computer and Information Science, Slovenia
- Martin Špendl, University of Ljubljana, Faculty of Computer and Information Science, Slovenia
- Tomaž Curk, University of Ljubljana, Faculty of Computer and Information Science, Slovenia
- Blaž Zupan, University of Ljubljana, Faculty of Computer and Information Science, Slovenia
Presentation Overview: Show
We learn more effectively through experience and reflection than through passive reception of information. Bioinformatics offers an excellent opportunity for project-based learning. Molecular data is abundant and accessible in open repositories, and important concepts in biology can be rediscovered by reanalyzing the data. In the manuscript, we report on five hands-on assignments we designed for master’s computer science students to train them in bioinformatics. These assignments are the cornerstones of our introductory bioinformatics course and are centered around the study of the SARS-CoV-2 virus. They assume no prior knowledge of molecular biology but do require programming skills. Through these assignments students learn about genomes and genes, discover their composition and function, relate SARS-CoV-2 to other viruses, and learn about the body’s response to infection. Student evaluation of the assignments confirms their usefulness and value, their appropriate mastery-level difficulty, and their interesting and motivating storyline.
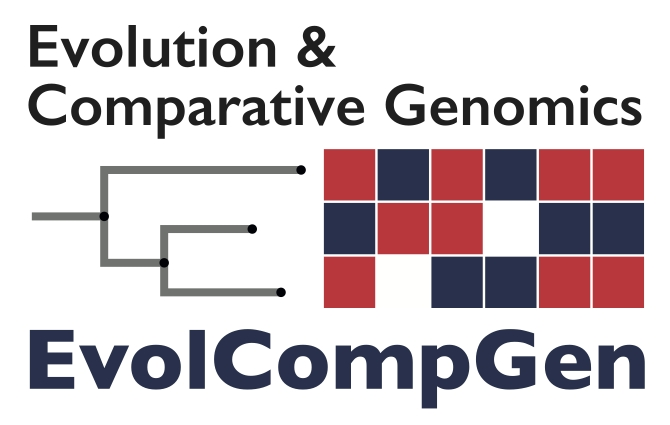
EvolCompGen
A machine-learning based alternative to phylogenetic bootstrap
- Noa Ecker, Tel Aviv University, Israel
- Tal Pupko, Tel Aviv University, Israel
- Itay Mayrose, Tel Aviv University, Israel
- Yishay Mansour, Tel Aviv University, Israel
- Dorothée Huchon, Tel Aviv University, Israel
Presentation Overview: Show
Currently used methods for estimating branch support in phylogenetic analyses often rely on the classic Felsenstein's bootstrap, parametric tests, or their approximations. As these branch support scores are widely used in phylogenetic analyses, having accurate, fast, and interpretable scores is of high importance.
Here, we employed a data-driven approach to estimate branch support values with a probabilistic interpretation. To this end, we simulated thousands of realistic phylogenetic trees and the corre-sponding multiple sequence alignments. Each of the obtained alignments was used to infer the phylogeny using state-of-the-art phylogenetic inference software, which was then compared to the true tree. Using these extensive data, we trained machine-learning algorithms to estimate branch support values for each bipartition within the maximum-likelihood trees obtained by each software. Our results demonstrate that our model provides fast and more accurate probability-based branch support values than commonly used procedures.
Joint inference of cell lineage and mitochondrial evolution from single-cell sequencing data
- Palash Sashittal, University of Illinois at Urbana-Champaign, United States
- Viola Chen, Princeton University, United States
- Amey Pasarkar, Princeton University, United States
- Ben Raphael, Princeton University, United States
Presentation Overview: Show
Eukaryotic cells contain organelles called mitochondria that have their own genome. Most cells contain thousands of mitochondria which replicate, even in non-dividing cells, by means of a relatively error-prone process resulting in somatic mutations in their genome. Because of the higher mutation rate compared to the nuclear genome, mitochondrial mutations have been used to track cellular lineage, particularly using single-cell sequencing that measures mitochondrial mutations in individual cells. However, existing methods to infer the cell lineage tree from mitochondrial mutations do not model heteroplasmy, which is the presence of multiple mitochondrial clones with distinct sets of mutations in an individual cell. Single-cell sequencing data thus provides a mixture of the mitochondrial clones in individual cells, with the ancestral relationships between these clones described by a mitochondrial clone tree that must be concordant with the cell lineage tree. We formalize the problem of inferring a concordant pair of a mitochondrial clone tree and a cell lineage tree from single-cell sequencing data as the NESTED PERFECT PHYLOGENY MIXTURE (NPPM) problem. We derive an algorithm, MERLIN, to solve the NPPM problem. We show on simulated data that MERLIN outperforms existing methods that do not model mitochondrial heteroplasmy nor the concordance between the mitochondrial clone tree and the cell lineage tree. We use MERLIN to analyze single-cell whole genome sequencing data of 5220 cells of a gastric cancer cell line and show that MERLIN infers a more biologically plausible cell lineage tree and mitochondrial clone tree compared to existing methods.
Maximum Likelihood Phylogeographic Inference of Cell Motility and Cell Division from Spatial Lineage Tracing Data
- Uyen Mai, Princeton University, United States
- Gary Hu, Princeton University, United States
- Ben Raphael, Princeton University, United States
Presentation Overview: Show
Recently developed spatial lineage tracing technologies induce somatic mutations at specific genomic loci in growing cells and then measure these mutations in the sampled cells along with their physical locations. These technologies enable high-throughput studies of developmental processes over space and time. However, these applications rely on accurate reconstruction of a spatial cell lineage tree describing the history of bothcell divisions and locations. We demonstrate that standard phylogeographic models based on Brownian motion are inadequate to describe the symmetric spatial displacement of cells during cell division. We introduce a new model for cell motility that includes symmetric displacements of daughter cells from the parental cell followed by independent diffusion of daughter cells. We show that this model more accurately describes the locations of cells in a real spatial lineage tracing of Drosophila melanogaster embryos. Combining the spatial model with an evolutionary model of DNA mutations, we obtain a comprehensive model for spatial lineage tracing, namely spalin. Using this model, we estimate time-resolved branch lengths, spatial diffusion rate, and mutation rate. On both simulated and real data, we show that the proposed method accurately estimates all parameters while the Brownian motion model overestimates spatial diffusion rate in all test cases. In addition, the inclusion of spatial information improves accuracy of branch length estimation compared to sequence data alone, suggesting augmenting lineage tracing technologies with spatial information is useful to overcome the limitations of genome-editing in developmental systems.
Median and Small Parsimony Problems on RNA trees
- Bertrand Marchand, Université de Sherbrooke, Canada
- Yoann Anselmetti, University of Sherbrooke, Canada
- Manuel Lafond, Université de Sherbrooke, Canada
- Aida Ouangraoua, Université de Sherbrooke, Canada
Presentation Overview: Show
Motivation:
Non-coding RNAs (ncRNAs) express their functions by adopting molecular structures. Specifically, RNA secondary structures serve as a relatively stable intermediate step before tertiary structures, offering a reliable signature of molecular function. Consequently, within an RNA functional family, secondary structures are generally more evolutionarily conserved than sequences. Conversely, homologous RNA families grouped within an RNA clan share ancestors but typically exhibit structural differences. Inferring the evolution of RNA structures within RNA families and clans is crucial for gaining insights into functional adaptations over time and providing clues about the Ancient RNA World Hypothesis.
Results:
We introduce the median problem and the small parsimony problem for ncRNA families, where secondary structures are represented as leaf-labelled trees. We utilize the Robinson-Foulds (RF) tree distance, which corresponds to a specific edit distance between RNA trees, and a new metric called the Internal-Leafset (IL) distance. While the RF tree distance compares sets of leaves descending from internal nodes of two RNA trees, the IL distance compares the collection of leaf-children of internal nodes. The latter is better at capturing differences in structural elements of RNAs than the RF distance, which is more focused on base pairs. We study the theoretical complexity of the median problem and the small parsimony problem under the three distance metrics and various biologically-relevant constraints, and we present polynomial-time maximum parsimony algorithms for solving some versions of the problems. Our algorithms are applied to ncRNA families from the RFAM database, illustrating their practical utility.
GenCompBio
An Empirical Study on KDIGO-Defined Acute Kidney Injury Prediction in the Intensive Care Unit
- Xinrui Lyu, Department of Computer Science, ETH Zürich, Switzerland, Switzerland
- Bowen Fan, Department of Biosystems Science and Engineering, ETH Zürich, Switzerland, Switzerland
- Matthias Hüser, Department of Computer Science, ETH Zürich, Switzerland, Switzerland
- Philip Hartout, Department of Biosystems Science and Engineering, ETH Zürich, Switzerland, Switzerland
- Thomas Gumbsch, Department of Biosystems Science and Engineering, ETH Zürich, Switzerland, Germany
- Martin Faltys, Department of Intensive Care, Austin Hospital, Melbourne, Australia, Australia
- Tobias Merz, Cardiovascular Intensive Care Unit, Auckland City Hospital, New Zealand, Switzerland
- Gunnar Rätsch, Department of Computer Science, ETH Zürich, Switzerland, Switzerland
- Karsten Borgwardt, Department of Biosystems Science and Engineering, ETH Zürich, Switzerland, Switzerland
Presentation Overview: Show
Motivation: Acute kidney injury (AKI) is a syndrome that affects up to a third of all critically ill patients, and early diagnosis to receive adequate treatment is as imperative as it is challenging to make early. Consequently, machine learning approaches have been developed to predict AKI ahead of time. However, the prevalence of AKI is often underestimated in state-of-the-art approaches, as they rely on an AKI event annotation solely based on creatinine, ignoring urine output.
Methods: We construct and evaluate early warning systems for AKI in a multi-disciplinary ICU setting, using the complete KDIGO definition of AKI. We propose several variants of gradient-boosted decision trees (GDBT)-based models, including a novel time-stacking based approach. A state-of-the-art LSTM-based model previously proposed for AKI prediction is used as a comparison, which was not specifically evaluated in ICU settings yet.
Results: We find that optimal performance is achieved by using GBDT with the time-based stacking technique (AUPRC=65.7%, compared with the LSTM-based model's AUPRC=62.6%), which is motivated by the high relevance of time since ICU admission for this task. Both models show mildly reduced performance in the limited training data setting, perform fairly across different subcohorts, and exhibit no issues in gender transfer.
Conclusion: Following the official KDIGO definition substantially increases the number of annotated AKI events. In our study GBDTs outperform LSTM models for AKI prediction. Generally, we find that both model types are robust in a variety of challenging settings arising in the ICU.
