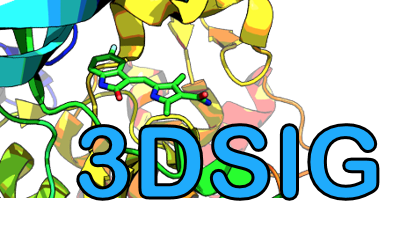
3DSIG
CATH-ddG: towards robust mutation effect prediction on protein–protein interactions out of CATH homologous superfamily
- Guanglei Yu, Central South University, China
- Xuehua Bi, Xinjiang Medical University, China
- Teng Ma, Central South University, China
- Yaohang Li, Old Dominion University, United States
- Jianxin Wang, Central South University, China
Presentation Overview: Show
Motivation: Protein-protein interactions (PPIs) are fundamental aspects in understanding biological processes. Accurately predicting the effects of mutations on PPIs remains a critical requirement for drug design and disease mechanistic studies. Recently, deep learning models using protein 3D structures have become predominant for predicting mutation effects. However, significant challenges remain in practical applications, in part due to the considerable disparity in generalization capabilities between easy and hard mutations. Specifically, a hard mutation is defined as one with its maximum TM-score < 0.6 when compared to the training set. Additionally, compared to physics-based approaches, deep learning models may overestimate performance due to potential data leakage.
Results:We propose new training/test splits that mitigate data leakage according to the CATH homologous superfamily. Under the constraints of physical energy, protein 3D structures and CATH domain objectives, we employ a hybrid noise strategy as data augmentation and present a geometric encoder scenario, named CATH-ddG, to represent the mutational microenvironment differences between wild-type and mutated protein complexes. Additionally, we fine-tune ESM2 representations by incorporating a lightweight nonlinear module to achieve the transferability of sequence co-evolutionary information. Finally, our study demonstrates that CATH-ddG framework provides enhanced generalization by outperforming other baselines on non-superfamily leakage splits, which plays a crucial role in exploring robust mutation effect regression prediction. Independent case studies demonstrate successful enhancement of binding affinity on 419 antibody variants to human epidermal growth factor receptor 2 (HER2) and 285 variants in the receptor-binding domain (RBD) of SARS-CoV-2 to angiotensin-converting enzyme 2 (ACE2) receptor.
DivPro: Diverse Protein Sequence Design with Direct Structure Recovery Guidance
- Xinyi Zhou, The Chinese University of Hong Kong, Hong Kong
- Guibao Shen, The Hong Kong University of Science and Technology, Guangzhou, China
- Yingcong Chen, The Hong Kong University of Science and Technology, Hong Kong
- Guangyong Chen, Hangzhou Institute of Medicine, Chinese Academy of Sciences, China
- Pheng Ann Heng, The Chinese University of Hong Kong, Hong Kong
Presentation Overview: Show
Motivation: Structure-based protein design is crucial for designing proteins with novel structures and functions, which aims to generate sequences that fold into desired structures. Current deep learning-based methods primarily focus on training and evaluating models using sequence recovery-based metrics. However, this approach overlooks the inherent ambiguity in the relationship between protein sequences and structures. Relying solely on sequence recovery as a training objective limits the models’ ability to produce diverse sequences that maintain similar structures. These limitations become more pronounced when dealing with remote homologous proteins, which share functional and structural similarities despite low sequence identity.
Results: Here, we present DivPro, a model that learns to design diverse sequences that can fold into similar structures. To improve sequence diversity, instead of learning a single fixed sequence representation for an input structure as in existing methods, DivPro learns a probabilistic sequence space from which diverse sequences could be sampled. We leverage the recent advancements in in-silico protein structure prediction. By incorporating structure prediction results as training guidance, DivPro ensures that sequences sampled from this learned space reliably fold into the target structure. We conduct extensive experiments on three sequence design benchmarks and evaluated the structures of designed sequences using structure prediction models including AlphaFold2. Results show that DivPro can maintain high structure recovery while significantly improve the sequence diversity.
FlowDock: Geometric Flow Matching for Generative Protein-Ligand Docking and Affinity Prediction
- Alex Morehead, University of Missouri-Columbia, United States
- Jianlin Cheng, University of Missouri-Columbia, United States
Presentation Overview: Show
Motivation: Powerful generative models of protein-ligand structure have recently been proposed, but few of these methods support both flexible protein-ligand docking and affinity estimation. Of those that do, none can directly model multiple binding ligands concurrently or have been rigorously benchmarked on pharmacologically relevant drug targets, hindering their widespread adoption in drug discovery efforts.
Results: In this work, we propose FlowDock, a deep geometric generative model based on conditional flow matching that learns to directly map unbound (apo) structures to their bound (holo) counterparts for an arbitrary number of binding ligands. Furthermore, FlowDock provides predicted structural confidence scores and binding affinity values with each of its generated protein-ligand complex structures, enabling fast virtual screening of new (multi-ligand) drug targets. For the commonly-used PoseBusters Benchmark dataset, FlowDock achieves a 51% blind docking success rate using unbound (apo) protein input structures and without any information derived from multiple sequence alignments, and for the challenging new DockGen-E dataset, FlowDock matches the performance of single-sequence Chai-1 for binding pocket generalization. Additionally, in the ligand category of the 16th community-wide Critical Assessment of Techniques for Structure Prediction (CASP16), FlowDock ranked among the top-5 methods for pharmacological binding affinity estimation across 140 protein-ligand complexes, demonstrating the efficacy of its learned representations in virtual screening.
Availability and implementation: Source code, data, and pre-trained models are available at https://github.com/BioinfoMachineLearning/FlowDock.
OrgNet: Orientation-gnostic protein stability assessment using convolutional neural networks.
- Ilya Buyanov, Constructor University, Germany
- Anastasia Sarycheva, Tetra D GmbH, Germany
- Petr Popov, Tetra D GmbH, Germany
Presentation Overview: Show
Accurately predicting the impact of single-point mutations on protein stability is crucial for elucidating molecular
mechanisms underlying diseases in life sciences and advancing protein engineering in biotechnology. With recent advances in deep learning and protein structure prediction, deep learning approaches are expected to surpass existing methods for predicting protein thermostability. However, structure-based deep learning models, specifically convolutional neural networks, are affected by orientation biases, leading to inconsistent predictions with respect to the input protein orientation. In this study, we present OrgNet, a novel orientation-gnostic deep learning model employing three-dimensional convolutional neural networks to predict protein thermostability change upon point mutation. OrgNet encodes protein structures as voxel grids, enabling the model to capture fine-grained, spatially localized atomic features. OrgNet implements spatial transforms to standardize input protein orientations, thus eliminating orientation bias problem. When evaluated on established benchmarks, including Ssym and S669, OrgNet achieves state-of-the-art performance, demonstrating superior accuracy and robust performance compared to existing methods. OrgNet is available at https://github.com/i-Molecule/OrgNet.
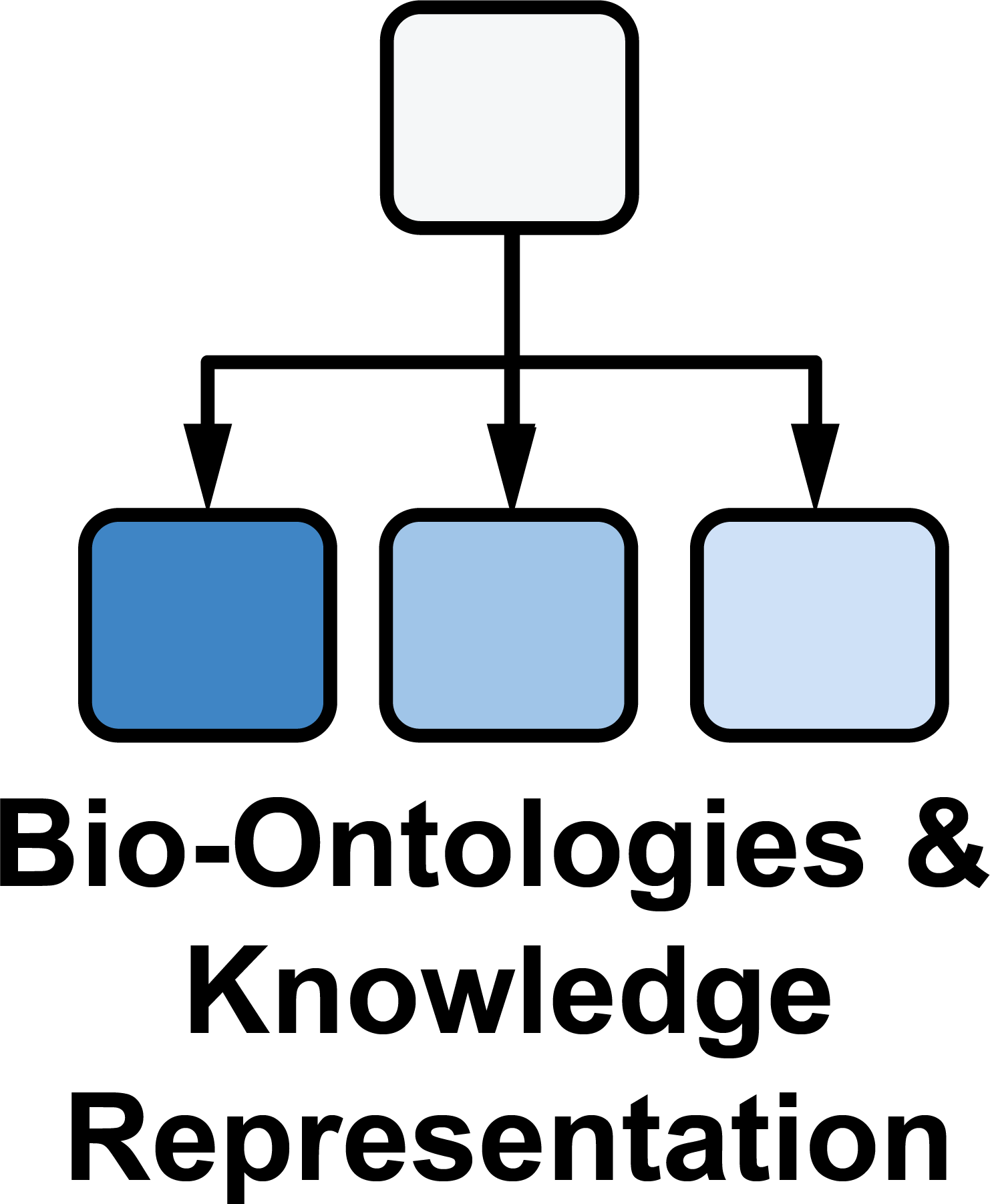
BOKR
ScGOclust: leveraging gene ontology to find functionally analogous cell types between distant species
- Yuyao Song, European Bioinformatics Institute, United Kingdom
- Yanhui Hu, Department of Genetics, Harvard Medical School, United States
- Julian Dow, School of Molecular Biosciences, University of Glasgow, United Kingdom
- Norbert Perrimon, Department of Genetics, Harvard Medical School and Howard Hughes Medical Institute, United States
- Irene Papatheodorou, European Bioinformatics Institute; Earlham Institute and University of East Anglia, United Kingdom
Presentation Overview: Show
Basic biological processes are shared across animal species, yet their cellular mechanisms are profoundly diverse. Comparing cell-type gene expression between species reveals conserved and divergent cellular functions. However, as phylogenetic distance increases, gene-based comparisons become less informative. The Gene Ontology (GO) knowledgebase offers a solution by serving as the most comprehensive resource of gene functions across a vast diversity of species, providing a bridge for distant species comparisons. Here, we present scGOclust, a computational tool that constructs de novo cellular functional profiles using GO terms, facilitating systematic and robust comparisons within and across species. We applied scGOclust to analyse and compare the heart, gut and kidney between mouse and fly, and whole-body data from C.elegans and H.vulgaris. We show that scGOclust effectively recapitulates the function spectrum of different cell types, characterises functional similarities between homologous cell types, and reveals functional convergence between unrelated cell types. Additionally, we identified subpopulations within the fly crop that show circadian rhythm-regulated secretory properties and hypothesize an analogy between fly principal cells from different segments and distinct mouse kidney tubules. We envision scGOclust as an effective tool for uncovering functionally analogous cell types or organs across distant species, offering fresh perspectives on evolutionary and functional biology.
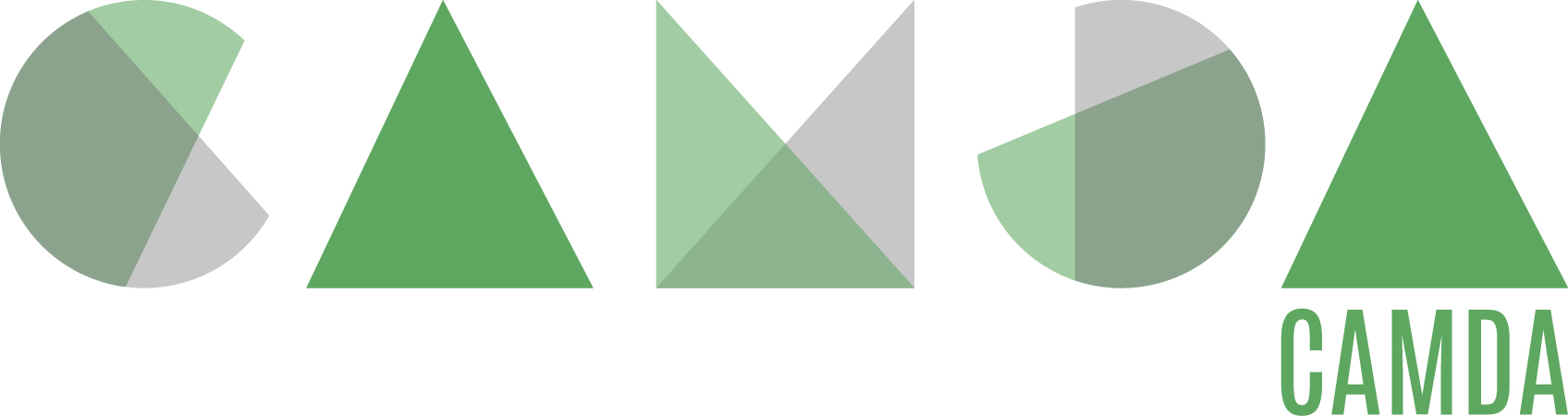
CAMDA
HI-MGSyn: A Hypergraph and Interaction-aware Multi-Granularity Network for Predicting Synergistic Drug Combinations
- Yuexi Gu, School of Mathematics and Statistics, Xi’an Jiaotong University, Shaanxi, People’s Republic of China, China
- Jian Zu, School of Mathematics and Statistics, Xi’an Jiaotong University, Shaanxi, People’s Republic of China, China
- Yongheng Sun, School of Mathematics and Statistics, Xi’an Jiaotong University, Shaanxi, People’s Republic of China, China
- Louxin Zhang, Department of Mathematics and Centre for Data Science and Machine Learning,National University of Singapore,Singapore, Singapore
Presentation Overview: Show
Motivation: Drug combinations can not only enhance drug efficacy but also effectively reduce toxic side effects and mitigate drug resistance. With the advancement of drug combination screening technologies, large amounts of data have been generated. The availability of large data enables researchers to develop deep learning methods for predicting drug targets for synergistic combination. However, these methods still lack sufficient accuracy for practical use, and most overlook the biological significance of their models.
Results: We propose the HI-MGSyn (Hypergraph and Interaction-aware Multi-granularity Network for Drug Synergy Prediction) model, which integrates a coarse-granularity module and a fine-granularity module to predict drug combination synergy. The former utilizes a hypergraph to capture global features, while the latter employs interaction-aware attention to simulate biological processes by modeling substructure-substructure and substructure-cell line interactions. HI-MGSyn outperforms state-of-the-art machine learning models on our validation datasets extracted from the DrugComb and GDSC2 databases. Furthermore, the fact that five of the 12 novel synergistic drug combinations predicted by HI-MGSyn are strongly supported by experimental evidence in the literature underscores its practical potential.
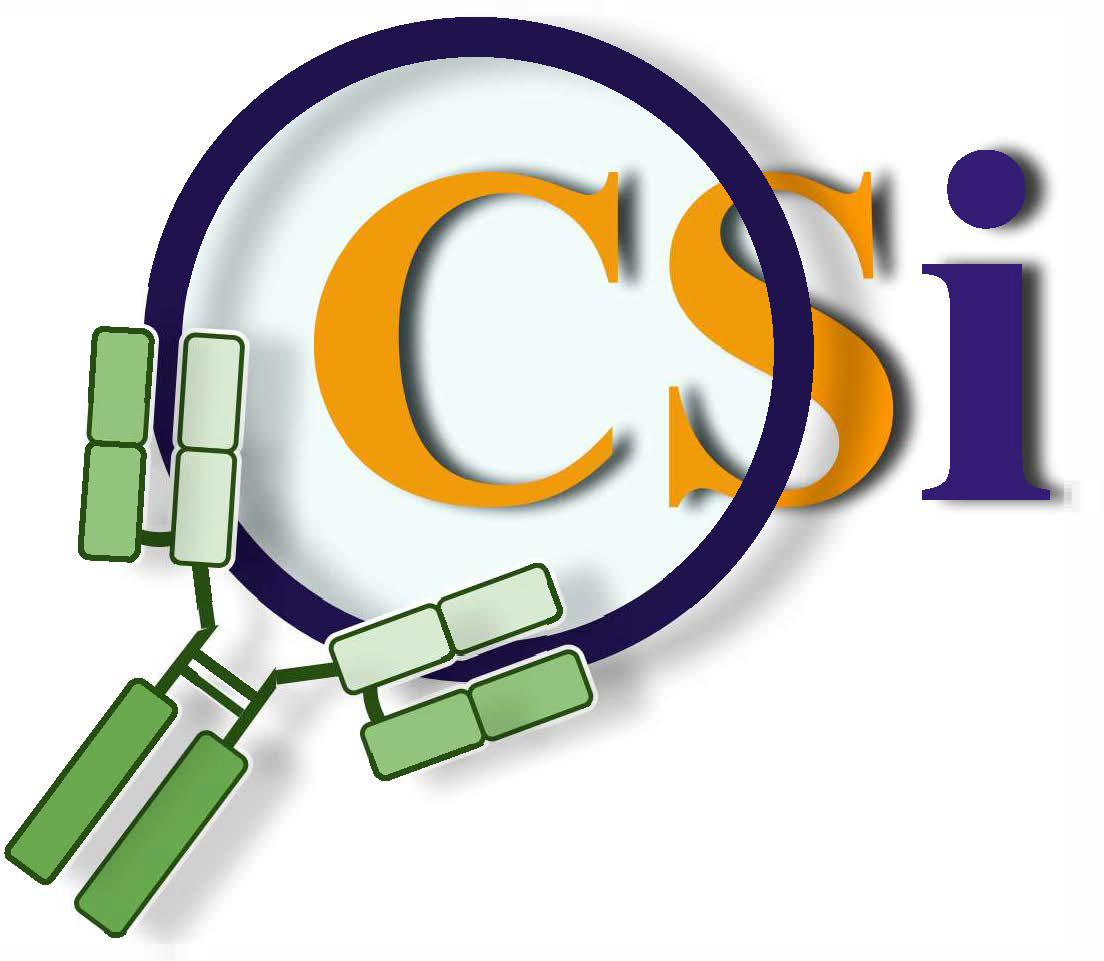
CSI
Iterative Attack-and-Defend Framework for Improving TCR-Epitope Binding Prediction Models
- Pengfei Zhang, School of Computing and Augmented Intelligence & Biodesign Institute, Arizona State University, United States
- Hao Mei, School of Computing and Augmented Intelligence & Biodesign Institute, Arizona State University, United States
- Seojin Bang, Google DeepMind, United States
- Heewook Lee, School of Computing and Augmented Intelligence & Biodesign Institute, Arizona State University, United States
Presentation Overview: Show
Reliable TCR-epitope binding prediction models are essential for development of adoptive T cell therapy and vaccine design. These models often struggle with false positives, which can be attributed to the limited data coverage in existing negative sample datasets. Common strategies for generating negative samples, such as pairing with background TCRs or shuffling within pairs, fail to account for model-specific vulnerabilities or biologically implausible sequences. To address these challenges, we propose an iterative attack-and-defend framework that systematically identifies and mitigates weaknesses in TCR-epitope prediction models. During the attack phase, a Reinforcement Learning from AI Feedback (RLAIF) framework is used to attack a prediction model by generating biologically implausible sequences that can easily deceive the model. During the defense phase, these identified false positives are incorporated into fine-tuning dataset, enhancing the model's ability to detect false positives. A comprehensive negative control dataset can be obtained by iteratively attacking and defending the model. This dataset can be directly used to improve model robustness, eliminating the need for users to conduct their own attack-and-defend cycles. We apply our framework to five existing binding prediction models, spanning diverse architectures and embedding strategies to show its efficacy. Experimental results show that our approach significantly improves these models' ability to detect adversarial false positives. The combined dataset constructed from these experiments also provides a benchmarking tool to evaluate and refine prediction models.
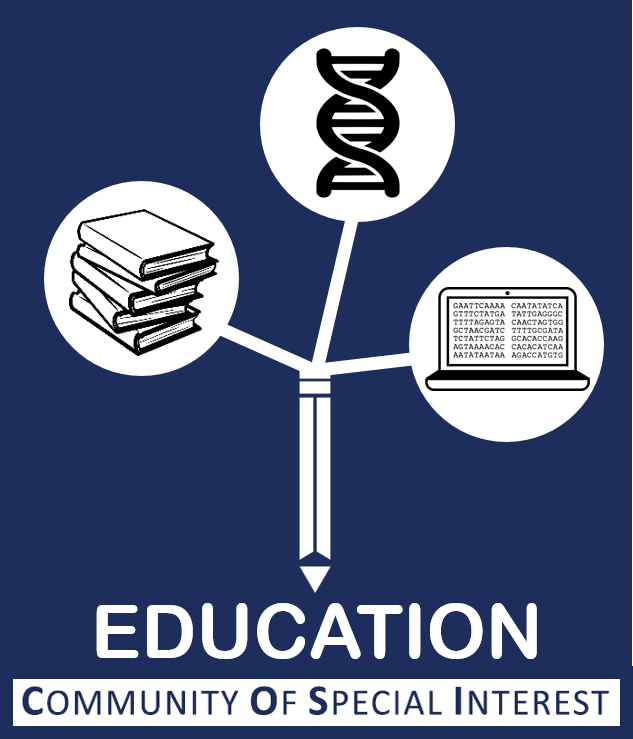
Education
An educator framework for organizing Wikipedia editathons for computational biology
- Nelly Sélem-Mojica, Centro de Ciencias Matemáticas, Universidad Nacional Autónoma de México, Mexico
- Tiago Lubiana, Department of Genetics and Evolutionary Biology, Institute of Biosciences, University of São Paulo, Brazil
- Toni Hermoso Pulido, Centre for Genomic Regulation, Barcelona Institute of Science and Technology, Spain
- Aarón Gallego-Crespo, University Medical Center of the Johannes Gutenberg University, Mainz, Germany
- Tülay Karakulak, Department of Molecular Life Sciences and Swiss Institute of Bioinformatics, University of Zurich, Switzerland
- Megha Hegde, Kingston University, London, United Kingdom
- Nicolas C Näpflin, Department of Molecular Life Sciences and Swiss Institute of Bioinformatics, University of Zurich, Zurich, Switzerland
- Audra Anjum, Ohio University, United States
- Pradeep Eranti, Université Paris Cité, Inserm, T3S, F-75006 Paris, France
- Dan DeBlasio, Ray and Stephanie Lane Computational Biology Department, Carnegie Mellon University, Pittsburgh, United States
- Jorge Noé García-Chávez, Laboratory of Agrogenomics Sciences, Universidad Nacional Autónoma de México, Mexico
- Cynthia Paola Rangel-Chavez, Biochemical Engineering Division, Tecnológico Nacional de México/Instituto Tecnológico Superior de Irapuato, Mexico
- Divanery Rodriguez-Gomez, Biochemical Engineering Division,Tecnológico Nacional de México/Instituto Tecnológico Superior de Irapuato, Mexico
- Varinia López-Ramírez, Biochemical Engineering Division,Tecnológico Nacional de México/Instituto Tecnológico Superior de Irapuato, Mexico
- Juan Vázquez-Martínez, Chemical Engineering Division,Tecnológico Nacional de México/Instituto Tecnológico Superior de Irapuato, Mexico
- Lonnie Welch, Ohio University, United States
- Alastair Kilpatrick, Centre for Regenerative Medicine, The University of Edinburgh, United Kingdom
- Farzana Rahman, Kingston University, London, United Kingdom
Presentation Overview: Show
Motivation
Wikipedia is a vital open educational resource in computational biology; however, a significant knowledge gap exists between English and Non-English Wikipedias. Reducing this knowledge gap via intensive editing events, or ‘editathons’, would be beneficial in reducing language barriers that disadvantage learners whose native language is not English.