Approximating facial expression effects on diagnostic accuracy via generative AI
- Tanviben Patel, Medical Genomics Unit, Medical Genetics Branch, NHGRI, Bethesda, 20892, Maryland, USA, United States
- Amna Othman, Medical Genomics Unit, Medical Genetics Branch, NHGRI, Bethesda, 20892, Maryland, USA, United States
- Omer Sumer, Institute of Computer Science, Augsburg University, Augsburg, 86159, Bavaria, Germany, Germany
- Fabio Hellman, Institute of Computer Science, Augsburg University, Augsburg, 86159, Bavaria, Germany, Germany
- Peter Krawitz, Institute for Genomic Statistics and Bioinformatics, University of Bonn, Germany, Germany
- Elisabeth Andre, Institute of Computer Science, Augsburg University, Augsburg, 86159, Bavaria, Germany, Germany
- Molly E. Ripper, Medical Genomics Unit, Medical Genetics Branch, NHGRI, Bethesda, 20892, Maryland, USA, United States
- Chris Fortney, Social and Behavioral Research Branch, NHGRI, Bethesda, 20892, Maryland, USA, United States
- Susan Persky, Social and Behavioral Research Branch, NHGRI, Bethesda, 20892, Maryland, USA, United States
- Ping Hu, Medical Genomics Unit, Medical Genetics Branch, NHGRI, Bethesda, 20892, Maryland, USA, United States
- Cedrik Tekendo-Ngongang, Medical Genomics Unit, Medical Genetics Branch, NHGRI, Bethesda, 20892, Maryland, USA, United States
- Suzanna Ledgister Hanchard, Medical Genomics Unit, Medical Genetics Branch, NHGRI, Bethesda, 20892, Maryland, USA, United States
- Kendall A. Flaharty, Medical Genomics Unit, Medical Genetics Branch, NHGRI, Bethesda, 20892, Maryland, USA, United States
- Rebekah L. Waikel, Medical Genomics Unit, Medical Genetics Branch, NHGRI, Bethesda, 20892, Maryland, USA, United States
- Dat Duong, Medical Genomics Unit, Medical Genetics Branch, NHGRI, Bethesda, 20892, Maryland, USA, United States
- Benjamin D. Solomon, Medical Genomics Unit, Medical Genetics Branch, NHGRI, Bethesda, 20892, Maryland, USA, United States
Presentation Overview: Show
Artificial Intelligence (AI) is increasingly used in genomics research and practice, and generative AI has garnered significant recent attention. In clinical applications of generative AI, aspects of the underlying datasets can impact results, and confounders should be studied and mitigated. One example involves the facial expressions of people with genetic conditions. Stereotypically, Williams (WS) and Angelman (AS) syndromes are associated with a “happy” demeanor, including a smiling expression. Clinical geneticists may be more likely to identify these conditions in images of smiling individuals. To study the impact of facial expression, we analyzed publicly available facial images of approximately 3500 individuals with genetic conditions. Using a deep learning (DL) image classifier, we found that WS and AS images with non-smiling expressions had significantly lower prediction probabilities for the correct syndrome labels than those with smiling expressions. This was not seen for 22q11.2 deletion and Noonan syndromes, which are not associated with a smiling expression. To further explore the effect of facial expressions, we computationally altered the facial expressions for these images. We trained HyperStyle, a GAN-inversion technique compatible with StyleGAN2, to determine the vector representations of our images. Then, following the concept of InterfaceGAN, we edited these vectors to recreate the original images in a phenotypically accurate way but with a different facial expression. Through online surveys and an eye-tracking experiment, we examined how altered facial expressions affect the performance of human experts. We overall found that facial expression is associated with diagnostic accuracy variably in different genetic conditions.
Efficient parameter estimation for ODE models of cellular processes using semi-quantitative data
- Domagoj Doresic, IRU Mathematics and Life Sciences, University of Bonn; Helmholtz Zentrum München, Computational Health Center, Germany
- Stephan Grein, IRU Mathematics and Life Sciences, University of Bonn, Germany
- Jan Hasenauer, IRU Mathematics and Life Sciences, University of Bonn; Technische Universität München; Helmholtz Zentrum München, Germany
Presentation Overview: Show
Quantitative dynamical models facilitate the understanding of biological processes and the prediction of their dynamics. The parameters of these models are commonly estimated from experimental data. Yet, experimental data generated from different techniques do not provide direct information about the state of the system but a non-linear (monotonic) transformation of it. For such semi-quantitative data, when this transformation is unknown, it is not apparent how the model simulations and the experimental data can be compared. Here, we propose a versatile spline-based approach for the integration of a broad spectrum of semi-quantitative data into parameter estimation. We derive analytical formulas for the gradients of the hierarchical objective function and show that this substantially increases the estimation efficiency. Subsequently, we demonstrate that the method allows for the reliable discovery of unknown measurement transformations. Furthermore, we show that this approach can significantly improve the parameter inference based on semi-quantitative data in comparison to available methods. Modelers can easily apply our method by using our implementation in the open-source Python Parameter EStimation TOolbox (pyPESTO).
Optimal Phylogenetic Reconstruction of Insertion and Deletion Events.
- Sanjana Tule, The University of Queensland, Australia
- Gabriel Foley, The University of Queensland, Australia
- Chongting Zhao, The University of Queensland, Australia
- Michael Forbes, The University of Queensland, Australia
- Mikael Boden, The University of Queensland, Australia
Presentation Overview: Show
Insertions and deletions (indels) influence the genetic code in fundamentally distinct ways from substitutions, significantly impacting gene product structure and function. Despite their influence, the evolutionary history of indels is often neglected in phylogenetic tree inference and ancestral sequence reconstruction, hindering efforts to comprehend biological diversity determinants and engineer variants for medical and industrial applications.
We frame determining the optimal history of indel events as a single Mixed-Integer Programming (MIP) problem, across all branch points in a phylogenetic tree adhering to topological constraints, and all sites implied by a given set of aligned, extant sequences. By disentangling the impact on ancestral sequences at each branch point, this approach identifies the minimal indel events that jointly explain the diversity in sequences mapped to the tips of that tree. MIP can recover alternate optimal indel histories, if available.
We evaluated MIP for indel inference on a dataset comprising 15 real phylogenetic trees associated with protein families ranging from 165 to 2000 extant sequences, and on 60 synthetic trees at comparable scales of data and reflecting realistic rates of mutation. Across relevant metrics, MIP outperformed alternative parsimony-based approaches and reported the fewest indel events, on par or below their occurrence in synthetic datasets. MIP offers a rational justification for indel patterns in extant sequences; importantly, it uniquely identifies global optima on complex protein data sets without making unrealistic assumptions of independence or evolutionary underpinnings, promising a deeper understanding of molecular evolution and aiding novel protein design.
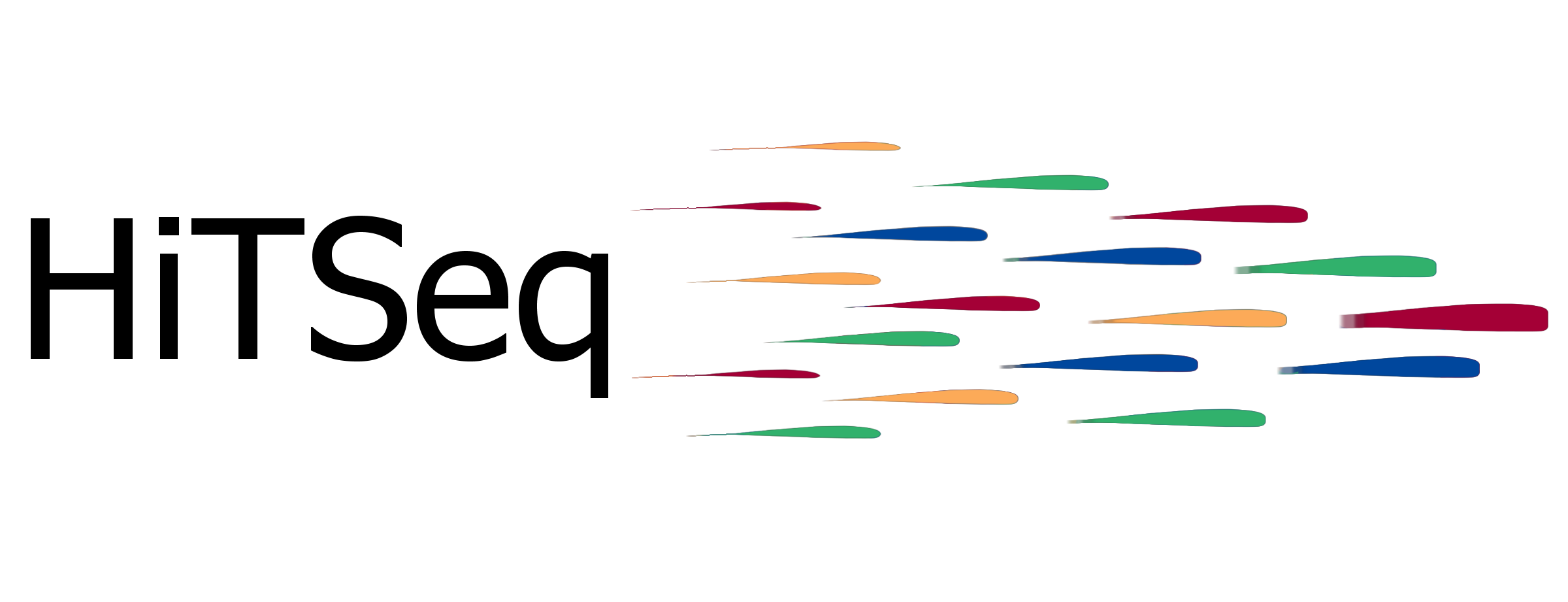
HiTSeq
Adaptive Digital Tissue Deconvolution
- Franziska Görtler, Department of Oncology and Medical Physics, Haukeland University Hospital, Norway
- Malte Mensching-Buhr, Department of Medical Bioinformatics, University Medical Center Göttingen, Germany
- Ørjan Skaar, Computational Biology Unit, University of Bergen, Norway
- Stefan Schrod, University Medical Center Göttingen, Germany
- Thomas Sterr, Institute of Theoretical Physics, University of Regensburg, Germany
- Andreas Schäfer, Institute of Theoretical Physics, University of Regensburg, Germany
- Tim Beissbarth, University Medicine Göttingen, Germany
- Anagha Joshi, Department of Clinical Science, Computational Biology Unit, University of Bergen, Norway
- Helena U. Zacharias, University Medical Center Schleswig-Holstein; Kiel University, Germany
- Sushma Nagaraja Grellscheid, Computational Biology Unit, University of Bergen, Norway
- Michael Altenbuchinger, Department of Medical Bioinformatics, University Medical Center Göttingen, Germany
Presentation Overview: Show
Motivation: The inference of cellular compositions from bulk and spatial transcriptomics data increasingly complements data analyses. Multiple computational approaches were suggested and recently, machine learning techniques were developed to systematically improve estimates. Such approaches allow to infer additional, less abundant cell types. However, they rely on training data which do not capture the full biological diversity encountered in transcriptomics analyses; data can contain cellular contributions not seen in the training data and as such, analyses can be biased or blurred. Thus, computational approaches have to deal with unknown, hidden contributions. Moreover, most methods are based on cellular archetypes which serve as a reference; e.g., a generic T-cell profile is used to infer the proportion of T-cells. It is well known that cells adapt their molecular phenotype to the environment and that pre-specified cell archetypes can distort the inference of cellular compositions.
Results: We propose Adaptive Digital Tissue Deconvolution (ADTD) to estimate cellular proportions of pre-selected cell types together with possibly unknown and hidden background contributions. Moreover, ADTD adapts prototypic reference profiles to the molecular environment of the cells, which further resolves cell-type specific gene regulation from bulk transcriptomics data. We verify this in simulation studies and demonstrate that ADTD improves existing approaches in estimating cellular compositions. In an application to bulk transcriptomics data from breast cancer patients, we demonstrate that ADTD provides insights into cell-type specific molecular differences between breast cancer subtypes.
Availability and implementation: A python implementation of ADTD and a tutorial are available at https://doi.org/10.5281/zenodo.7548362 (doi:10.5281/zenodo.7548362).
Conway-Bromage-Lyndon (CBL): an exact, dynamic representation of k-mer sets
- Igor Martayan, Univ Lille, France
- Bastien Cazaux, Univ. Lille, CNRS, Centrale Lille, UMR 9189 CRIStAL, F-59000 Lille, France, France
- Antoine Limasset, CNRS, France
- Camille Marchet, CNRS, France
Presentation Overview: Show
In this paper, we introduce the Conway-Bromage-Lyndon (CBL) structure, a compressed, dynamic and exact method for
representing k-mer sets. Originating from Conway and Bromage’s concept, CBL innovatively employs the smallest cyclic
rotations of k-mers, akin to Lyndon words, to leverage lexicographic redundancies. In order to support dynamic operations
and set operations, we propose a dynamic bit vector structure that draws a parallel with Elias-Fano’s scheme. This
structure is encapsulated in a Rust library, demonstrating a balanced blend of construction efficiency, cache locality, and
compression. Our findings suggest that CBL outperforms existing k-mer set methods, particularly in dynamic scenarios.
Unique to this work, CBL stands out as the only known exact k-mer structure offering in-place set operations. Its different
combined abilities positions it as a flexible Swiss knife structure for k-mer set management.
Fast Multiple Sequence Alignment via Multi-Armed Bandits
- Kayvon Mazooji, University of Illinois Urbana-Champaign, United States
- Ilan Shomorony, University of Illinois at Urbana-Champaign, United States
Presentation Overview: Show
Multiple sequence alignment is an important problem in computational biology with applications that include phylogeny and the detection of remote homology between protein sequences. UPP is a popular software package that constructs accurate multiple sequence alignments for large datasets based on ensembles of Hidden Markov Models (HMMs). A computational bottleneck for this method is a sequence-to-HMM assignment step, which relies on the precise computation of probability scores on the HMMs. In this work, we show that we can speed up this assignment step significantly by replacing these HMM probability scores with alternative scores that can be efficiently estimated. Our proposed approach utilizes a Multi-Armed Bandit algorithm to adaptively and efficiently compute estimates of these scores. This allows us to achieve similar alignment accuracy as UPP with a significant reduction in computation time.
Forseti: A mechanistic and predictive model of the splicing status of scRNA-seq reads
- Dongze He, University of Maryland, College Park, United States
- Yuan Gao, University of Maryland, College Park, United States
- Spencer Skylar Chan, University of Maryland, College Park, United States
- Natalia Quintana-Parrilla, University of Puerto Rico, Mayagüez Campus, United States
- Rob Patro, University of Maryland, College Park, United States
Presentation Overview: Show
Motivation: Short-read single-cell RNA-sequencing (scRNA-seq) has been used to study cellular heterogeneity, cellular fate, and transcriptional dynamics. Modeling splicing dynamics in scRNA-seq data is challenging, with inherent difficulty in even the seemingly straightforward task of elucidating the splicing status of the molecules from which the underlying sequenced fragments are drawn. This difficulty arises, in part, from the limited read length and positional biases, which substantially reduce the specificity of the sequenced fragments. As a result, the splicing status of many reads in scRNA-seq is ambiguous because of a lack of definitive evidence. We are therefore in need of methods that can recover the splicing status of ambiguous reads which, in turn, can lead to more accuracy and confidence in downstream analyses.
Results: We develop Forseti, a predictive model to probabilistically assign a splicing status to scRNA-seq reads. Our model has two key components. First, we train a binding affinity model to assign a probability that a given transcriptomic site is
used in fragment generation. Second, we fit a robust fragment length distribution model that generalizes well across datasets deriving from different species and tissue types. Forseti combines these two trained models to predict the splicing status of the molecule of origin of reads by scoring putative fragments that associate each alignment of sequenced reads with proximate potential priming sites. Using both simulated and experimental data, we show that our model can precisely predict the splicing status of reads and identify the true gene origin of multi-gene mapped reads.
Label-guided seed-chain-extend alignment on annotated De Bruijn graphs
- Harun Mustafa, ETH Zurich, Switzerland
- Mikhail Karasikov, ETH Zurich, Switzerland
- Nika Mansouri Ghiasi, ETH Zurich, Switzerland
- Gunnar Rätsch, ETH Zürich, Department for Computer Science, Switzerland
- André Kahles, ETH Zurich, Switzerland
Presentation Overview: Show
Motivation: Exponential growth in sequencing databases has motivated scalable De Bruijn graph-based (DBG) indexing for searching these data, using annotations to label nodes with sample IDs. Low-depth sequencing samples correspond to fragmented subgraphs, complicating finding the long contiguous walks required for alignment queries. Aligners that target single-labelled subgraphs reduce alignment lengths due to fragmentation, leading to low recall for long reads. While some (e.g., label-free) aligners partially overcome fragmentation by combining information from multiple samples, biologically-irrelevant combinations in such approaches can inflate the search space or reduce accuracy.
Results: We introduce a new scoring model, multi-label alignment (MLA), for annotated DBGs. MLA leverages two new operations: To promote biologically-relevant sample combinations, Label Change incorporates more informative global sample similarity into local scores. To improve connectivity, Node Length Change dynamically adjusts the DBG node length during traversal. Our fast, approximate, yet accurate MLA implementation has two key steps: a single-label seed-chain-extend aligner (SCA) and a multi-label chainer (MLC). SCA uses a traditional scoring model adapting recent chaining improvements to assembly graphs and provides a curated pool of alignments.
MLC extracts seeds from SCA’s alignments, produces multi-label chains using MLA scoring, then finally forms multi-label alignments. We show via substantial improvements in taxonomic classification accuracy that MLA produces biologically-relevant alignments, decreasing average weighted UniFrac errors by 63.1–66.8% and covering 45.5–47.4% (median) more long-read query characters than state-of-the-art aligners. MLA’s runtimes are competitive with label-free alignment and substantially faster than single-label alignment.
Availability: https://github.com/ratschlab/mla.
Learning Locality-Sensitive Bucketing Functions
- Xin Yuan, Pennsylvania State University, United States
- Ke Chen, Pennsylvania State University, United States
- Xiang Li, Pennsylvania State University, United States
- Qian Shi, Pennsylvania State University, United States
- Mingfu Shao, Pennsylvania State University, United States
Presentation Overview: Show
Many tasks in sequence analysis ask to identify biologically related sequences in a large set. Edit distance is widely used in these tasks as a measure. To avoid all-vs-all pairwise comparisons and save on expensive edit distance computations, locality-sensitive bucketing (LSB) functions have been proposed. Formally, a (d1,d2)-LSB function sends sequences into multiple buckets with the guarantee that pairs of sequences of edit distance at most d1 can be found within a same bucket while those of edit distance at least d2 do not share any. LSB functions generalize the locality-sensitive hashing (LSH) functions and admit favorable properties, making them potentially ideal solutions to the above problem. But constructing LSB functions for practical use is scarcely possible. In this work, we aim to utilize machine learning techniques to train LSB functions. With the development of a novel loss function and insights in the neural network structures that can extend beyond this specific task, we obtained LSB functions that exhibit nearly perfect accuracy for certain (d1,d2). Comparing to the state-of-the-art method OMH, the trained LSB functions achieve a 2- to 5-fold improvement on the sensitivity of recognizing similar sequences. An experiment on analyzing erroneous cell barcode data is also included to demonstrate the application of the trained LSB functions.
Sigmoni: classification of nanopore signal with a compressed pangenome index
- Vikram Shivakumar, Johns Hopkins University, United States
- Omar Ahmed, Johns Hopkins University, United States
- Sam Kovaka, Johns Hopkins University, United States
- Mohsen Zakeri, Johns Hopkins University, United States
- Ben Langmead, Johns Hopkins University, United States
Presentation Overview: Show
Improvements in nanopore sequencing necessitate efficient classification methods, including pre-filtering and adaptive sampling algorithms that enrich for reads of interest. Signal-based approaches circumvent the computational bottleneck of basecalling. But past methods for signal-based classification do not scale efficiently to large, repetitive references like pangenomes, limiting their utility to partial references or individual genomes. We introduce Sigmoni: a rapid, multiclass classification method based on the r-index that scales to references of hundreds of Gbps. Sigmoni quantizes nanopore signal into a discrete alphabet of picoamp ranges. It performs rapid, approximate matching using matching statistics, classifying reads based on distributions of picoamp matching statistics and co-linearity statistics, all in linear query time without the need for seed-chain-extend. Sigmoni is 10-100× faster than previous methods for adaptive sampling in host depletion experiments with improved accuracy, and can query reads against large microbial or human pangenomes. Sigmoni is the first signal-based tool to scale to a complete human genome and pangenome while remaining fast enough for adaptive sampling applications.
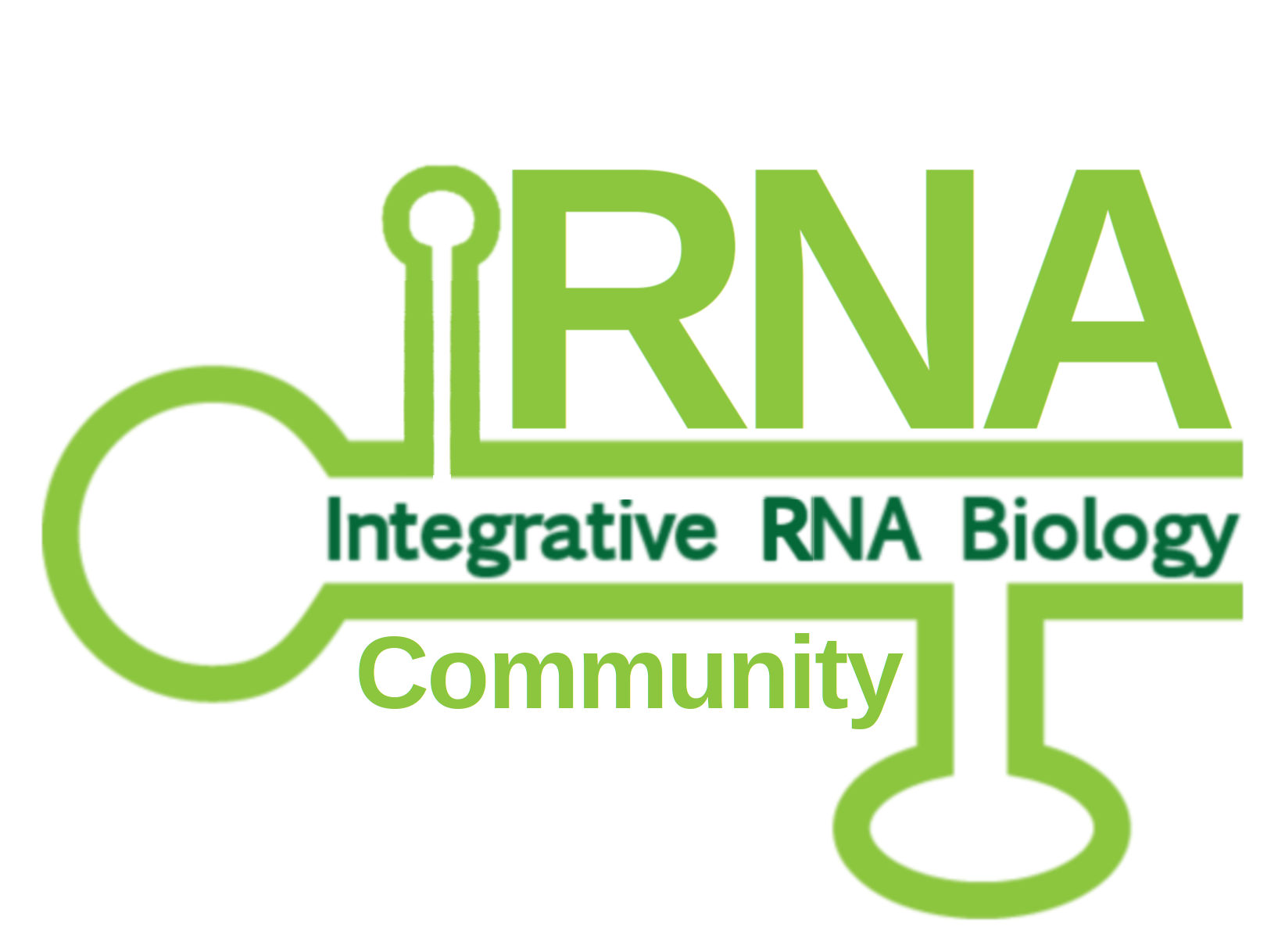
iRNA
Accurate Assembly of Multiple RNA-seq Samples with Aletsch
- Qian Shi, Department of Computer Science and Engineering, The Pennsylvania State University, United States
- Qimin Zhang, Department of Computer Science and Engineering, The Pennsylvania State University, United States
- Mingfu Shao, Department of Computer Science and Engineering, The Pennsylvania State University, United States
Presentation Overview: Show
High-throughput RNA sequencing has become indispensable for decoding gene activities, yet the challenge of reconstructing full-length transcripts persists. Traditional single-sample assemblers frequently produce fragmented transcripts, especially in single-cell RNA-seq data. While algorithms designed for assembling multiple samples exist, they encounter various limitations. We present Aletsch, a new assembler for multiple bulk or single-cell RNA-seq samples. Aletsch incorporates several algorithmic innovations, including a “bridging” system that can effectively integrate multiple samples to restore missed junctions in individual samples, and a new graph-decomposition algorithm that leverages “supporting information across multiple samples to guide the decomposition of complex vertices. A standout feature of Aletsch is its application of a random forest model with 50 well-designed features for scoring transcripts. We demonstrate its robust adaptability across different chromosomes, datasets, and species. Our experiments, conducted on RNA-seq data from several protocols, firmly demonstrate Aletsch’s significant outperformance over existing meta-assemblers. As an example, when measured with the partial area under the precision-recall curve (pAUC) , Aletsch surpasses the leading assemblers TransMeta by 21.2%-57.4% and PsiCLASS by 21.9%-172.5% on human datasets. Aletsch is freely available at https://github.com/Shao-Group/aletsch.