Results
We present a framework to guide educators in organizing editathons for learners to improve and create relevant Wikipedia articles. As a case study, we present the results of an editathon held at the 2024 ISCB Latin America conference, in which ten new articles were created in Spanish Wikipedia. We also present a web tool, ‘compbio-on-wiki’, which identifies relevant English Wikipedia articles missing in other languages. We demonstrate the value of editathons to expand the accessibility and visibility of computational biology content in multiple languages.
Availability and Implementation
Source code for the compbio-on-wiki Toolforge site is available at: https://github.com/lubianat/compbio-on-wiki
Automated Assignment Grading with Large Language Models: Insights From a Bioinformatics Course
- Pavlin G. Poličar, University of Ljubljana, Faculty of Computer and Information Science, Slovenia
- Martin Špendl, University of Ljubljana, Faculty of Computer and Information Science, Slovenia
- Tomaž Curk, University of Ljubljana, Faculty of Computer and Information Science, Slovenia
- Blaž Zupan, University of Ljubljana, Faculty of Computer and Information Science, Slovenia
Presentation Overview: Show
Providing students with individualized feedback through assignments is a cornerstone of education that supports their learning and development. Studies have shown that timely, high-quality feedback plays a critical role in improving learning outcomes. However, providing personalized feedback on a large scale in classes with large numbers of students is often impractical due to the significant time and effort required. Recent advances in natural language processing and large language models (LLMs) offer a promising solution by enabling the efficient delivery of personalized feedback. These technologies can reduce the workload of course staff while improving student satisfaction and learning outcomes. Their successful implementation, however, requires thorough evaluation and validation in real classrooms.
We present the results of a practical evaluation of LLM-based graders for written assignments in the 2024/25 iteration of the Introduction to Bioinformatics course at the University of Ljubljana. Over the course of the semester, more than 100 students answered 36 text-based questions, most of which were automatically graded using LLMs. In a blind study, students received feedback from both LLMs and human teaching assistants without knowing the source, and later rated the quality of the feedback. We conducted a systematic evaluation of six commercial and open-source LLMs and compared their grading performance with human teaching assistants. Our results show that with well-designed prompts, LLMs can achieve grading accuracy and feedback quality comparable to human graders. Our results also suggest that open-source LLMs perform as well as commercial LLMs, allowing schools to implement their own grading systems while maintaining privacy.
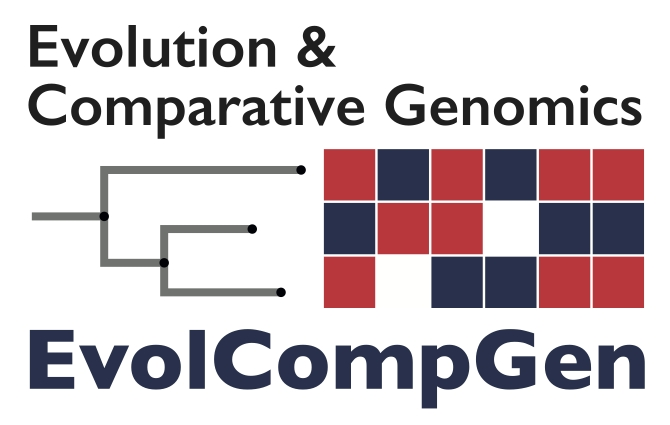
EvolCompGen
Bayesian inference of fitness landscapes via tree-structured branching processes
- Xiang Ge Luo, ETH Zurich, Switzerland
- Jack Kuipers, ETH Zurich, D-BSSE, Computational Biology Group, Switzerland
- Kevin Rupp, ETH Zurich, Switzerland
- Koichi Takahashi, MD Anderson Cancer Center, United States
- Niko Beerenwinkel, ETH Zurich, Switzerland
Presentation Overview: Show
Motivation: The complex dynamics of cancer evolution, driven by mutation and selection, underlies the molecular heterogeneity observed in tumors. The evolutionary histories of tumors of different patients can be encoded as mutation trees and reconstructed in high resolution from single-cell sequencing data, offering crucial insights for studying fitness effects of and epistasis among mutations. Existing models, however, either fail to separate mutation and selection or neglect the evolutionary histories encoded by the tumor phylogenetic trees.
Results: We introduce FiTree, a tree-structured multi-type branching process model with epistatic fitness parameterization and a Bayesian inference scheme to learn fitness landscapes from single-cell tumor mutation trees. Through simulations, we demonstrate that FiTree outperforms state-of-the-art methods in inferring the fitness landscape underlying tumor evolution. Applying FiTree to a single-cell acute myeloid leukemia dataset, we identify epistatic fitness effects consistent with known biological findings and quantify uncertainty in predicting future mutational events. The new model unifies probabilistic graphical models of cancer progression with population genetics, offering a principled framework for understanding tumor evolution and informing therapeutic strategies.
Fair molecular feature selection unveils universally tumor lineage-informative methylation sites in colorectal cancer
- Xuan Li, University of Maryland, College Park, United States
- Yuelin Liu, University of Maryland, College Park, United States
- Alejandro Schäffer, National Cancer Institute, United States
- Stephen Mount, University of Maryland, College Park, United States
- Cenk Sahinalp, National Cancer Institute, United States
Presentation Overview: Show
In the era of precision medicine, performing comparative analysis over diverse patient populations is a fundamental step towards tailoring healthcare interventions. However, the critical aspect of fairly selecting molecular features across multiple patients is often overlooked. To address this challenge, we introduce FALAFL (FAir muLti-sAmple Feature seLection), an algorithmic approach based on combinatorial optimization. FALAFL is designed to perform feature selection in sequencing data which ensures a balanced selection of features from all patient samples in a cohort. We have applied FALAFL to the problem of selecting lineage-informative CpG sites within a cohort of colorectal cancer patients subjected to low read coverage single-cell methylation sequencing. Our results demonstrate that FALAFL can rapidly and robustly determine the optimal set of CpG sites, which are each well covered by cells across the vast majority of the patients, while ensuring that in each patient a large proportion of these sites have high read coverage. An analysis of the FALAFL-selected sites reveals that their tumor lineage-informativeness exhibits a strong correlation across a spectrum of diverse patient profiles. Furthermore, these universally lineage-informative sites are highly enriched in the inter-CpG island regions. FALAFL brings unsupervised fairness considerations into the molecular feature selection from single-cell sequencing data obtained from a patient cohort. We hope that it will aid in designing panels for diagnostic and prognostic purposes and help propel fair data science practices in the exploration of complex diseases.
Fast tumor phylogeny regression via tree-structured dual dynamic programming
- Henri Schmidt, Princeton University, United States
- Yuanyuan Qi, University of Illinois at Urbana–Champaign, United States
- Ben Raphael, Princeton University, United States
- Mohammed El-Kebir, University of Illinois at Urbana-Champaign, United States
Presentation Overview: Show
Reconstructing the evolutionary history of tumors from bulk DNA sequencing of multiple tissue samples remains a challenging computational problem, requiring simultaneous deconvolution of the tumor tissue and inference of its evolutionary history. Recently, phylogenetic reconstruction methods have made significant progress by breaking the reconstruction problem into two parts: a regression problem over a fixed topology and a search over tree space. While effective techniques have been developed for the latter search problem, the regression problem remains a bottleneck in both method design and implementation due to the lack of fast, specialized algorithms. Here, we introduce fastppm, a fast tool to solve the regression problem via tree-structured dual dynamic programming. fastppm supports arbitrary, separable convex loss functions including the L2, piecewise linear, binomial and beta-binomial loss and provides asymptotic improvements for the L2 and piecewise linear loss over existing algorithms. We find that fastppm empirically outperforms both specialized and general purpose regression algorithms, obtaining 50-450x speedups while providing as accurate solutions as existing approaches. Incorporating fastppm into several phylogeny inference algorithms immediately yields up to 400x speedups, requiring only a small change to the program code of existing software. Finally, fastppm enables analysis of low-coverage bulk DNA sequencing data on both simulated data and in a patient-derived mouse model of colorectal cancer, outperforming state-of-the-art phylogeny inference algorithms in terms of both accuracy and runtime.
Recomb-Mix: fast and accurate local ancestry inference
- Yuan Wei, University of Central Florida, United States
- Degui Zhi, University of Texas Health Science Center at Houston, United States
- Shaojie Zhang, University of Central Florida, United States
Presentation Overview: Show
Motivation: The availability of large genotyped cohorts brings new opportunities for revealing the high-resolution genetic structure of admixed populations via local ancestry inference (LAI), the process of identifying the ancestry of each segment of an individual haplotype. Though current methods achieve high accuracy in standard cases, LAI is still challenging when reference populations are more similar (e.g., intra-continental), when the number of reference populations is too numerous, or when the admixture events are deep in time, all of which are increasingly unavoidable in large biobanks.
Results: In this work, we present a new LAI method, Recomb-Mix. Recomb-Mix integrates the elements of existing methods of the site-based Li and Stephens model and introduces a new graph collapsing trick to simplify counting paths with the same ancestry label readout. Through comprehensive benchmarking on various simulated datasets, we show that Recomb-Mix is more accurate than existing methods in diverse sets of scenarios while being competitive in terms of resource efficiency. We expect that Recomb-Mix will be a useful method for advancing genetics studies of admixed populations.
Availability and Implementation: The implementation of Recomb-Mix is available at https://github.com/ucfcbb/Recomb-Mix.
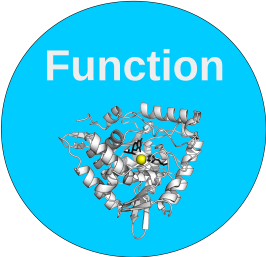
Function
GOAnnotator: Accurate protein function annotation using automatically retrieved literature
- Huiying Yan, Fudan University, China
- Hancheng Liu, Fudan University, China
- Shaojun Wang, Fudan University, China
- Shanfeng Zhu, Fudan University, China
Presentation Overview: Show
Automated protein function prediction/annotation (AFP) is vital for understanding biological processes and advancing biomedical research. Existing text-based AFP methods including the state-of-the-art method, GORetriever, rely on expert-curated relevant literature, which is costly and time-consuming, and covers only a small portion of the proteins in UniProt. To overcome this limitation, we propose GOAnnotator, a novel framework for automated protein function annotation. It consists of two key modules: PubRetriever, a hybrid system for retrieving and re-ranking relevant literature, and GORetriever+, an enhanced module for identifying Gene Ontology (GO) terms from the retrieved texts. Extensive experiments over three benchmark datasets demonstrate that GOAnnotator delivers high-quality functional annotations, surpassing GORetriever by uncovering unique literature and predicting additional functions. These results highlight its great potential to streamline and enhance the annotation of protein functions without relying on manual curation.
Semi-Supervised Data-Integrated Feature Importance Enhances Performance and Interpretability of Biological Classification Tasks
- Jun Kim, Department of Biomedical Data Science, Stanford University, United States
- Russ Altman, Department of Biomedical Data Science, Stanford University, United States
Presentation Overview: Show
Accurate model performance on training data does not ensure alignment between the model’s feature weighting patterns and human knowledge, which can limit the model’s relevance and applicability. We propose Semi-Supervised Data-Integrated Feature Importance (DIFI), a method that numerically integrates a priori knowledge, represented as a sparse knowledge map, into the model’s feature weighting. By incorporating the similarity between the knowledge map and the feature map into a loss function, DIFI causes the model’s feature weighting to correlate with the knowledge. We show that DIFI can improve the performance of neural networks using two biological tasks. In the first task, cancer type prediction from gene expression profiles was guided by identities of cancer type-specific biomarkers. In the second task, enzyme/non-enzyme classification from protein sequences was guided by the locations of the catalytic residues. In both tasks, DIFI leads to improved performance and feature weighting that is interpretable. DIFI is a novel method for injecting knowledge to achieve model alignment and interpretability.