Partial RNA Design
- Frederic Runge, University of Freiburg, Germany
- Jörg K.H. Franke, University of Freiburg, Germany
- Daniel Fertmann, University of Freiburg, Germany
- Rolf Backofen, University of Freiburg, Germany
- Frank Hutter, University of Freiburg, Germany
Presentation Overview: Show
RNA design is a key technique to achieve new functionality in fields like synthetic biology or biotechnology. Computational tools could help to find such RNA sequences but they are often limited in their formulation of the search space. In this work, we propose partial RNA design, a novel RNA design paradigm that addresses the limitations of current RNA design formulations. Partial RNA design describes the problem of designing RNAs from arbitrary RNA sequences and structure motifs with different design goals. By separating the design space from the objectives, our formulation enables the design of RNAs with variable lengths and desired properties, while still allowing precise control over sequence and structure constraints at individual positions. Based on this formulation, we introduce a new algorithm, libLEARNA, capable of efficiently solving different constraint RNA design tasks. A comprehensive analysis of various problems, including a realistic riboswitch design task, reveals the outstanding performance of libLEARNA and its robustness.
MICROBIOME
Floria: Fast and accurate strain haplotyping in metagenomes
- Jim Shaw, University of Toronto, Canada
- Jean-Sebastien Gounot, Genome Institute of Singapore, Singapore
- Hanrong Chen, Genome Institute of Singapore, Singapore
- Niranjan Nagarajan, Genome Institute of Singapore, Singapore
- Yun William Yu, Carnegie Mellon University, United States
Presentation Overview: Show
Shotgun metagenomics allows for direct analysis of microbial community genetics, but scalable computational methods for the recovery of bacterial strain genomes from microbiomes remains a key challenge. We introduce Floria, a novel method designed for rapid and accurate recovery of strain haplotypes from short and long-read metagenome sequencing data, based on minimum error correction (MEC) read clustering and a strain-preserving network flow model. Floria can function as a standalone haplotyping method, outputting alleles and reads that co-occur on the same strain, as well as an end-to-end read-to-assembly pipeline (Floria-PL) for strain-level assembly. Benchmarking evaluations on synthetic metagenomes showed that Floria is > 3x faster and recovers 21% more strain content than base-level assembly methods (Strainberry), while being over an order of magnitude faster when only phasing is required. Applying Floria to a set of 109 deeply sequenced nanopore metagenomes took < 20 minutes on average per sample, and identified several species that have consistent strain heterogeneity. Applying Floria’s short-read haplotyping to a longitudinal gut metagenomics dataset revealed a dynamic multi-strain Anaerostipes hadrus community with frequent strain loss and emergence events over 636 days. With Floria, accurate haplotyping of metagenomic datasets takes mere minutes on standard workstations, paving the way for extensive strain-level metagenomic analyses.
Reference-free Structural Variant Detection in Microbiomes via Long-read Co-assembly Graphs
- Kristen Curry, Rice University, United States
- Feiqiao Yu, Arc Institute, United States
- Summer Vance, University of California, Berkeley, United States
- Santiago Segarra, Rice University, United States
- Devaki Bhaya, Carnegie Institute for Science, United States
- Rayan Chikhi, Institut Pasteur, Université Paris Cité, France
- Eduardo Rocha, Institut Pasteur, Université Paris Cité, France
- Todd Treangen, Rice University, United States
Presentation Overview: Show
Bacterial genome dynamics are vital for understanding the mechanisms underlying microbial adaptation, growth, and their broader impact on host phenotype. Structural variants (SVs), genomic alterations of 50 base pairs or more, play a pivotal role in driving evolutionary processes and maintaining genomic heterogeneity within bacterial populations. While SV detection in isolate genomes is relatively straightforward, metagenomes present broader challenges due to the absence of clear reference genomes and the presence of mixed strains. In response, our proposed method rhea, forgoes reference genomes and metagenome-assembled genomes (MAGs) by encompassing a single metagenome co-assembly graph constructed from all samples in a series. The log fold change in graph coverage between subsequent samples is then calculated to call SVs that are thriving or declining throughout the series. We show rhea to outperform existing methods for SV and horizontal gene transfer (HGT) detection in two simulated mock metagenomes, which is particularly noticeable as the simulated reads diverge from reference genomes and an increase in strain diversity is incorporated. We additionally demonstrate use cases for rhea on series metagenomic data of environmental and fermented food microbiomes to detect specific sequence alterations between successive time and temperature samples, suggesting host advantage. Our approach leverages previous work in assembly graph structural and coverage patterns to provide versatility in studying SVs across diverse and poorly characterized microbial communities for more comprehensive insights into microbial gene flux.
Scalable de novo Classification of Antimicrobial Resistance of Mycobacterium Tuberculosis
- Mohammadali Serajian, Unievrsity of Florida, United States
- Simone Marini, University of Florida, United States
- Jarno N. Alanko, University of Helsinki, Finland
- Noelle R. Noyes, University of Minnesota, United States
- Mattia Prosperi, University of Florida, United States
- Christina Boucher, University of Florida, United States
Presentation Overview: Show
We develop a robust machine learning classifier using both linear and nonlinear models (i.e., LASSO logistic regression (LR) and random forests (RF)) to predict the phenotypic resistance of \emph{Mycobacterium tuberculosis} (MTB) for a broad range of antibiotic drugs. We use data from the CRyPTIC consortium to train our classifier, which consists of whole genome sequencing and antibiotic susceptibility testing (AST) phenotypic data for 13 different antibiotics. To train our model, we assemble the sequence data into genomic contigs, identify all unique 31-mers in the set of contigs, and build a feature matrix M, where M[i,j] is equal to the number of times the i-th 31-mer occurs in the j-th genome. Due to the size of this feature matrix (over 350 million unique 31-mers), we build and use a sparse matrix representation. Our method, which we refer to as MTB++, leverages compact data structures and iterative methods to allow for the screening of all the 31-mers in the development of both LASSO LR and RF. MTB++ is able to achieve high discrimination (F-1 greater than 80%) for the first-line antibiotics. Moreover, MTB++ had the highest F-1 score in all but three classes and was the most comprehensive since it had an F-1 score greater than 75% in all but four (rare) antibiotic drugs. We use our feature selection to contextualize the 31-mers that are used for the prediction of phenotypic resistance, leading to some insights about sequence similarity to genes in MEGARes.
Towards more accurate microbial source tracking via non-negative matrix factorization (NMF)
- Ziyi Huang, City University of Hong Kong, Hong Kong
- Dehan Cai, City University of Hong Kong, Hong Kong
- Yanni Sun, City University of Hong Kong, Hong Kong
Presentation Overview: Show
Motivation: The microbiome of a sampled habitat often consists of microbial communities from various sources, including potential contaminants. Microbial source tracking (MST) can be used to discern the contribution of each source to the observed microbiome data, thus enabling the identification and tracking of microbial communities within a sample. Therefore, MST has various applications, from monitoring microbial contamination in clinical labs to tracing the source of pollution in environmental samples. Despite promising results in MST development, there is still room for improvement, particularly for applications where precise quantification of each source’s contribution is critical.
Results: In this study, we introduce a novel tool called SourceID-NMF towards more precise microbial source tracking. SourceID-NMF utilizes a non-negative matrix factorization (NMF) algorithm to trace the microbial sources contributing to a target sample, without assuming specific probability distributions. By leveraging the taxa abundance in both available sources and the target sample, SourceID-NMF estimates the proportion of available sources present in the target sample. To evaluate the performance of SourceID-NMF, we conducted a series of benchmarking experiments using simulated and real data. The simulated experiments mimic realistic yet challenging scenarios for identifying highly similar sources, irrelevant sources, unknown sources, low abundance sources, and noise sources. The results demonstrate the superior accuracy of SourceID-NMF over existing methods. Particularly, SourceID-NMF accurately estimated the proportion of irrelevant and unknown sources while other tools either over- or under-estimated them. Additionally, the noise sources experiment also demonstrated the robustness of SourceID-NMF for MST.
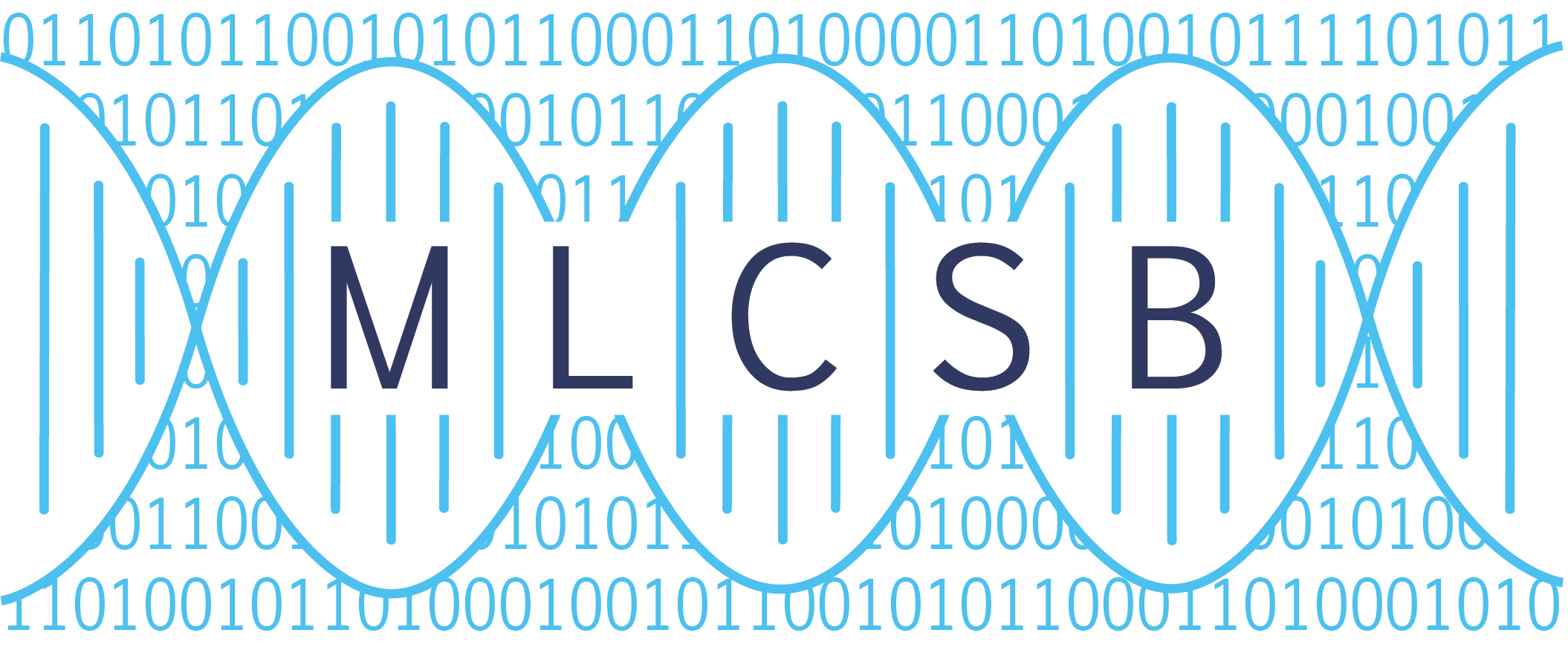
MLCSB
AttentionPert: Accurately Modeling Multiplexed Genetic Perturbations with Multi-scale Effects
- Ding Bai, Mohamed bin Zayed University of Artificial Intelligence (MBZUAI), United Arab Emirates
- Caleb Ellington, Carnegie Mellon University, United States
- Shentong Mo, Mohamed bin Zayed University of Artificial Intelligence (MBZUAI), United Arab Emirates
- Le Song, Mohamed bin Zayed University of Artificial Intelligence (MBZUAI), United Arab Emirates
- Eric Xing, Mohamed bin Zayed University of Artificial Intelligence (MBZUAI) & Carnegie Mellon University, United Arab Emirates
Presentation Overview: Show
Genetic perturbations (e.g. knockouts, variants) have laid the foundation for our understanding of many diseases, implicating pathogenic mechanisms and indicating therapeutic targets. However, experimental assays are fundamentally limited by the number of measurable perturbations. Computational methods can fill this gap by predicting perturbation effects under novel conditions, but accurately predicting the transcriptional responses of cells to unseen perturbations remains a significant challenge. We address this by developing a novel attention-based neural network, AttentionPert, which accurately predicts gene expression under multiplexed perturbations and generalizes to unseen conditions. AttentionPert integrates global and local effects in a multi-scale model, representing both the non-uniform system-wide impact of the genetic perturbation and the localized disturbance in a network of gene-gene similarities, enhancing its ability to predict nuanced transcriptional responses to both single and multi-gene perturbations. In comprehensive experiments, AttentionPert demonstrates superior performance across multiple datasets outperforming the state-of-the-art method in predicting differential gene expressions and revealing novel gene regulations. AttentionPert marks a significant improvement over current methods, particularly in handling the diversity of gene perturbations and in predicting out-of-distribution scenarios.
CODEX: COunterfactual Deep learning for the in-silico EXploration of cancer cell line perturbations
- Stefan Schrod, University Medical Center Göttingen, Germany
- Helena Zacharias, Hannover Medical School, Germany
- Tim Beissbarth, University Medical Center Göttingen, Germany
- Anne-Christin Hauschild, University Medical Center G ̈ottingen, Germany
- Michael Altenbuchinger, University Medical Center Göttingen, Germany
Presentation Overview: Show
Motivation: High-throughput screens (HTS) provide a powerful tool to decipher the causal effects of chemical and genetic perturbations on cancer cell lines. Their ability to evaluate a wide spectrum of interventions, from single drugs to intricate drug combinations and CRISPR-interference, has established them as an invaluable resource for the development of novel therapeutic approaches. Nevertheless, the combinatorial complexity of potential interventions makes a comprehensive exploration intractable. Hence, prioritizing interventions for further experimental investigation becomes of utmost importance.
Results: We propose CODEX as a general framework for the causal modeling of HTS data, linking perturbations to their downstream consequences. CODEX relies on a stringent causal modeling strategy based on counterfactual reasoning. As such, CODEX predicts drug-specific cellular responses, comprising cell survival and molecular alterations, and facilitates the in-silico exploration of drug combinations. This is achieved for both bulk and single-cell HTS. We further show that CODEX provides a rationale to explore complex genetic modifications from CRISPR-interference in silico in single cells.
Availability and Implementation: Our implementation of CODEX is publicly available at https://github.com/sschrod/CODEX. All data used in this article are publicly available.
Supplementary information: Supplementary materials are available at Bioinformatics online.
Deciphering High-order Structures in Spatial Transcriptomes with Graph-guided Tucker Decomposition
- Charles Broadbent, University of Minnesota Twin Cities, United States
- Tianci Song, University of Minnesota Twin Cities, United States
- Rui Kuang, University of Minnesota Twin Cities, United States
Presentation Overview: Show
Spatial transcripome (ST) profiling can reveal cells’ structural organizations and functional roles in tissues. However, deciphering the spatial context of gene expressions in ST data is a challenge—the high-order structure hiding in whole transcriptome space over 2D/3D spatial coordinates requires modeling and detection of interpretable high-order elements and components for further functional analysis and interpretation. This paper presents a new method GraphTucker—-graph-regularized Tucker tensor decomposition for learning high-order factorization in ST data. GraphTucker is based on a non-negative Tucker decomposition algorithm regularized by a high-order graph that captures spatial relation among spots and functional relation among genes. In the experiments on several Visium and Stereo-seq datasets, the novelty and advantage of modeling multi-way multilinear relationships among the components in Tucker decomposition are demonstrated as opposed to the Canonical Polyadic Decomposition (CPD) and conventional matrix factorization models by evaluation of detecting spatial components of gene modules, clustering spatial coefficients for tissue segmentation and imputing complete spatial transcriptomes. The results of visualization show strong evidences that GraphTucker detect more interpretable spatial components in the context of the spatial domains in the tissues. Availability: https://github.com/kuanglab/GraphTucker.
Integrating patients in time series clinical transcriptomics data
- Euxhen Hasanaj, Carnegie Mellon University, United States
- Sachin Mathur, Sanofi, United States
- Ziv Bar-Joseph, Carnegie Mellon University, United States
Presentation Overview: Show
Motivation: Analysis of time series transcriptomics data from clinical trials is challenging. Such studies usually profile very few time points from several individuals with varying response patterns and dynamics. Current methods for these datasets are mainly based on linear, global orderings using visit times which do not account for the varying response rates and subgroups within a patient cohort.
Results We developed a new method that utilizes multi-commodity flow algorithms for trajectory inference in large scale clinical studies. Recovered trajectories satisfy individual-based timing restrictions while integrating data from multiple patients. Testing the method on multiple drug datasets demonstrated an improved performance compared to prior approaches suggested for this task, while identifying novel endotypes that correspond to heterogeneous patient response patterns.
Availability: The source code and instructions to download the data have been deposited on GitHub at https://github.com/euxhenh/Truffle
MolPLA: A Molecular Pre-training Framework for Learning Cores, R-Groups and their Linker Joints
- Mogan Gim, Korea University, South Korea
- Jueon Park, Korea University, South Korea
- Soyon Park, Korea University, South Korea
- Sanghoon Lee, Korea University, South Korea
- Seungheun Baek, Korea University, South Korea
- Junhyun Lee, Korea University, South Korea
- Ngoc-Quang Nguyen, Korea University, South Korea
- Jaewoo Kang, Korea University, South Korea
Presentation Overview: Show
Motivation: Molecular core structures and R-groups are essential concepts especially in compound analysis and lead optimization. Integration of these concepts with conventional graph pre-training approaches can promote deeper understanding in both local and global properties of molecules. We propose MolPLA, a dual molecular pre-training framework that promotes understanding in a molecule's core structure with peripheral R-groups and extends it with the ability to help chemists find replaceable R-groups in lead optimization scenarios.
Results: Experimental results on molecular property prediction show that MolPLA exhibits predictability comparable to current state-of-the-art models. Qualitative analysis implicate that MolPLA is capable of distinguishing core and R-group sub-structures, identifying decomposable regions in molecules and contributing to lead optimization scenarios by rationally suggesting R-group replacements given various query core templates.
oncotree2vec – A method for embedding and clustering of tumor mutation trees
- Monica-Andreea Baciu-Dragan, ETHZ, Switzerland
- Niko Beerenwinkel, ETHZ, Switzerland
Presentation Overview: Show
Understanding the genomic heterogeneity of tumors is an important task in computational oncology, especially in the context of finding personalized treatments based on the genetic profile of each patient’s tumor. Tumor clustering that takes into account the temporal order of genetic events, as represented by tumor mutation trees, is a powerful approach for grouping together patients with genetically and evolutionarily similar tumors and can provide insights into discovering tumor subtypes, for more accurate clinical diagnosis and prognosis. Here, we propose oncotree2vec, a method for clustering tumor mutation trees by learning vector representations of mutation trees that capture the different relationships between subclones in an unsupervised manner. Learning low-dimensional tree embeddings facilitates the visualization of relations between trees in large cohorts and can be used for downstream analyses, such as deep learning approaches for single-cell multi-omics data integration. We assessed the performance and the usefulness of our method in three simulation studies, and on two real datasets: a cohort of 43 trees from six cancer types with different branching patterns corresponding to different modes of spatial tumor evolution and a cohort of 123 AML mutation trees.
Predicting single-cell cellular responses to perturbations using cycle consistency learning
- Wei Huang, College of Computer and Information Engineering, Nanjing Tech University, 211816, Jiangsu, China, China
- Hui Liu, College of Computer and Information Engineering, Nanjing Tech University, 211816, Jiangsu, China, China
Presentation Overview: Show
Phenotype-based screening has emerged as a powerful approach for identifying compounds that actively interact with cells. Transcriptional and proteomic profiling of cell population and single cell provide insights into the cellular changes that occur at the molecular level in response to external perturbations, such as drugs or genetic manipulations. In this paper, we propose cycleCDR, a novel deep learning framework to predict cellular response to drugs or gene perturbations. We leverage the power of autoencoders to maps the unperturbed cellular states to a latent space, in which we postulate the effects of drug perturbations on cellular states follow a linear additive model. Next, we introduce the cycle consistency constraints to ensure that unperturbed cellular state subjected to drug perturbation in the latent space would produce the perturbed cellular state through the decoder. Conversely, removal of perturbations from the perturbed cellular states could restore the unperturbed cellular state. The cycle consistency constraints and linear modeling in latent space enable to learn transferable representations of external perturbations, so that our model can generalize well to unseen drugs. We validate our model on four different types of datasets, including bulk transcriptional responses, bulk proteomic responses, and single-cell transcriptional responses to drug/gene perturbations. The experimental results demonstrate that our model consistently outperforms existing state-of-the-art methods, indicating our method is highly versatile and applicable to a wide range of scenarios.