GenCompBio
ADME-Drug-Likeness: Enriching Molecular Foundation Models via Pharmacokinetics-Guided Multi-Task Learning for Drug-likeness Prediction
- Dongmin Bang, Seoul National University, South Korea
- Juyeon Kim, Seoul National University, South Korea
- Haerin Song, Seoul National University, South Korea
- Sun Kim, Seoul National University, South Korea
Presentation Overview: Show
Recent breakthroughs in AI-driven generative models enable the rapid design of extensive molecular libraries, creating an urgent need for fast and accurate drug-likeness evaluation. Traditional approaches, however, rely heavily on structural descriptors and overlook pharmacokinetic (PK) factors such as absorption, distribution, metabolism, and excretion (ADME). Furthermore, existing deep-learning models neglect the complex interdependencies among ADME tasks, which play a pivotal role in determining clinical viability.
We introduce ADME-DL (drug likeness), a novel two-step pipeline that first enhances diverse range of Molecular Foundation Models (MFMs) via sequential ADME multi-task learning. By enforcing an A→D→M→E flow—grounded in a data-driven task dependency analysis that aligns with established pharmacokinetic principles—our method more accurately encodes PK information into the learned embedding space.
In Step 2, the resulting ADME-informed embeddings are leveraged for drug-likeness classification, distinguishing approved drugs from negative sets drawn from chemical libraries.
Through comprehensive experiments, our sequential ADME multi-task learning achieves up to +2.4% improvement over state-of-the-art baselines, and enhancing performance across tested MFMs by up to +18.2%. Case studies with clinically annotated drugs validate that respecting the PK hierarchy produces more relevant predictions, reflecting drug discovery phases. These findings underscore the potential of ADME-DL to significantly enhance the early-stage filtering of candidate molecules, bridging the gap between purely structural screening methods and PK-aware modeling.
Efficient 3D kernels for molecular property prediction
- Ankit, Indian Institute of Technology Palakkad, India
- Sahely Bhadra, Indian Institute of Technology Palakkad, India
- Juho Rousu, Aalto University, Finland
Presentation Overview: Show
This paper addresses the challenge of incorporating 3-dimensional structural information in graph kernels for machine learning-based virtual screening, a crucial task in drug discovery. Existing kernels that capture 3D information often suffer from high computational complexity, which limits their scalability. To overcome this, we propose the 3-dimensional chain motif graph kernel (c-MGK), which effectively integrates essential 3D structural properties—bond length, bond angle, and torsion angle—within the three-hop neighborhood of each atom in a molecule. In addition, we introduce a more computationally efficient variant, the 3-dimensional graph hopper kernel (3DGHK), which reduces the complexity from the state-of-the-art $\mathcal{O}(n^{6})$ (for the 3D pharmacophore kernel) to $\mathcal{O}(n^{2}(m + \log(n) + \delta^2 +dT^{6}))$. Here, $n$ is the number of nodes, $T$ is the highest degree of the node, $m$ is the number of edges, $\delta$ is the diameter of the graph, and $d$ is the dimension of the attributes of the nodes. We conducted experiments on 21 datasets, demonstrating that 3DGHK not only outperforms state-of-the-art 2D and 3D graph kernels, but also surpasses deep learning models in classification accuracy, offering a powerful and scalable solution for virtual screening tasks.
FACT: Feature Aggregation and Convolution with Transformers for predicting drug classification code
- Gwang-Hyeon Yun, Yonsei University - Mirae Campus, South Korea
- Jong-Hoon Park, Yonsei University - Mirae Campus, South Korea
- Young-Rae Cho, Yonsei University - Mirae Campus, South Korea
Presentation Overview: Show
Motivation: Drug repositioning, identifying new therapeutic applications for existing drugs, can significantly reduce the time and cost involved in drug development. Recent studies have explored the use of Anatomical Therapeutic Chemical (ATC) codes in drug repositioning, offering a systematic framework to predict ATC codes for a drug. The ATC classification system organizes drugs according to their chemical properties, pharmacological actions, and therapeutic effects. However, its complex hierarchical structure and the limited scalability at higher levels present significant challenges for achieving accurate ATC code prediction.
Results: We propose a novel approach to predict ATC codes of drugs, named Feature Aggregation and Convolution with Transformer models (FACT). This method computes three types of drug similarities, incorporating ATC code similarity with hierarchical weights and masked drug-ATC code associations. These features are then aggregated for each target drug-ATC code pair and processed through a convolution-transformer encoder to generate three embeddings. The embeddings are finally used to estimate the probability of an association between the target pair. The experimental results demonstrate that the proposed method achieves an AUROC of 0.9805 and an AUPRC of 0.9770 at level 4 of the ATC codes, outperforming the previous methods by 15.05% and 18.42%, respectively. This study highlights the effectiveness of integrating diverse drug features and the potential of transformer-based models in ATC code prediction.
From High-Throughput Evaluation to Wet-Lab Studies: Advancing Mutation Effect Prediction with a Retrieval-Enhanced Model
- Yang Tan, East China University of Science and Technology, China
- Ruilin Wang, East China University of Science and Technology, China
- Banghao Wu, Shanghai Jiao Tong University, China
- Liang Hong, Shanghai Jiao Tong University, China
- Bingxin Zhou, Shanghai Jiao Tong University, China
Presentation Overview: Show
Enzyme engineering is a critical approach for producing enzymes that meet industrial and research demands by modifying wild-type proteins to enhance properties such as catalytic activity and thermostability. Beyond traditional methods like directed evolution and rational design, recent advancements in deep learning offer cost-effective and high-performance alternatives. By encoding implicit coevolutionary patterns, these pre-trained models have become powerful tools for mutation effect prediction, with the central challenge being to uncover the intricate relationships among protein sequence, structure, and function. In this study, we present VenusREM, a retrieval-enhanced protein language model designed to capture local amino acid interactions across both spatial and temporal scales. VenusREM achieves state-of-the-art performance on 217 assays from the ProteinGym benchmark. Beyond high-throughput open benchmark validations, we conducted a low-throughput post-hoc analysis on more than 30 mutants to verify the model’s ability to improve the stability and binding affinity of a VHH antibody. We also validated the practical effectiveness of VenusREM by designing 10 novel mutants of a DNA polymerase and performing wet-lab experiments to evaluate their enhanced activity at elevated temperatures. Both in silico and experimental evaluations not only confirm the reliability of VenusREM as a computational tool for enzyme engineering but also demonstrate a comprehensive evaluation framework for future computational studies in mutation effect prediction. The implementation is publicly available at https://github.com/tyang816/VenusREM.
Harnessing Deep Learning for Proteome-Scale Detection of Amyloid Signaling Motifs
- Krzysztof Pysz, Politechnika Wrocławska, Poland
- Jakub Gałązka, Politechnika Wrocławska, Poland
- Witold Dyrka, Politechnika Wrocławska, Poland
Presentation Overview: Show
Amyloid signaling sequences adopt the cross-β fold that is capable of self-replication in the templating process. Propagation of the amxyloid fold from the receptor to the effector protein is used for signal transduction in the immune response pathways in animals, fungi and bacteria. So far, a dozen of families of amyloid signaling motifs (ASMs) have been classified. Unfortunately, due to the wide variety of ASMs it is difficult to identify them in large protein databases available, which limits the possibility of conducting experimental studies. To date, various deep learning (DL) models have been applied across a range of protein-related tasks, including domain family classification and the prediction of protein structure and protein-protein interactions. In this study, we develop tailor-made bidirectional LSTM and BERT-based architectures to model ASM, and compare their performance against a state-of-the-art machine learning grammatical model. Our research is focused on developing a discriminative model of generalized amyloid signaling motifs, capable of detecting ASMs in large data sets. The DL-based models are trained on a diverse set of motif families and a global negative set, and used to identify ASMs from remotely related families. We analyze how both models represent the data and demonstrate that the DL-based approaches effectively detect ASMs, including novel motifs, even at the genome scale.
HIDE: Hierarchical cell-type Deconvolution
- Dennis Völkl, Institute of Theoretical Physics, University of Regensburg, Germany
- Malte Mensching-Buhr, Department of Medical Bioinformatics, University Medical Center Göttingen, Germany
- Thomas Sterr, Institute of Theoretical Physics, University of Regensburg, Germany
- Sarah Bolz, Institute of Human Anatomy and Embryology, University of Regensburg, Germany
- Andreas Schäfer, Institute of Theoretical Physics, University of Regensburg, Germany, Germany
- Nicole Seifert, Department of Medical Bioinformatics, University Medical Center Göttingen, Germany
- Jana Tauschke, Peter L. Reichertz Institute for Medical Informatics of TU Braunschweig and Hannover Medical School, Germany
- Austin Rayford, Department of Biomedicine and Centre for Cancer Biomarkers, University of Bergen, Norway
- Oddbjørn Straume, Cancer Clinic, Haukeland University Hospital, Norway
- Helena U. Zacharias, Peter L. Reichertz Institute for Medical Informatics of TU Braunschweig and Hannover Medical School, Germany
- Sushma Nagaraja Grellscheid, Computational Biology Unit, University of Bergen, Norway
- Tim Beissbarth, University Medicine Göttingen, Germany
- Michael Altenbuchinger, Department of Medical Bioinformatics, University Medical Center Göttingen, Germany
- Franziska Görtler, Cancer Clinic, Haukeland University Hospital, Norway
Presentation Overview: Show
Motivation: Cell-type deconvolution is a computational approach to infer cellular distributions from bulk transcriptomics data. Several methods have been proposed, each with its own advantages and disadvantages. Reference based approaches make use of archetypic transcriptomic profiles representing individual cell types. Those reference profiles are ideally chosen such that the observed bulks can be reconstructed as a linear combination thereof. This strategy, however, ignores the fact that cellular populations arise through the process of cellular differentiation, which entails the gradual emergence of cell groups with diverse morphological and functional characteristics.
Results: Here, we propose Hierarchical cell-type Deconvolution (HIDE), a cell-type deconvolution approach which incorporates a cell hierarchy for improved performance and interpretability. This is achieved by a hierarchical procedure that preserves estimates of major cell populations while inferring their respective subpopulations. We show in simulation studies that this procedure produces more reliable and more consistent results than other state-of-the-art approaches. Finally, we provide an example application of HIDE to explore breast cancer specimens from TCGA.
Availability: A python implementation of HIDE is available at zenodo: doi:10.5281/zenodo.14724906.
Precise Prediction of Hotspot Residues in Protein-RNA Complexes Using Graph Attention Networks and Pre-trained Protein Language Models
- Siyuan Shen, Central South University, China
- Jie Chen, Xinjiang University, China
- Zhijian Huang, Central South University, China
- Yuanpeng Zhang, Xinjiang University, China
- Ziyu Fan, Central South University, China
- Yuting Kong, Xinjiang Institute of Engineering, China
- Lei Deng, Central South University, China
Presentation Overview: Show
Motivation: Protein-RNA interactions play a pivotal role in biological processes and disease mechanisms, with hotspot residues being critical for targeted drug design. Traditional experimental methods for identifying hotspot residues are often inefficient and expensive. Moreover, many existing prediction methods rely heavily on high-resolution structural data, which may not always be available. Consequently, there is an urgent need for an accurate and efficient sequence-based computational approach for predicting hotspot residues in protein-RNA complexes.
Results: In this study, we introduce DeepHotResi, a sequence-based computational method designed to predict hotspot residues in protein-RNA complexes. DeepHotResi leverages a pre-trained protein language model to predict protein structure and generate an amino acid contact map. To enhance feature representation, DeepHotResi integrates the Squeeze-and-Excitation (SE) module, which processes diverse amino acid-level features. Next, it constructs an amino acid feature network from the contact map and SE-Module-derived features. Finally, DeepHotResi employs a Graph Attention Network (GAT) to model hotspot residue prediction as a graph node classification task. Experimental results demonstrate that DeepHotResi outperforms state-of-the-art methods, effectively identifying hotspot residues in protein-RNA complexes with superior accuracy on the test set.
RVINN: A Flexible Modeling for Inferring Dynamic Transcriptional and Post-Transcriptional Regulation Using Physics-Informed Neural Networks
- Osamu Muto, Division of Cancer Informatics, Nagoya University Graduate School of Medicine, Japan
- Zhongliang Guo, Division of Cancer Systems Biology, Aichi Cancer Center Research Institute, Japan
- Rui Yamaguchi, Division of Cancer Systems Biology, Aichi Cancer Center Research Institute, Japan
Presentation Overview: Show
Dynamic gene expression is controlled by transcriptional and post-transcriptional regulation. Recent studies on transcriptional bursting and buffering have increasingly highlighted the dynamic gene regulatory mechanisms. However, direct measurement techniques still face various constraints and require complementary methodologies, which are both comprehensive and versatile. To address this issue, inference approaches based on transcriptome data and differential equation models representing the messenger RNA lifecycle have been proposed. However, the inference of complex dynamics under diverse experimental conditions and biological scenarios remains challenging. In this study, we developed a flexible modeling using Physics-Informed Neural Networks and demonstrated its performance using simulation and experimental data. Our model has the ability to computationally revalidate and visualize dynamic biological phenomena, such as transcriptional ripple, co-bursting, and buffering in a breast cancer cell line. Furthermore, our results suggest putative molecular mechanisms underlying these phenomena. We propose a novel approach for inferring transcriptional and post-transcriptional regulation and expect to offer valuable insights for experimental and systems biology.
SVQ-MIL: Small-Cohort Whole Slide Image Classification via Split Vector Quantization
- Dawei Shen, The University of Tokyo, Japan
- Yao-Zhong Zhang, The University of Tokyo, Japan
- Keita Tamura, Hiroshima University, Japan
- Yohei Okubo, The University of Tokyo, Japan
- Seiya Imoto, The University of Tokyo, Japan
Presentation Overview: Show
Whole Slide Images (WSIs) are high-resolution digital scans of microscope slides that play important roles in pathological analysis. Recent advancements in deep learning have significantly improved WSI classification.
However, challenges persist, particularly in small cohorts with limited training samples.
Multiple Instance Learning (MIL) has emerged as a leading framework for WSI classification. In MIL, each WSI is divided into image tiles, and each tile is represented by an embedding generated by a pretrained vision foundation model. Nevertheless, these embeddings are general-purpose and typically exhibit high variability, rendering them suboptimal for specific classification tasks.
In this study, we introduce SVQ-MIL, a generalized framework that leverages Split Vector Quantization (SVQ) with a learnable codebook to quantize instance embeddings. The learned codebook reduces embedding variability and abbreviates the input for MIL model, making it advantageous for small-cohort datasets. Additionally, SVQ-MIL enhances model interpretability by providing a profiling of the WSI instances through the learned codebook. Experimental evaluations demonstrate that SVQ-MIL achieves competitive performance compared with the-state-of-the-art methods on two benchmark datasets. \textcolor{red}{The source code is available at \url{https://github.com/aCoalBall/SVQMIL}.}
Trustworthy Causal Biomarker Discovery: A Multiomics Brain Imaging Genetics based Approach
- Jin Zhang, Northwestern Polytechnical University, China
- Yan Yang, Northwestern Polytechnical University, China
- Muheng Shang, Northwestern Polytechnical University, China
- Lei Guo, Northwestern Polytechnical University, China
- Daoqiang Zhang, Nanjing University of Aeronautics and Astronautics, China
- Lei Du, Northwestern Polytechnical University, China
Presentation Overview: Show
Discovering genetic variations underpinning brain disorders is important to understand their pathogenesis. Indirect associations or spurious causal relationships pose a threat to the reliability of biomarker discovery for brain disorders, potentially misleading or incurring bias in subsequent decision-making. Unfortunately, the stringent selection of reliable biomarker candidates for brain disorders remains a predominantly unexplored challenge. In this paper, to fill this gap, we propose a fresh and powerful scheme, referred to as the Causality-aware Genotype intermediate Phenotype Correlation Approach (Ca-GPCA). Specifically, we design a bidirectional association learning framework, integrated with a parallel causal variable decorrelation module and sparse variable regularizer module, to identify trustworthy causal biomarkers. A disease diagnosis module is further incorporated to ensure accurate diagnosis and identification of causal effects for pathogenesis. Additionally, considering the large computational burden incurred by high-dimensional genotype-phenotype covariances, we develop a fast and efficient strategy to reduce the runtime and prompt practical availability and applicability. Extensive experimental results on four simulation data and real neuroimaging genetic data clearly show that Ca-GPCA outperforms state-of-the-art methods with excellent built-in interpretability. This can provide novel and reliable insights into the underlying pathogenic mechanisms of brain disorders.
Understanding the Sources of Performance in Deep Drug Response Models Reveals Insights and Improvements
- Nikhil Branson, queen mary university of london, United Kingdom
- Pedro Rodriguez Cutillas, Barts Cancer Institute, QMUL, United Kingdom
- Conrad Bessant, Queen Mary - University of London, United Kingdom
Presentation Overview: Show
Anti-cancer drug response prediction (DRP) using cancer cell lines (CLs) is crucial in stratified medicine and drug discovery. Recently new deep learning models for DRP have improved performance over their predecessors. However, different models use different input data types and architectures making it hard to find the source of these improvements. Here we consider published DRP models that report state-of-the-art performance predicting continuous response values. These models take chemical structures of drugs and omics profiles of CLS as input. By experimenting with these models and comparing with our simple benchmarks we show that no performance comes from drug features, instead, performance is due to the transcriptomics CL profiles. Furthermore, we show that, depending on the testing type, much of the current reported performance is a property of the training target values. We address these limitations by creating BinaryET and BinaryCB that predict binary drug response values, guided by the hypothesis that this reduces the noise in the drug efficacy data. Thus, better aligning them with biochemistry that can be learnt from the input data. BinaryCB leverages a chemical foundation model, while BinaryET is trained from scratch using a transformer-type architecture. We show that these models learn useful chemical drug features, which is the first time this has been demonstrated for multiple testing types to our knowledge. We further show binarising the drug response values causes the models to learn useful chemical drug features. We also show that BinaryET improves performance over BinaryCB, and the published models that report state-of-the-art performance.
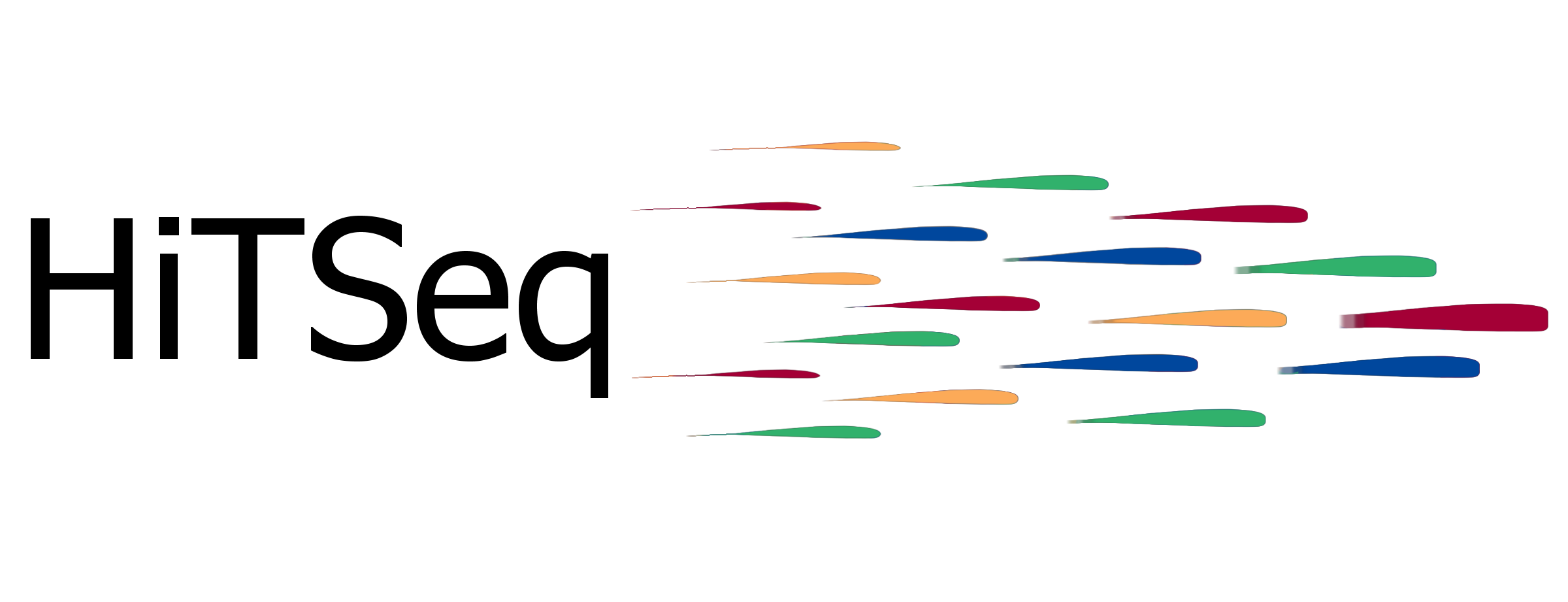
HiTSeq
Alevin-fry-atac enables rapid and memory frugal mapping of single-cell ATAC-Seq data using virtual colors for accurate genomic pseudoalignment
- Noor Pratap Singh, Department of Computer Science, University of Maryland - College Park, United States
- Jamshed Khan, Department of Computer Science, University of Maryland - College Park, United States
- Rob Patro, Department of Computer Science, University of Maryland - College Park, United States
Presentation Overview: Show
Ultrafast mapping of short reads via lightweight mapping techniques such as pseudoalignment has significantly accelerated transcriptomic and metagenomic analyses with minimal accuracy loss compared to alignment-based methods. However,
applying pseudoalignment to large genomic references, like chromosomes, is challenging due to their size and repetitive sequences. We introduce a new and modified pseudoalignment scheme that partitions each reference into “virtual colors”. These are essentially overlapping bins of fixed maximal extent on the reference sequences that are treated as distinct “colors” from the perspective of the pseudoalignment algorithm. We apply this modified pseudoalignment procedure to
process and map single-cell ATAC-seq data in our new tool alevin-fry-atac. We compare alevin-fry-atac to both Chromap and Cell Ranger ATAC. Alevin-fry-atac is highly scalable and, when using 32 threads, is approximately 2.8 times faster than Chromap (the second fastest approach) while using approximately 3 times less memory and mapping slightly more reads. The resulting peaks and clusters generated from alevin-fry-atac show high concordance with those obtained from both Chromap and the Cell Ranger ATAC pipeline, demonstrating that virtual color-enhanced pseudoalignment directly to the genome provides a fast, memory-frugal, and accurate alternative to existing approaches for single-cell ATAC-seq processing. The development of alevin-fry-atac brings single-cell ATAC-seq processing into a unified ecosystem with single-cell RNA-seq processing (via alevin-fry) to work toward providing a truly open alternative to many of the varied capabilities of CellRanger.
CREMSA: Compressed Indexing of (Ultra) Large Multiple Sequence Alignments
- Mikaël Salson, CRIStAL, UMR 9189 Université de Lille, CNRS, France
- Arthur Boddaert, Université de Lille, France
- Awa Bousso Gueye, Université de Lille, France
- Laurent Bulteau, CNRS - Université Gustave Eiffel, France, France
- Yohan Hernandez-Courbevoie, Université de Lille, France
- Camille Marchet, CNRS, France
- Nan Pan, LIX - Ecole Polytechnique, France
- Sebastian Will, Ecole Polytechnique, France
- Yann Ponty, CNRS/LIX, Polytechnique, France
Presentation Overview: Show
Recent viral outbreaks motivate a systematic collection of pathogenic genomes, including a strong focus on genomic RNA, in order to accelerate their study and monitor the apparition/spread of variants. Due to their limited length and temporal proximity of their collection, viral genomes are usually organized, and analyzed as oversized Multiple Sequence Alignments (MSAs). Such MSAs are largely ungapped, and mostly homogeneous on a column-wise level but not at a sequential level due to local variations, hindering the performances of sequential compression algorithms.