Probabilistic Pathway-based Multimodal Factor Analysis
- Alexander Immer, Biomedical Informatics Group, Department of Computer Science, ETH Zurich, Zurich, Switzerland
- Stefan G. Stark, Biomedical Informatics Group, Department of Computer Science, ETH Zurich, Zurich, Switzerland, Switzerland
- Francis Jacob, Ovarian Cancer Research, Department of Biomedicine, University Hospital Basel and University of Basel, Switzerland, Switzerland
- Ximena Bonilla, Biomedical Informatics Group, Department of Computer Science, ETH Zurich, Zurich, Switzerland, Switzerland
- Tinu Thomas, Biomedical Informatics Group, Department of Computer Science, ETH Zurich, Zurich, Switzerland, Switzerland
- Andre Kahles, Biomedical Informatics Group, Department of Computer Science, ETH Zurich, Zurich, Switzerland, Switzerland
- Sandra Goetze, Institute of Translational Medicine, Dep. of Health Sciences and Technology, ETH Zurich, Zurich, Switzerland, Switzerland
- Emanuela S. Milani, Institute of Translational Medicine, Dep. of Health Sciences and Technology, ETH Zurich, Zurich, Switzerland, Switzerland
- Bernd Wollscheid, Institute of Translational Medicine, Dep. of Health Sciences and Technology, ETH Zurich, Zurich, Switzerland, Switzerland
- Gunnar Rätsch, Biomedical Informatics Group, Department of Computer Science, ETH Zurich, Zurich, Switzerland, Switzerland
- Kjong-Van Lehmann, Cancer Research Center Cologne-Essen, Uniklinik Koeln, Germany
Presentation Overview: Show
Multimodal profiling strategies promise to produce more informative insights into biomedical cohorts via the integration of the information each modality contributes. In order to perform this integration, however, the development of novel analytical strategies are needed. Multimodal profiling strategies often come at the expense of lower sample numbers, which can challenge methods to uncover shared signals across a cohort. Thus, factor analysis approaches are commonly used for the analysis of high-dimensional data in molecular biology, however they typically do not yield representations that are directly interpretable, whereas many research questions often center around the analysis of pathways associated with specific observations.
We develop PathFA, a novel approach for multimodal factor analysis over the space of pathways. PathFA produces integrative and interpretable views across multimodal profiling technologies, which allow for the derivation of concrete hypotheses. PathFA combines a pathway-learning approach with integrative multimodal capability under a Bayesian procedure that is efficient, hyper-parameter free, and able to automatically infer observation noise from the data. We demonstrate strong performance on small sample sizes within our simulation framework and on matched proteomics and transcriptomics profiles from real tumor samples taken from the Swiss Tumor Profiler consortium. On a subcohort of melanoma patients, PathFA recovers pathway activity that has been independently associated with poor outcome. We further demonstrate the ability of this approach to identify pathways associated with the presence of specific cell-types as well as tumor heterogeneity. Our results show that we capture known biology, making it well suited for analyzing multimodal sample cohorts.
SPRITE: improving spatial gene expression imputation with gene and cell networks
- Eric Sun, Stanford University, United States
- Rong Ma, Harvard T.H. Chan School of Public Health, United States
- James Zou, Stanford University, United States
Presentation Overview: Show
Spatially resolved single-cell transcriptomics have provided unprecedented insights into gene expression {\it in situ}, particularly in the context of cell interactions or organization of tissues. However, current technologies for profiling spatial gene expression at single-cell resolution are generally limited to the measurement of a small number of genes. To address this limitation, several algorithms have been developed to impute or predict the expression of additional genes that were not present in the measured gene panel. Current algorithms do not leverage the rich spatial and gene relational information in spatial transcriptomics. To improve spatial gene expression predictions, we introduce SPRITE (Spatial Propagation and Reinforcement of Imputed Transcript Expression) as a meta-algorithm that processes predictions obtained from existing methods by propagating information across gene correlation networks and spatial neighborhood graphs. SPRITE improves spatial gene expression predictions across multiple spatial transcriptomics datasets. Furthermore, SPRITE predicted spatial gene expression leads to improved clustering, visualization, and classification of cells. SPRITE is available as a software package and can be used in spatial transcriptomics data analysis to improve inferences based on predicted gene expression.
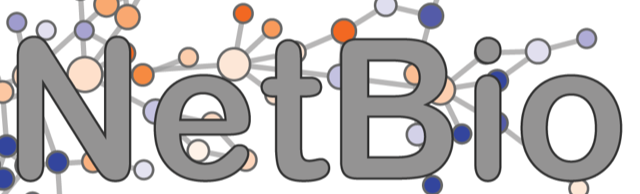
NetBio
GraphCompass: Spatial metrics for differential analyses of cell organization across conditions
- Mayar Ali, Helmholtz Munich, Germany
- Merel Kuijs, Helmholtz Munich, Germany
- Soroor Hediyeh-zadeh, Helmholtz Munich, Germany
- Tim Treis, Helmholtz Munich, Germany
- Karin Hrovatin, Helmholtz Munich, Germany
- Giovanni Palla, Helmholtz Munich, Germany
- Anna Schaar, Helmholtz Munich, Germany
- Fabian Theis, Helmholtz Munich, Germany
Presentation Overview: Show
Spatial omics technologies are increasingly leveraged to characterize how disease disrupts tissue organization and cellular niches. While multiple methods to analyze spatial variation within a sample have been published, statistical and computational approaches to compare cell spatial organization across samples or conditions are mostly lacking. We present GraphCompass, a comprehensive set of omics-adapted graph analysis methods to quantitatively evaluate and compare the spatial arrangement of cells in samples representing diverse biological conditions. GraphCompass builds upon the Squidpy spatial omics toolbox and encompasses various statistical approaches to perform cross-condition analyses at the level of individual cell types, niches, and samples. Additionally, GraphCompass provides custom visualization functions that enable effective communication of results. We demonstrate how GraphCompass can be used to address key biological questions, such as how cellular organization and tissue architecture differ across various disease states and which spatial patterns correlate with a given pathological condition. GraphCompass can be applied to various popular omics techniques, including, but not limited to, spatial proteomics (e.g. MIBI-TOF), spot-based transcriptomics (e.g. 10x Genomics Visium), and single-cell resolved transcriptomics (e.g. Stereo-seq). In this work, we showcase the capabilities of GraphCompass through its application to three different studies that may also serve as benchmark datasets for further method development. With its easy-to-use implementation, extensive documentation, and comprehensive tutorials, GraphCompass is accessible to biologists with varying levels of computational expertise. By facilitating comparative analyses of cell spatial organization, GraphCompass promises to be a valuable asset in advancing our understanding of tissue function in health and disease.
Identifying new cancer genes based on the integration of annotated gene sets via hypergraph neural networks
- Chao Deng, Central South University, China
- Hongdong Li, Central South University, China
- Lishen Zhang, Central South University, China
- Yiwei Liu, Central South University, China
- Yaohang Li, Old Dominion University, United States
- Jianxin Wang, Central South University, China
Presentation Overview: Show
Motivation: Identifying cancer genes remains a significant challenge in cancer genomics research. Annotated gene sets encode functional associations among multiple genes, and cancer genes have been shown to cluster in hallmark signaling pathways and biological processes. The knowledge of annotated gene sets is critical for discovering cancer genes but remains to be fully exploited.
Results: Here, we present the DIsease-Specific Hypergraph neural network (DISHyper), a hypergraph-based computational method that integrates the knowledge from multiple types of annotated gene sets to predict cancer genes. First, our benchmark results demonstrate that DISHyper outperforms the existing state-of-the-art methods and highlight the advantages of employing hypergraphs for representing annotated gene sets. Second, we validate the accuracy of DISHyper-predicted cancer genes using functional validation results and multiple independent functional genomics data. Third, our model predicts 44 novel cancer genes, and subsequent analysis shows their significant associations with multiple types of cancers. Overall, our study provides a new perspective for discovering cancer genes and reveals previously undiscovered cancer genes.
Availability: DISHyper is freely available for download at https://github.com/genemine/DISHyper.
Modeling metastatic progression from cross-sectional cancer genomics data
- Kevin Rupp, ETH Zurich, Switzerland
- Andreas Lösch, University of Regensburg, Germany
- Y. Linda Hu, University of Regensburg, Germany
- Chenxi Nie, ETH Zurich, Switzerland
- Rudolf Schill, ETH Zurich, Switzerland
- Maren Klever, RWTH Aachen, Germany
- Simon Pfahler, University of Regensburg, Germany
- Lars Grasedyck, RWTH Aachen, Germany
- Tilo Wettig, University of Regensburg, Germany
- Niko Beerenwinkel, ETH Zurich, Switzerland
- Rainer Spang, University of Regensburg, Germany
Presentation Overview: Show
Metastasis formation is a hallmark of cancer lethality. Yet, metastases are generally unobservable during their early stages
of dissemination and spread to distant organs. Genomic datasets of matched primary tumors and metastases may offer
insights into the underpinnings and the dynamics of metastasis formation. We present metMHN, a cancer progression
model designed to deduce the joint progression of primary tumors and metastases using cross-sectional cancer genomics
data. The model elucidates the statistical dependencies among genomic events, the formation of metastasis, and the clinical
emergence of both primary tumors and their metastatic counterparts. metMHN enables the chronological reconstruction
of mutational sequences and facilitates estimation of the timing of metastatic seeding. In a study of nearly 5000 lung
adenocarcinomas, metMHN pinpointed TP53 and EGFR as mediators of metastasis formation. Furthermore, the study
revealed that post-seeding adaptation is predominantly influenced by frequent copy number alterations. All datasets and
code are available on GitHub at https://github.com/cbg-ethz/metMHN.
Transfer Learning of Condition-Specific Perturbation in Gene Interactions Improves Drug Response Prediction
- Dongmin Bang, Seoul National University, South Korea
- Bonil Koo, Seoul National University, South Korea
- Sun Kim, Seoul National University, South Korea
Presentation Overview: Show
Drug response is conventionally measured at the cell level, often quantified by metrics like IC50. However, to gain a deeper understanding of drug response, cellular outcomes need to be understood in terms of pathway perturbation. This perspective leads us to recognize a challenge posed by the gap between two widely used large-scale databases, LINCS L1000 and GDSC, measuring drug response at different levels – L1000 captures information at the gene expression level, while GDSC operates at the cell line level. Our study aims to bridge this gap by integrating the two databases through transfer learning, focusing on condition-specific perturbations in gene interactions from L1000 to interpret drug response integrating both gene and cell levels in GDSC. This transfer learning strategy involves pretraining on the transcriptomic-level L1000 dataset, with parameter-frozen fine-tuning to cell line-level drug response. Our novel Condition-Specific Gene-Gene Attention (CSG2A) mechanism dynamically learns gene interactions specific to input conditions, guided by both data and biological network priors. The CSG2A network, equipped with transfer learning strategy, achieves state-of-the-art performance in cell line-level drug response prediction. In two case studies, well-known mechanisms of drugs are well represented in both the learned gene-gene attention and the predicted transcriptomic profiles. This alignment supports the modeling power in terms of interpretability and biological relevance. Furthermore, our model's unique capacity to capture drug response in terms of both pathway perturbation and cell viability extends predictions to the patient level using TCGA data, demonstrating its expressive power obtained from both gene and cell levels.