In order to enable an efficient manipulation of MSAs, including subsequent statistical analyses, we introduce CREMSA (Column-wise Run-length Encoding for Multiple Sequence Alignments), a new index that builds on sparse bitvector representations to compress an existing or streamed MSA, all the while allowing for an expressive set of accelerated requests to query the alignment without prior decompression.
Using CREMSA, a 65GB MSA consisting of 1.9M SARS-CoV 2 genomes could be compressed into 22MB using less than half a gigabyte of main memory, while supporting access requests in the order of 100ns. Such a speed up enables a comprehensive analysis of covariation over this very large MSA. We further assess the impact of the sequence ordering on the compressibility of MSAs and propose a resorting strategy that, despite the proven NP-hardness of an optimal sort, induces greatly increased compression ratios at a marginal computational cost.
Exploiting uniqueness: seed-chain-extend alignment on elastic founder graphs
- Nicola Rizzo, University of Helsinki, Finland
- Manuel Cáceres, Aalto University, Finland
- Veli Mäkinen, University of Helsinki, Finland
Presentation Overview: Show
Sequence-to-graph alignment is a central challenge of computational pangenomics. To overcome the theoretical hardness of the problem, state-of-the-art tools use seed-and-extend or seed-chain-extend heuristics to alignment. We implement a complete seed-chain-extend alignment workflow based on indexable elastic founder graphs (iEFGs) that support linear-time exact searches unlike general graphs.
We show how to construct iEFGs, find high-quality seeds, chain, and extend them at the scale of a telomere-to-telomere assembled human chromosome.
Our sequence-to-graph alignment tool and the scripts to replicate our experiments are available in https://github.com/algbio/SRFAligner.
GreedyMini: Generating low-density DNA minimizers
- Shay Golan, Reichman University and University of Haifa, Israel
- Ido Tziony, Bar-Ilan University, Israel
- Matan Kraus, Bar-Ilan University, Israel
- Yaron Orenstein, Bar-Ilan University, Israel
- Arseny Shur, Bar Ilan University, Israel
Presentation Overview: Show
Motivation:
Minimizers are the most popular k-mer selection scheme in algorithms and data structures analyzing high-throughput sequencing (HTS) data. In a minimizer scheme, the smallest k-mer by some predefined order is selected as the representative of a sequence window containing w consecutive k-mers, which results in overlapping windows often selecting the same k-mer. Minimizers that achieve the lowest frequency of selected k-mers over a random DNA sequence, termed the expected density, are desired for improved performance of HTS analyses. Yet, no method to date exists to generate minimizers that achieve minimum expected density. Moreover, for k and w values used by common HTS algorithms and data structures there is a gap between densities achieved by existing selection schemes and the theoretical lower bound.
Results:
We developed GreedyMini, a toolkit of methods to generate minimizers with low expected or particular density, to improve minimizers, to extend minimizers to larger alphabets, k, and w, and to measure the expected density of a given minimizer efficiently. We demonstrate over various combinations of k and w values, including those of popular HTS methods, that GreedyMini can generate DNA minimizers that achieve expected densities very close to the lower bound, and both expected and particular densities much lower compared to existing selection schemes. Moreover, we show that GreedyMini's k-mer rank-retrieval time is comparable to common k-mer hash functions. We expect GreedyMini to improve the performance of many HTS algorithms and data structures and advance the research of k-mer selection schemes.
LYCEUM: Learning to call copy number variants on low coverage ancient genomes
- Mehmet Alper Yilmaz, Bilkent University, Turkey
- Ahmet Arda Ceylan, Bilkent University, Turkey
- Gun Kaynar, Carnegie Mellon University, United States
- A. Ercument Cicek, Bilkent University, Turkey
Presentation Overview: Show
Motivation: Copy number variants (CNVs) are pivotal in driving phenotypic variation that facilitates species adaptation. They are significant contributors to various disorders, making ancient genomes crucial for uncovering the genetic origins of disease susceptibility across populations. However, detecting CNVs in ancient DNA (aDNA) samples poses substantial challenges due to several factors: (i) aDNA is often highly degraded; (ii) contamination from microbial DNA and DNA from closely related species introduce additional noise into sequencing data; and finally, (iii) the typically low coverage of aDNA renders accurate CNV detection particularly difficult.
Conventional CNV calling algorithms, which are optimized for high coverage read-depth signals, underperform under such conditions.
Results: To address these limitations, we introduce LYCEUM, the first machine learning-based CNV caller for aDNA. To overcome challenges related to data quality and scarcity, we employ a two-step training strategy. First, the model is pre-trained on whole genome sequencing data from the 1000 Genomes Project, teaching it CNV-calling capabilities similar to conventional methods. Next, the model is fine-tuned using high-confidence CNV calls derived from only a few existing high-coverage aDNA samples. During this stage, the model adapts to making CNV calls based on the downsampled read depth signals of the same aDNA samples. LYCEUM achieves accurate detection of CNVs even in typically low-coverage ancient genomes. We also observe that the segmental deletion calls made by LYCEUM show correlation with the demographic history of the samples and
exhibit patterns of negative selection inline with natural selection.
Availability: LYCEUM is available at https://github.com/ciceklab/LYCEUM.
Oarfish: Enhanced probabilistic modeling leads to improved accuracy in long read transcriptome quantification
- Zahra Zare Jousheghani, University of Maryland, College Park, United States
- Noor Pratap Singh, University of Maryland, College Park, United States
- Rob Patro, University of Maryland, College Park, United States
Presentation Overview: Show
Motivation: Long read sequencing technology is becoming an increasingly indispensable tool in genomic and transcriptomic analysis. In transcriptomics in particular, long reads offer the possibility of sequencing full-length isoforms, which can vastly simplify the identification of novel transcripts and transcript quantification. However, despite this promise, the focus of much long read method development to date has been on transcript identification, with comparatively little attention paid to quantification. Yet, due to differences in the underlying protocols and technologies, lower throughput (i.e. fewer reads sequenced per sample compared to short read technologies), as well as technical artifacts, long read quantification remains a challenge, motivating the continued development and assessment of quantification methods tailored to this increasingly prevalent type of data.
Results: We introduce a new method and corresponding user-friendly software tool for long read transcript quantification called oarfish. Our model incorporates a novel coverage score, which affects the conditional probability of fragment assignment in the underlying probabilistic model. We demonstrate, in both simulated and experimental data, that by accounting for this coverage information, oarfish is able to produce more accurate quantification estimates than existing long read quantification tools.
Availability and Implementation: Oarfish is implemented in the Rust programming language, and is made available as free and open-source software under the BSD 3-clause license. The source code is available at https://www.github.com/COMBINE-lab/oarfish.
Spatial transcriptomics deconvolution methods generalize well to spatial chromatin accessibility data
- Sarah Ouologuem, Technical University Munich, Germany
- Laura D. Martens, Technical University Munich, Germany
- Anna C. Schaar, Technical University Munich, Germany
- Maiia Shulman, Helmholtz Center Munich, Germany
- Julien Gagneur, Technical University Munich, Germany
- Fabian J. Theis, Helmholtz Center Munich, Germany
Presentation Overview: Show
Motivation: Spatially resolved chromatin accessibility profiling offers the potential to investigate gene regulatory processes within the spatial context of tissues. However, current methods typically work at spot resolution, aggregating measurements from multiple cells, thereby obscuring cell-type-specific spatial patterns of accessibility. Spot deconvolution methods have been developed and extensively benchmarked for spatial transcriptomics, yet no dedicated methods exist for spatial chromatin accessibility, and it is unclear if RNA-based approaches are applicable to that modality.
Results: Here, we demonstrate that these RNA-based approaches can be applied to spot-based chromatin accessibility data by a systematic evaluation of five top-performing spatial transcriptomics deconvolution methods. To assess performance, we developed a simulation framework that generates both transcriptomic and accessibility spot data from dissociated single-cell and targeted multiomic datasets, enabling direct comparisons across both data modalities. Our results show that Cell2location and RCTD, in contrast to other methods, exhibit robust performance on spatial chromatin accessibility data, achieving accuracy comparable to RNA-based deconvolution. Generally, we observed that RNA-based deconvolution exhibited slightly better performance compared to chromatin accessibility-based deconvolution, especially for resolving rare cell types, indicating room for future development of specialized methods. In conclusion, our findings demonstrate that existing deconvolution methods can be readily applied to chromatin accessibility-based spatial data. Our work provides a simulation framework and establishes a performance baseline to guide the development and evaluation of methods optimized for spatial epigenomics.
Availability: All methods, simulation frameworks, peak selection strategies, analysis notebooks and scripts are available at https://github.com/theislab/deconvATAC.
Transcriptome Assembly at Single-Cell Resolution with Beaver
- Qian Shi, The Pennsylvania State University, United States
- Qimin Zhang, The Pennsylvania State University, United States
- Mingfu Shao, The Pennsylvania State University, United States
Presentation Overview: Show
Motivation: The established single-cell RNA sequencing technologies (scRNA-seq) has revolutionized biological and biomedical research by enabling the measurement of gene expression at single-cell resolution. However, the fundamental challenge of reconstructing full-length transcripts for individual cells remains unresolved. Existing single-sample assembly approaches cannot leverage shared information across cells while meta-assembly approaches often fail to strike a balance between consensus assembly and preserving cell-specific expression signatures.
Results: We present Beaver, a cell-specific transcript assembler designed for short-read scRNA-seq data. Beaver implements a transcript fragment graph to organize individual assemblies and designs an efficient dynamic programming algorithm that searches for candidate full-length transcripts from the graph. Beaver incorporates two random forest models trained on 51 meticulously engineered features that accurately estimate the likelihood of each candidate transcript being expressed in individual cells. Our experiments, performed using both real and simulated Smart-seq3 scRNA-seq data, firmly show that Beaver substantially outperforms existing meta-assemblers and single-sample assemblers. At the same level of sensitivity, Beaver achieved 32.0%-64.6%, 13.5%-36.6%, and 9.8%-36.3% higher precision in average compared to meta-assemblers Aletsch, TransMeta, and PsiCLASS, respectively, with similar improvements over single-sample assemblers Scallop2 (10.1%-43.6%) and StringTie2 (24.3%-67.0%).
Availability: Beaver is freely available at https://github.com/Shao-Group/beaver. Scripts that reproduce the
experimental results of this manuscript are available at https://github.com/Shao-Group/beaver-test.
Ultrafast and Ultralarge Multiple Sequence Alignments using TWILIGHT
- Yu-Hsiang Tseng, University of California San Diego, United States
- Sumit Walia, University of California San Diego, United States
- Yatish Turakhia, University of California San Diego, United States
Presentation Overview: Show
Motivation: Multiple sequence alignment (MSA) is a fundamental operation in bioinformatics, yet existing MSA tools are struggling to keep up with the speed and volume of incoming data. This is because the runtimes and memory requirements of current MSA tools become untenable when processing large numbers of long input sequences and they also fail to fully harness the parallelism provided by modern CPUs and GPUs.
Results: We present TWILIGHT (Tall and Wide Alignments at High Throughput), a novel MSA tool optimized for speed, accuracy, scalability, and memory constraints, with both CPU and GPU support. TWILIGHT incorporates innovative parallelization and memory-efficiency strategies that enable it to build ultralarge alignments at high speed even on memory-constrained devices. On challenging datasets, TWILIGHT outperformed all other tools in speed and accuracy. It scaled beyond the limits of existing tools and performed an alignment of 1 million RNASim sequences within 30 minutes while utilizing less than 16 GB of memory. TWILIGHT is the first tool to align over 8 million publicly available SARS-CoV-2 sequences, setting a new standard for large-scale genomic alignment and data analysis.
Availability: TWILIGHT’s code is freely available under the MIT license at https://github.com/TurakhiaLab/TWILIGHT. The test datasets and experimental results, including our alignment of 8 million SARS-CoV-2 sequences, are available at https://zenodo.org/records/14722035.
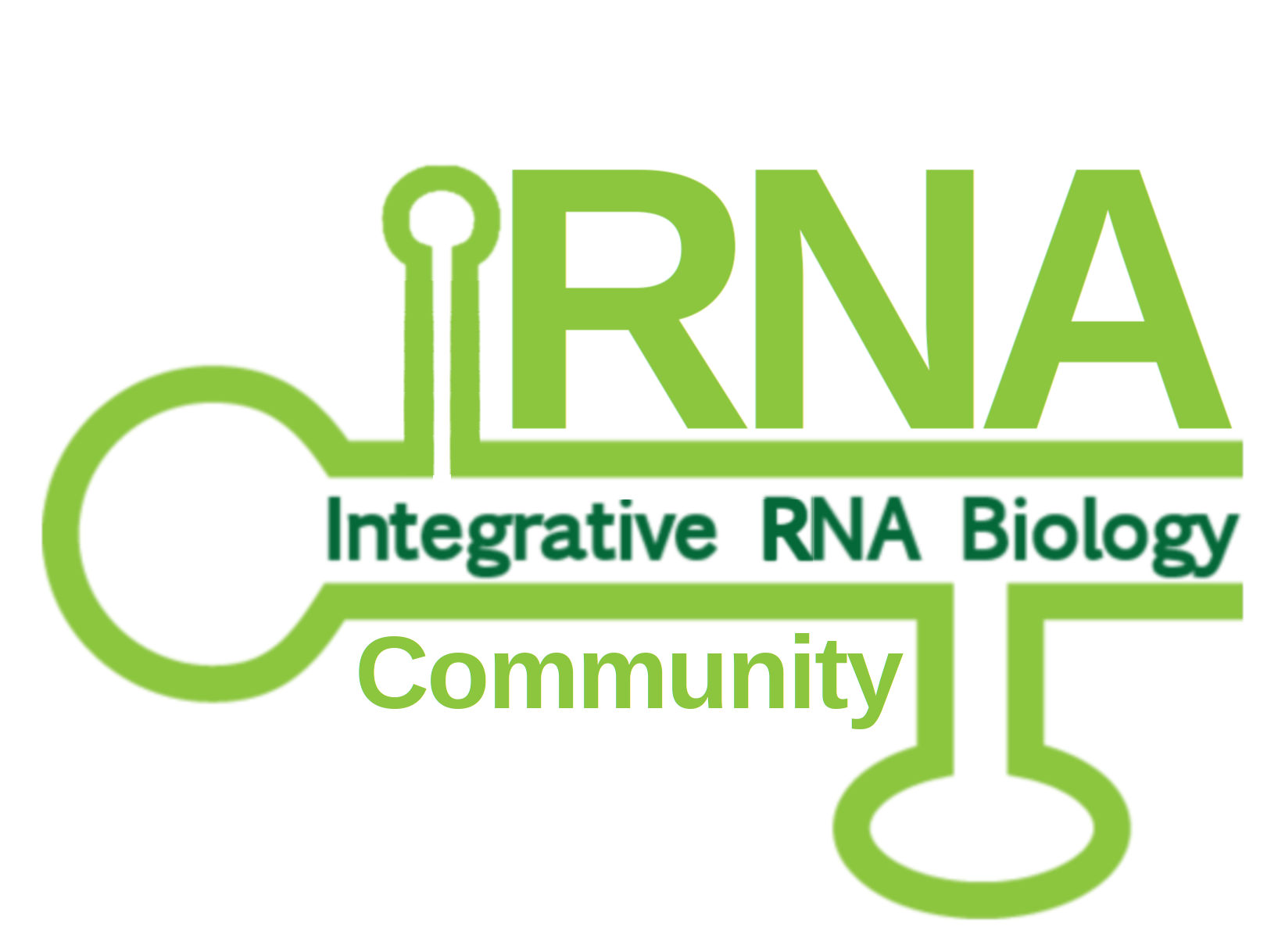
iRNA
EnsembleDesign: Messenger RNA Design Minimizing Ensemble Free Energy via Probabilistic Lattice Parsing
- Ning Dai, Oregon State University, United States
- Tianshuo Zhou, Oregon State University, United States
- Wei Yu Tang, Oregon State University, United States
- David Mathews, University of Rochester, United States
- Liang Huang, Oregon State University, United States
Presentation Overview: Show
The task of designing optimized messenger RNA (mRNA) sequences has received much attention in recent years thanks to breakthroughs in mRNA vaccines during the COVID-19 pandemic. Because most previous work aimed to minimize the minimum free energy (MFE) of the mRNA in order to improve stability and protein expression, which only considers one particular structure per mRNA sequence, millions of alternative conformations in equilibrium are neglected. More importantly, we prefer an mRNA to populate multiple stable structures and be flexible among them during translation when the ribosome unwinds it. Therefore, we consider a new objective to minimize the ensemble free energy of an mRNA, which includes all possible structures in its Boltzmann ensemble. However, this new problem is much harder to solve than the original MFE optimization. To address the increased complexity of this problem, we introduce EnsembleDesign, a novel algorithm that employs continuous relaxation to optimize the expected ensemble free energy over a distribution of candidate sequences. EnsembleDesign extends both the lattice representation of the design space and the dynamic programming algorithm from LinearDesign to their probabilistic counterparts. Our algorithm consistently outperforms LinearDesign in terms of ensemble free energy, especially on long sequences. Interestingly, as byproducts, our designs also enjoy lower average unpaired probabilities (AUP, which correlates with degradation) and flatter Boltzmann ensembles (more flexibility between conformations). Our code is available on: https://github.com/LinearFold/EnsembleDesign.
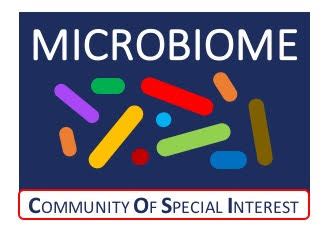
MICROBIOME
DNABERT-S: Pioneering Species Differentiation with Species-Aware DNA Embeddings
- Zhihan Zhou, Northwestern University, United States
- Weimin Wu, Northwestern University, United States
- Harrison Ho, University of California, Merced, United States
- Jiayi Wang, Northwestern University, United States
- Lizhen Shi, Northwestern University, United States
- Ramana Davuluri, Stony Brook University, United States
- Zhong Wang, Lawrence Berkeley National Laboratory, United States
- Han Liu, Northwestern University, United States
Presentation Overview: Show
We introduce DNABERT-S, a tailored genome model that develops species-aware embeddings to naturally cluster and segregate DNA sequences of different species in the embedding space. Differentiating species from genomic sequences (i.e., DNA and RNA) is vital yet challenging, since many real-world species remain uncharacterized, lacking known genomes for reference. Embedding-based methods are therefore used to differentiate species in an unsupervised manner. DNABERT-S builds upon a pre-trained genome foundation model named DNABERT-2. To encourage effective embeddings to error-prone long-read DNA sequences, we introduce Manifold Instance Mixup (MI-Mix), a contrastive objective that mixes the hidden representations of DNA sequences at randomly selected layers and trains the model to recognize and differentiate these mixed proportions at the output layer. We further enhance it with the proposed Curriculum Contrastive Learning (C$^2$LR) strategy. Empirical results on 23 diverse datasets show DNABERT-S's effectiveness, especially in realistic label-scarce scenarios. For example, it identifies twice more species from a mixture of unlabeled genomic sequences, doubles the Adjusted Rand Index (ARI) in species clustering, and outperforms the top baseline's performance in 10-shot species classification with just a 2-shot training.
GiantHunter: Accurate detection of giant virus in metagenomic data using reinforcement-learning and Monte Carlo tree search
- Fuchuan Qu, Department of Electrical Engineering, City University of Hong Kong, Hong Kong
- Cheng Peng, Department of Electrical Engineering, City University of Hong Kong, Hong Kong
- Jiaojiao Guan, Department of Electrical Engineering, City University of Hong Kong, Hong Kong
- Donglin Wang, School of Environmental Science & Engineering, Shandong University, China
- Yanni Sun, Department of Electrical Engineering, City University of Hong Kong, Hong Kong
- Jiayu Shang, Department of Information Engineering, Chinese University of Hong Kong, Hong Kong
Presentation Overview: Show
Motivation: Nucleocytoplasmic large DNA viruses (NCLDVs) are notable for their large genomes and extensive gene repertoires, which contribute to their widespread environmental presence and critical roles in processes such as host metabolic reprogramming and nutrient cycling. Metagenomic sequencing has emerged as a powerful tool for uncovering novel NCLDVs in environmental samples. However, identifying NCLDV sequences in metagenomic data remains challenging due to their high genomic diversity, limited reference genomes, and shared regions with other microbes. Existing alignment-based and machine learning methods struggle with achieving optimal trade-offs between sensitivity and precision.
Results: In this work, we present GiantHunter, a reinforcement learning-based tool for identifying NCLDVs from metagenomic data. By employing a Monte Carlo tree search strategy, GiantHunter dynamically selects representative non-NCLDV sequences as the negative training data, enabling the model to establish a robust decision boundary. Benchmarking on rigorously designed experiments shows that GiantHunter achieves high precision while maintaining competitive sensitivity, improving the F1-score by 10% and reducing computational cost by 90% compared to the second-best method. To demonstrate its real-world utility, we applied GiantHunter to 60 metagenomic datasets collected from six cities along the Yangtze River, located both upstream and downstream of the Three Gorges Dam. The results reveal significant differences in NCLDV diversity correlated with proximity to the dam, likely influenced by reduced flow velocity caused by the dam. These findings highlight GiantHunter's potential to advance our understanding of NCLDVs and their ecological roles in diverse environments.
Leveraging Large Language Models to Predict Antibiotic Resistance in Mycobacterium tuberculosis
- Conrad Testagrose, University of Florida, United States
- Sakshi Pandey, University of Florida, United States
- Mohammadali Serajian, University of Florida, United States
- Simone Marini, University of Florida, United States
- Mattia Prosperi, University of Florida, United States
- Christina Boucher, University of Florida, United States
Presentation Overview: Show
Antibiotic resistance in Mycobacterium tuberculosis (MTB) poses a significant challenge to global public health. Rapid and accurate prediction of antibiotic resistance can inform treatment strategies and mitigate the spread of resistant strains. In this study, we present a novel approach leveraging large language models (LLMs) to predict antibiotic resistance in MTB (LLMTB). Our model is trained on a large dataset of genomic data and associated resistance profiles, utilizing natural language processing techniques to capture patterns and mutations linked to resistance. The model's architecture integrates state-of-the-art transformer-based LLMs, enabling the analysis of complex genomic sequences and the extraction of critical features relevant to antibiotic resistance. We evaluate our model's performance using a comprehensive dataset of MTB strains, demonstrating its ability to achieve high performance in predicting resistance to various antibiotics. Unlike traditional machine learning methods, fine-tuning or few-shot learning open avenues for LLMs to adapt to new or emerging drugs thereby reducing reliance on extensive data curation. Beyond predictive accuracy, LLMTB uncovers deeper biological insights, identifying critical genes, intergenic regions, and novel resistance mechanisms. This method marks a transformative shift in resistance prediction and offers significant potential for enhancing diagnostic capabilities and guiding personalized treatment plans, ultimately contributing to the global effort to combat tuberculosis and antibiotic resistance. All source code is publicly available at https://github.com/ctestagrose/LLMTB.
Predicting coarse-grained representations of biogeochemical cycles from metabarcoding data
- Arnaud Belcour, Univ. Grenoble Alpes, Inria, France
- Loris Megy, Gricad, Inria, CNRS, Université Grenoble Alpes, Grenoble INP, France
- Sylvain Stephant, French Geological Survey (BRGM), France
- Caroline Michel, French Geological Survey (BRGM), France
- Sétareh Rad, French Geological Survey (BRGM), France
- Petra Bombach, Isodetect GmbH, Germany
- Nicole Dopffel, NORCE Norwegian Research Center AS, Norway
- Hidde de Jong, Univ. Grenoble Alpes, Inria, France
- Delphine Ropers, Univ. Grenoble Alpes, Inria, France
Presentation Overview: Show
Motivation: Taxonomic analysis of environmental microbial communities is now routinely performed thanks to advances in DNA sequencing. Determining the role of these communities in global biogeochemical cycles requires the identification of their metabolic functions, such as hydrogen oxidation, sulfur reduction, and carbon fixation. These functions can be directly inferred from metagenomics data, but in many environmental applications metabarcoding is still the method of choice. The reconstruction of metabolic functions from metabarcoding data and their integration into coarse-grained representations of geobiochemical cycles remains a difficult bioinformatics problem today.