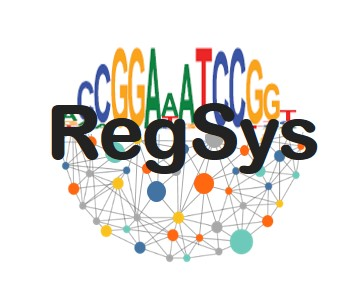
RegSys
A count-based model for delineating cell-cell interactions in spatial transcriptomics data
- Hirak Sarkar, Princeton University, United States
- Uthsav Chitra, Princeton University, United States
- Julian Gold, Princeton University, United States
- Benjamin Raphael, Princeton University, United States
Presentation Overview: Show
Motivation: Cell-cell interactions (CCIs) consist of cells exchanging signals with themselves and neighboring cells by expressing ligand and receptor molecules, and play a key role in cellular development, tissue homeostasis, and other critical biological functions. Since direct measurement of CCIs is challenging, multiple methods have been developed to infer CCIs by quantifying correlations between the gene expression of the ligands and receptors that mediate CCIs, originally from bulk RNA sequencing data and more recently from single-cell or spatial transcriptomics data. Spatial transcriptomics has a particular advantage over single-cell approaches since ligand-receptor correlations can be computed between cells or spots that are physically close in the tissue. However, the transcript counts of individual ligands and receptors in spatial transcriptomics data are generally low, complicating the inference of CCIs from expression correlations.
Results: We introduce Copulacci, a count-based model for inferring CCIs from spatial transcriptomics data. Copulacci uses a Gaussian copula to model dependencies between the expression of ligands and receptors from nearby spatial locations even when the transcript counts are low. On simulated data, Copulacci outperforms existing CCI inference methods based on the standard Spearman and Pearson correlation coefficients. Using several real spatial transcriptomics datasets, we show that Copulacci discovers biologically meaningful ligand-receptor interactions that are lowly expressed and undiscoverable by existing CCI inference methods.
Availability: Copulacci is implemented in Python and available at https://github.com/raphael-group/copulacci
Enhancing Hi-C contact matrices for loop detection with Capricorn, a multi-view diffusion model
- Tangqi Fang, University of Washington, United States
- Yifeng Liu, University of Washington, United States
- Addie Woicik, University of Washington, United States
- Minsi Lu, University of Washington, United States
- Anupama Jha, University of Washington, United States
- Xiao Wang, Purdue University, United States
- Gang Li, University of Washington, United States
- Borislav Hristov, University of Washington, United States
- Zixuan Liu, University of Washington, United States
- Hanwen Xu, University of Washington, United States
- William Noble, University of Washington, United States
- Sheng Wang, University of Washington, United States
Presentation Overview: Show
Motivation: High-resolution Hi-C contact matrices reveal the detailed three-dimensional architecture of the genome, but high-coverage experimental Hi-C data are expensive to generate. On the other hand, chromatin structure analyses struggle with extremely sparse contact matrices. To address this problem, computational methods to enhance low-coverage contact matrices have been developed, but existing methods are largely based on resolution enhancement methods for natural images and hence often employ models that do not distinguish between biologically meaningful contacts, such as loops, and other stochastic contacts.
Results: We present Capricorn, a machine learning model for Hi-C resolution enhancement that incorporates small-scale chromatin features as additional views of the input Hi-C contact matrix and leverages a diffusion probability model backbone to generate a high-coverage matrix. We show that Capricorn outperforms the state of the art in a cross-cell-line setting, improving on existing methods by 17% in mean squared error and 26% in F1 score for chromatin loop identification from the generated high-coverage data. We demonstrate that Capricorn performs well in the cross-chromosome setting and cross-chromosome, cross-cell-line setting. We further show that our multi-view idea can also be used to improve several existing methods, HiCARN and HiCNN, indicating the wide applicability of this approach. Finally, we use DNA sequence to validate discovered loops and find that the fraction of CTCF-supported loops from Capricorn is similar to those identified from the high-coverage data. Capricorn is a powerful Hi-C resolution enhancement method that enables scientists to find chromatin features that cannot be identified in the low-coverage contact matrix.
Optimal sequencing budget allocation for trajectory reconstruction of single cells
- Noa Moriel, Hebrew University of Jerusalem, Israel
- Edvin Memet, Harvard University, United States
- Mor Nitzan, Hebrew University of Jerusalem, Israel
Presentation Overview: Show
Charting cellular trajectories over gene expression is key to understanding dynamic cellular processes and their underlying mechanisms. While advances in single-cell RNA-sequencing technologies and computational methods have pushed forward the recovery of such trajectories, trajectory inference remains a challenge due to the noisy, sparse, and high-dimensional nature of single-cell data. This challenge can be alleviated by increasing either the number of cells sampled along the trajectory (breadth) or the sequencing depth, i.e. the number of reads captured per cell (depth). Generally, these two factors are coupled due to an inherent breadth-depth tradeoff that arises when the sequencing budget is constrained due to financial or technical limitations. Here we study the optimal allocation of a fixed sequencing budget to optimize the recovery of trajectory attributes. Empirical results reveal that reconstruction accuracy of internal cell structure in expression space scales with the logarithm of either the breadth or depth of sequencing. We additionally observe a power law relationship between the optimal number of sampled cells and the corresponding sequencing budget. For linear trajectories, non-monotonicity in trajectory reconstruction across the breadth-depth tradeoff can impact downstream inference, such as expression pattern analysis along the trajectory. We demonstrate these results for five single-cell RNA-sequencing datasets encompassing differentiation of embryonic stem cells, pancreatic β cells, hepatoblast and multipotent haematopoietic cells, as well as induced reprogramming of embryonic fibroblasts into neurons. By addressing the challenges of single-cell data, our study offers insights into maximizing the efficiency of cellular trajectory analysis through strategic allocation of sequencing resources.
REUNION: transcription factor binding prediction and regulatory association inference from single-cell multi-omics data
- Yang Yang, Memorial Sloan Kettering Cancer Center, Howard Hughes Medical Institute, United States
- Dana Pe'er, Memorial Sloan Kettering Cancer Center, Howard Hughes Medical Institute, United States
Presentation Overview: Show
Motivation: Profiling of gene expression and chromatin accessibility by single-cell multi-omics approaches can help to systematically decipher how transcription factors (TFs) regulate target gene expression via cis-region interactions. However, integrating information from different modalities to discover regulatory associations is challenging, in part because motif scanning approaches miss many likely TF binding sites.
Results: We develop REUNION, a framework for predicting genome-wide TF binding and cis-region-TF-gene “triplet” regulatory associations using single-cell multi-omics data. The first component of REUNION, Unify, utilizes information theory-inspired complementary score functions that incorporate TF expression, chromatin accessibility, and target gene expression to identify regulatory associations. The second component, Rediscover, takes Unify estimates as input for pseudo semi-supervised learning to predict TF binding in accessible genomic regions that may or may not include detected TF motifs. Rediscover leverages latent chromatin accessibility and sequence feature spaces of the genomic regions, without requiring chromatin immunoprecipitation data for model training. Applied to peripheral blood mononuclear cell data, REUNION outperforms alternative methods in TF binding prediction on average performance. In particular, it recovers missing region-TF associations from regions lacking detected motifs, which circumvents the reliance on motif scanning and facilitates discovery of novel associations involving potential co-binding transcriptional regulators. Newly identified region-TF associations, even in regions lacking a detected motif, improve the prediction of target gene expression in regulatory triplets, and are thus likely to genuinely participate in the regulation.
Availability and implementation: All source code is available at https://github.com/yangymargaret/REUNION.
scGrapHiC: Deep learning-based graph deconvolution for Hi-C using single cell gene expression
- Ghulam Murtaza, Department of Computer Science, Brown University, United States
- Byron Butaney, Department of Computer Science, Brown University, United States
- Justin Wagner, Material Measurement Laboratory, National Institute of Standards and Technology, United States
- Ritambhara Singh, Department of Computer Science and Center for Computational Molecular Biology, Brown University, United States
Presentation Overview: Show
Single-cell Hi-C (scHi-C) protocol helps identify cell-type-specific chromatin interactions and sheds light on cell differentiation and disease progression. Despite providing crucial insights, scHi-C data is often underutilized due to the high cost and the complexity of the experimental protocol. We present a deep learning framework, scGrapHiC, that predicts pseudo-bulk scHi-C contact maps using pseudo-bulk scRNA-seq data. Specifically, scGrapHiC performs graph deconvolution to extract genome-wide single-cell interactions from a bulk Hi-C contact map using scRNA-seq as a guiding signal. Our evaluations show that scGrapHiC, trained on 7 cell-type co-assay datasets, outperforms typical sequence encoder approaches. For example, scGrapHiC achieves a substantial improvement of 23.2% in recovering cell-type-specific Topologically Associating Domains over the baselines. It also generalizes to unseen embryo and brain tissue samples. scGrapHiC is a novel method to generate cell-type-specific scHi-C contact maps using widely available genomic signals that enables the study of cell-type-specific chromatin interactions.
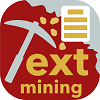
TextMining
BioCoder: A Benchmark for Bioinformatics Code Generation with Large Language Models
- Xiangru Tang, Yale University, United States
- Bill Qian, Yale University, United States
- Rick Gao, Yale University, United States
- Jiakang Chen, Yale University, United States
- Xinyun Chen, Google, United States
- Mark Gerstein, Yale University, United States
Presentation Overview: Show
Pre-trained large language models have significantly improved code generation. As these models scale up, there is an increasing need for the output to handle more intricate tasks and to be appropriately specialized to particular domains. Here, we target bioinformatics due to the amount of specialized domain knowledge, algorithms, and data operations this discipline requires. We present BioCoder, a benchmark developed to evaluate large language models (LLMs) in generating bioinformatics-specific code. BioCoder spans a broad spectrum of the field and covers cross-file dependencies, class declarations, and global variables. It incorporates 1026 Python functions and 1243 Java methods extracted from GitHub, along with 253 examples from the Rosalind Project, all pertaining to bioinformatics. Using topic modeling we show that overall coverage of the included code is representative of the full spectrum of bioinformatics calculations. BioCoder incorporates a fuzz-testing framework for evaluation. We have applied it to evaluate many models including InCoder, CodeGen, CodeGen2, SantaCoder, StarCoder, StarCoder+, InstructCodeT5+, GPT-3.5, and GPT-4. Furthermore, we finetuned StarCoder, demonstrating how our dataset can effectively enhance the performance of LLMs on our benchmark (by >15% in terms of Pass@K in certain prompt configurations and always >3%). The results highlight two key aspects of successful models: (1) Successful models accommodate a long prompt (> 2600 tokens) with full context, for functional dependencies. (2) They contain specific domain knowledge of bioinformatics, beyond just general coding knowledge. This is evident from the performance gain of GPT-3.5/4 compared to the smaller models on the benchmark (50% vs up to 25%).
Improving Medical Reasoning through Retrieval and Self-Reflection with Retrieval-Augmented Large Language Models
- Minbyul Jeong, Korea University, South Korea
- Jiwoong Sohn, Korea University, South Korea
- Mujeen Sung, School of Computing, Kyung Hee University, South Korea
- Jaewoo Kang, Korea University, AIGEN Sciences, South Korea
Presentation Overview: Show
Recent proprietary large language models (LLMs), such as GPT-4, have achieved a milestone in tackling diverse challenges in the biomedical domain, ranging from multiple-choice questions to long-form generations.
To address challenges that still cannot be handled with the encoded knowledge of LLMs, various retrieval-augmented generation (RAG) methods have been developed by searching documents from the knowledge corpus and appending them unconditionally or selectively to the input of LLMs for generation.
However, when applying existing methods to different domain-specific problems, poor generalization becomes apparent, leading to fetching incorrect documents or making inaccurate judgments.
In this paper, we introduce Self-BioRAG, a framework reliable for biomedical text that specializes in generating explanations, retrieving domain-specific documents, and self-reflecting generated responses.
We utilize 84k filtered biomedical instruction sets to train Self-BioRAG that can assess its generated explanations with customized reflective tokens.
Our work proves that domain-specific components, such as a retriever, domain-related document corpus, and instruction sets are necessary for adhering to domain-related instructions.
Using three major medical question-answering benchmark datasets, experimental results of Self-BioRAG demonstrate significant performance gains by achieving a 7.2% absolute improvement on average over the state-of-the-art open-foundation model with a parameter size of 7B or less.
We analyze that Self-BioRAG finds the clues in the question, retrieves relevant documents if needed, and understands how to answer with information from retrieved documents and encoded knowledge as a medical expert does.
We release our data and code for training our framework components and model weights
to enhance capabilities in biomedical and clinical domains.
MolLM: A Unified Language Model for Integrating Biomedical Text with 2D and 3D Molecular Representations
- Xiangru Tang, Yale University, United States
- Andrew Tran, Yale University, United States
- Jeffrey Tan, Yale University, United States
- Mark Gerstein, Yale University, United States
Presentation Overview: Show
The current paradigm of deep learning models for the joint representation of molecules and text primarily relies on 1D or 2D molecular formats, neglecting significant 3D structural information that offers valuable physical insight. This narrow focus inhibits the models’ versatility and adaptability across a wide range of modalities. Conversely, the limited research focusing on explicit 3D representation tends to overlook textual data within the biomedical domain. We present a unified pre-trained language model, MolLM, that concurrently captures 2D and 3D molecular information alongside biomedical text. MolLM consists of a text Transformer encoder and a molecular Transformer encoder, designed to encode both 2D and 3D molecular structures. To support MolLM’s self-supervised pre-training, we constructed 160K molecule-text pairings. Employing contrastive learning as a supervisory signal for cross-modal information learning, MolLM demonstrates robust molecular representation capabilities across 4 downstream tasks, including cross-modality molecule and text matching, property prediction, captioning, and text-prompted molecular editing. Through ablation, we demonstrate that the inclusion of explicit 3D representations improves performance in these downstream tasks.
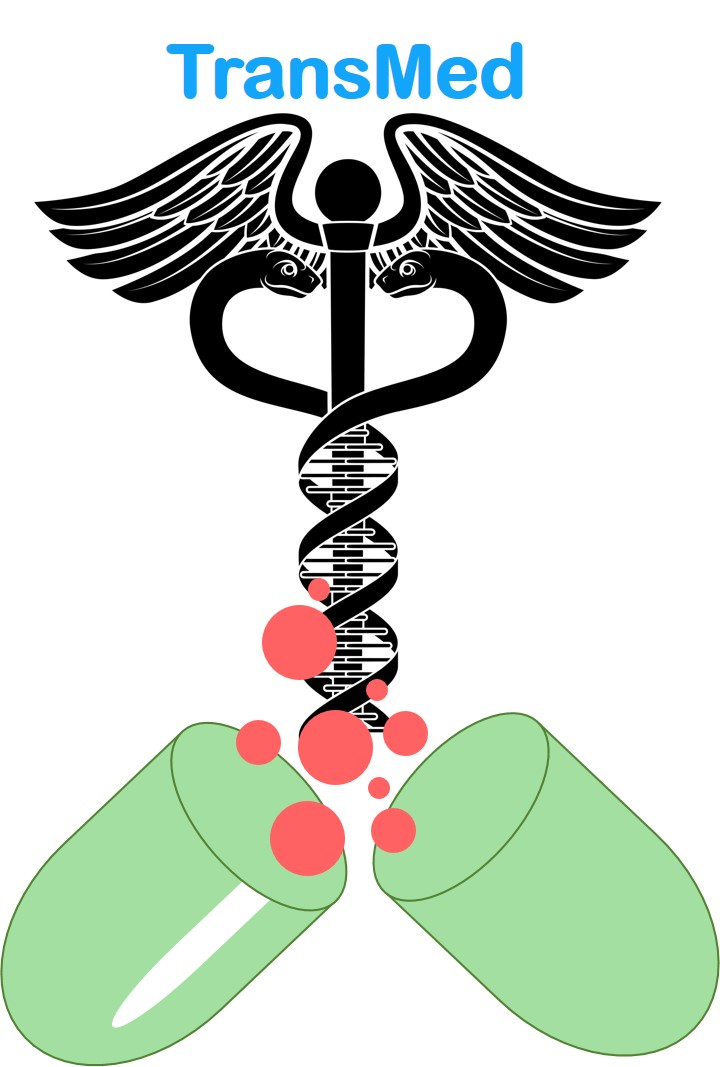
TransMed
Epidemiological topology data analysis links severe COVID-19 to RAAS and hyperlipidemia associated metabolic syndrome conditions
- Daniel E. Platt, IBM Research, NY, United States
- Aritra Bose, IBM Research, NY, United States
- Kahn Rhrissorrakrai, IBM Research, NY, United States
- Chaya Levovitz, IBM Research, NY, United States
- Laxmi Parida, IBM Research, NY, United States
Presentation Overview: Show
The emergence of COVID-19 created incredible worldwide challenges but offers unique opportunities to understand the physiology of its risk factors and their interactions with complex disease conditions, such as metabolic syndrome. To address the challenges of discovering clinically relevant interactions, we employed a unique approach for epidemiological analysis powered by Redescription-based TDA (RTDA). Here RTDA was applied to Explorys data to discover associations among severe COVID19 and metabolic syndrome. This approach was able to further explore the probative value of drug prescriptions to capture the involvement of RAAS and hypertension with COVID-19, as well as modification of risk factor impact by hyperlipidemia on severe COVID-19. RTDA found higher-order relationships between RAAS pathway and severe COVID-19 along with demographic variables of age, gender, and comorbidities such as obesity, statin prescriptions, hyperlipidemia, chronic kidney failure and disproportionately affecting African Americans. RTDA combined with CuNA (Cumulant-based Network Analysis) yielded an higher-order interaction network derived from cumulants that furthered supported the central role that RAAS plays. TDA techniques can provide a novel outlook beyond typical logistic regressions in epidemiology. From an observational cohort of electronic medical records, it can find out how RAAS drugs interact with comorbidities, such as hypertension and hyperlipidemia, of patients with severe bouts of COVID-19. Where single variable association tests with outcome can struggle, TDA's higher-order interaction network between different variables enables the discovery of the comorbidities of a disease such as COVID-19 work in concert.
PhiHER2: Phenotype-informed weakly supervised model for HER2 status prediction from pathological images
- Chaoyang Yan, College of Computer Science, Centre for Bioinformatics and Intelligent Medicine, Nankai University, China
- Jialiang Sun, College of Computer Science, Centre for Bioinformatics and Intelligent Medicine, Nankai University, China
- Yiming Guan, College of Computer Science, Centre for Bioinformatics and Intelligent Medicine, Nankai University, China
- Jiuxin Feng, College of Computer Science, Centre for Bioinformatics and Intelligent Medicine, Nankai University, China
- Hong Liu, The Second Surgical Department of Breast Cancer, Tianjin Medical University Cancer Institute & Hospital, China
- Jian Liu, College of Computer Science, Centre for Bioinformatics and Intelligent Medicine, Nankai University, China
Presentation Overview: Show
Motivation: HER2 status identification enables physicians to assess the prognosis risk and determine the treatment schedule for patients. In clinical practice, pathological slides serve as the gold standard, offering morphological information on cellular structure and tumoral regions. Computational analysis of pathological images has the potential to discover morphological patterns associated with HER2 molecular targets and achieve precise status prediction. However, pathological images are typically equipped with high-resolution attributes, and HER2 expression in breast cancer images often manifests the intratumoral heterogeneity.
Results: We present a phenotype-informed weakly-supervised multiple instance learning architecture (PhiHER2) for the prediction of the HER2 status from pathological images of breast cancer. Specifically, a hierarchical prototype clustering module is designed to identify representative phenotypes across whole slide images. These phenotype embeddings are then integrated into a cross-attention module, enhancing feature interaction and aggregation on instances. This yields a prototype-based feature space that leverages the intratumoral morphological heterogeneity for HER2 status prediction. Extensive results demonstrate that PhiHER2 captures a better WSI-level representation by the typical phenotype guidance and significantly outperforms existing methods on real-world datasets. Additionally, interpretability analyses of both phenotypes and WSIs provide explicit insights into the heterogeneity of morphological patterns associated with molecular HER2 status.
TA-RNN: an Attention-based Time-aware Recurrent Neural Network Architecture for Electronic Health Records
- Mohammad Al Olaimat, University of North Texas, United States
- Serdar Bozdag, University of North Texas, United States
Presentation Overview: Show
Motivation: Electronic Health Records (EHR) represent a comprehensive resource of a patient's medical history. EHR are essential for utilizing advanced technologies such as deep learning (DL), enabling healthcare providers to analyze extensive data, extract valuable insights, and make precise and data-driven clinical decisions. DL methods such as Recurrent Neural Networks (RNN) have been utilized to analyze EHR to model disease progression and predict diagnosis. However, these methods do not address some inherent irregularities in EHR data such as irregular time intervals between clinical visits. Furthermore, most DL models are not interpretable. In this study, we propose two interpretable DL architectures based on RNN, namely Time-Aware RNN (TA-RNN) and TA-RNN-Autoencoder (TA-RNN-AE) to predict patient’s clinical outcome in EHR at next visit and multiple visits ahead, respectively. To mitigate the impact of irregular time intervals, we propose incorporating time embedding of the elapsed times between visits. For interpretability, we propose employing a dual-level attention mechanism that operates between visits and features within each visit.
Results: The results of the experiments conducted on Alzheimer’s Disease Neuroimaging Initiative (ADNI) and National Alzheimer’s Coordinating Center (NACC) datasets indicated superior performance of proposed models for predicting Alzheimer’s Disease (AD) compared to state-of-the-art and baseline approaches based on F2 and sensitivity. Additionally, TA-RNN showed superior performance on Medical Information Mart for Intensive Care (MIMIC-III) dataset for mortality prediction. In our ablation study, we observed enhanced predictive performance by incorporating time embedding and attention mechanisms. Finally, investigating attention weights helped identify influential visits and features in predictions.
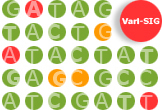
VarI
Representing Mutations for Predicting Cancer Drug Response
- Patrick Wall, UC San Diego, United States
- Trey Ideker, UC San Diego, United States
Presentation Overview: Show
Motivation. Predicting cancer drug response requires a comprehensive assessment of many mutations present across a tumor genome. While current drug response models generally use a binary mutated/unmutated indicator for each gene, not all mutations in a gene are equivalent.
Results. Here, we construct and evaluate a series of predictive models based on leading methods for quantitative mutation scoring. These methods include VEST4 and CADD, which score the likely impact of a mutation on normal gene function, and CHASMplus, which scores the likelihood the mutation drives cancer. These models capture cellular responses to dabrafenib, which specifically targets BRAF V600 mutations, whereas models based on binary mutation status do not. These performance improvements generalize to other drug responses, extending genetic indications for PIK3CA, ERBB2, EGFR, PARP1, and ABL1 inhibitors.
Conclusion. Introducing quantitative mutation features in drug response models increases predictive performance and mechanistic understanding.
Availability. Source code and a sample input dataset are available at https://github.com/pgwall/qms.