Results: We developed a pipeline, called Tabigecy, which exploits taxonomic affiliations to predict metabolic functions constituting biogeochemical cycles. In a first step, Tabigecy uses the tool EsMeCaTa to predict consensus proteomes from input affiliations. To optimise this process, we generated a precomputed database containing information about 2,404 taxa from UniProt. The consensus proteomes are searched using bigecyhmm, a newly developed Python package relying on Hidden Markov Models to identify key enzymes involved in metabolic function of biogeochemical cycles. The metabolic functions are then projected on coarse-grained representation of the cycles. We applied Tabigecy to two salt cavern datasets and validated its predictions with microbial activity and hydrochemistry measurements performed on the samples. The results highlight the utility of the approach to investigate the impact of microbial communities on geobiochemical processes.
Availability: The Tabigecy pipeline is available at https://github.com/ArnaudBelcour/tabigecy.
The Python package bigecyhmm and the precomputed EsMeCaTa database are also separately available at \https://github.com/ArnaudBelcour/bigecyhmm and https://doi.org/10.5281/zenodo.13354073, respectively.
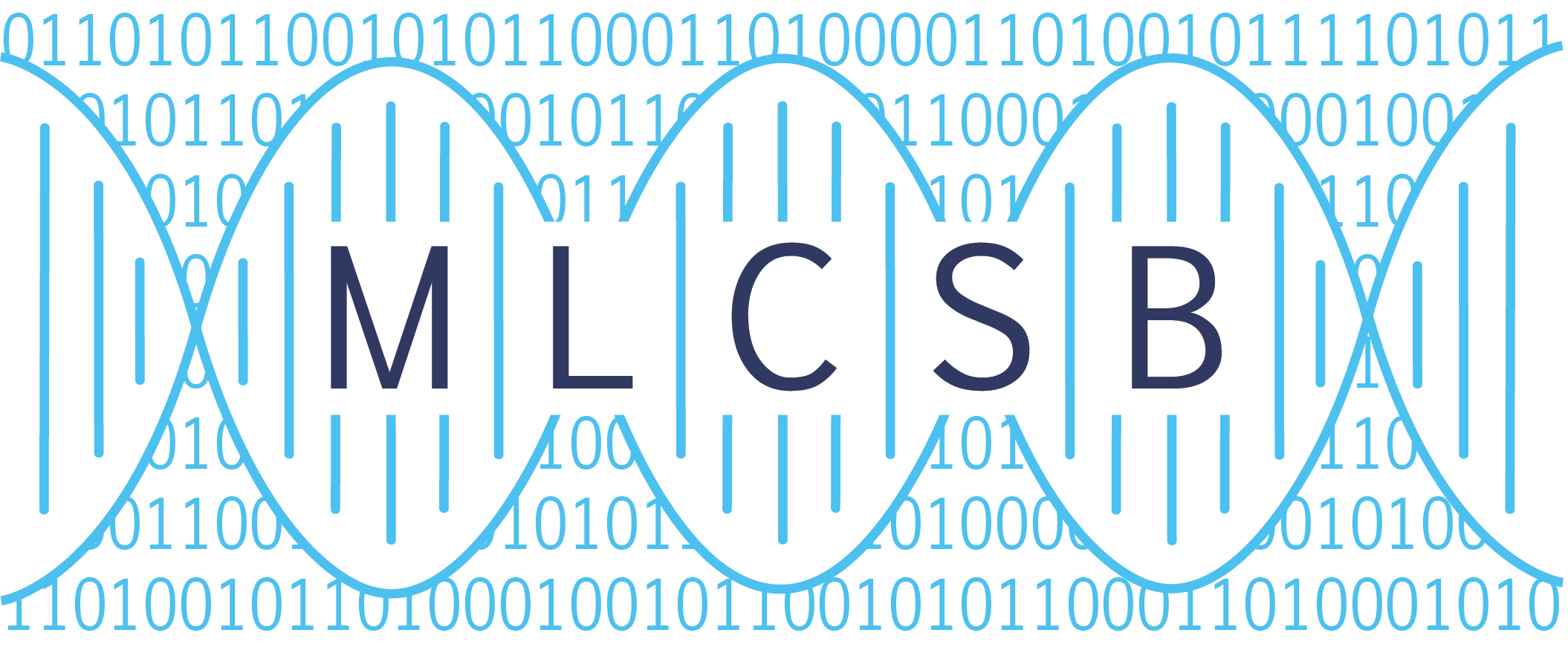
MLCSB
Accurate PROTAC targeted degradation prediction with DegradeMaster
- Jie Liu, The University of Adelaide, Australia
- Michael Roy, The University of Adelaide, Australia
- Luke Isbel, The University of Adelaide, Australia
- Fuyi Li, The University of Adelaide, Australia
Presentation Overview: Show
Motivation: Proteolysis-targeting chimeras (PROTACs) are heterobifunctional molecules that can degrade ‘undruggable’ protein of interest (POI) by recruiting E3 ligases and hijacking the ubiquitin-proteasome system. Some efforts have been made to develop deep learning-based approaches to predict the degradation ability of a given PROTAC. However, existing deep learning methods either simplify proteins and PROTACs as 2D graphs by disregarding crucial 3D spatial information or exclusively rely on limited labels for supervised learning without considering the abundant information from unlabeled data. Nevertheless, considering the potential to accelerate drug discovery, developing more accurate computational methods for PROTAC-targeted protein degradation prediction is critical.
Results: This study proposes DegradeMaster, a semi-supervised E(3)-equivariant graph neural network-based predictor for targeted degradation prediction of PROTACs. DegradeMaster leverages an E(3)-equivariant graph encoder to incorporate 3D geometric constraints into the molecular representations and utilizes a memory-based pseudo-labeling strategy to enrich annotated data during training. A mutual attention pooling module is also designed for interpretable graph representation. Experiments on both supervised and semi-supervised PROTAC datasets demonstrate that DegradeMaster outperforms state-of-the-art baselines, substantially improving AUROC by 10.5%. Case studies show DegradeMaster achieves 88.33% and 77.78% accuracy in predicting the degradability of VZ185 candidates on BRD9 and ACBI3 on KRAS mutants. Visualization of attention weights on 3D molecule graph demonstrates that DegradeMaster recognises linking and binding regions of warhead and E3 ligands and emphasizes the importance of structural information in these areas for degradation prediction. Together, this shows the potential for cutting-edge tools to highlight functional PROTAC components, thereby accelerating novel compound generation.
Deep learning models for unbiased sequence-based PPI prediction plateau at an accuracy of 0.65
- Timo Reim, Technical University of Munich; Friedrich-Alexander-Universität Erlangen-Nürnberg, Germany
- Anne Hartebrodt, Friedrich-Alexander-Universität Erlangen-Nürnberg, Germany
- David B. Blumenthal, Friedrich-Alexander-Universität Erlangen-Nürnberg, Germany
- Judith Bernett, Technical University of Munich, Germany
- Markus List, Technical University of Munich, Germany
Presentation Overview: Show
As most proteins interact with other proteins to perform their respective functions, methods to computationally predict these interactions have been developed.
However, flawed evaluation schemes and data leakage in test sets have obscured the fact that sequence-based protein-protein interaction (PPI) prediction is still an open problem. Recently, methods achieving better-than-random performance on leakage-free PPI data have been proposed. Here, we show that the use of ESM-2 protein embeddings explains this performance gain irrespective of model architecture. We compared the performance of models with varying complexity, per-protein, and per-token embeddings, as well as the influence of self- or cross-attention, where all models plateaued at an accuracy of 0.65. Moreover, we show that the tested sequence-based models cannot implicitly learn a contact map as an intermediate layer.
These results imply that other input types, such as structure, might be necessary for producing reliable PPI predictions.
Fast and scalable Wasserstein-1 neural optimal transport solver for single-cell perturbation prediction
- Yanshuo Chen, Department of Computer Science, University of Maryland, United States
- Zhengmian Hu, Department of Computer Science, University of Maryland, United States
- Wei Chen, Department of Pediatrics, UPMC Children’s Hospital of Pittsburgh, United States
- Heng Huang, Department of Computer Science, University of Maryland, United States
Presentation Overview: Show
Predicting single-cell perturbation responses requires mapping between two unpaired single-cell data distributions. Optimal transport (OT) theory provides a principled framework for constructing such mappings by minimizing transport cost. Recently, Wasserstein-2 ($W_2$) neural optimal transport solvers (\textit{e.g.}, CellOT) have been employed for this prediction task. However, $W_2$ OT relies on the general Kantorovich dual formulation, which involves optimizing over two conjugate functions, leading to a complex min-max optimization problem that converges slowly. To address these challenges, we propose a novel solver based on the Wasserstein-1 ($W_1$) dual formulation. Unlike $W_2$, the $W_1$ dual simplifies the optimization to a maximization problem over a single 1-Lipschitz function, thus eliminating the need for time-consuming min-max optimization. While solving the $W_1$ dual only reveals the transport direction and does not directly provide a unique optimal transport map, we incorporate an additional step using adversarial training to determine an appropriate transport step size, effectively recovering the transport map. Our experiments demonstrate that the proposed $W_1$ neural optimal transport solver can mimic the $W_2$ OT solvers in finding a unique and ``monotonic" map on 2D datasets. Moreover, the $W_1$ OT solver achieves performance on par with or surpasses $W_2$ OT solvers on real single-cell perturbation datasets. Furthermore, we show that $W_1$ OT solver achieves $25 \sim 45\times$ speedup, scales better on high dimensional transportation task, and can be directly applied on single-cell RNA-seq dataset with highly variable genes. Our implementation and experiments are open-sourced at \url{https://github.com/poseidonchan/w1ot}.
GPO-VAE: Modeling Explainable Gene Perturbation Responses utilizing GRN-Aligned Parameter Optimization
- Seungheun Baek, Korea University, South Korea
- Soyon Park, Korea University, South Korea
- Yan Ting Chok, Korea University, Malaysia
- Mogan Gim, Hankuk University of Foreign Studies, South Korea
- Jaewoo Kang, Korea University, Aigen Sciences, South Korea
Presentation Overview: Show
Predicting cellular responses to genetic perturbations is essential for understanding biological systems and developing targeted therapeutic strategies. While variational autoencoders (VAEs) have shown promise in modeling perturbation responses, their limited explainability poses a significant challenge, as the learned features often lack clear biological meaning. Nevertheless, model explainability is one of the most important aspects in the realm of biological AI. One of the most effective ways to achieve explainability is incorporating the concept of gene regulatory networks (GRNs) in designing deep learning models such as VAEs. GRNs elicit the underlying causal relationships between genes and are capable of explaining the transcriptional responses caused by genetic perturbation treatments. We propose GPO-VAE, an explainable VAE enhanced by GRN-aligned Parameter Optimization that explicitly models gene regulatory networks in the latent space. Our key approach is to optimize the learnable parameters related to latent perturbation effects towards GRN-aligned explainability. Experimental results on perturbation prediction show our model achieves state-of-the-art performance in predicting transcriptional responses across multiple benchmark datasets. Furthermore, additional results on evaluating the GRN inference task reveal our model's ability to generate meaningful GRNs compared to other methods. According to qualitative analysis, GPO-VAE posseses the ability to construct biologically explainable GRNs that with experimentally validated regulatory pathways.
Incorporating Hierarchical Information into Multiple Instance Learning for Patient Phenotype Prediction with scRNA-seq Data
- Chau Do, Aalto University, Finland
- Harri Lähdesmäki, Aalto University, Finland
Presentation Overview: Show
Multiple Instance Learning (MIL) provides a structured approach to patient phenotype prediction with single-cell RNA-sequencing (scRNA-seq) data. However, existing MIL methods tend to overlook the hierarchical structure inherent in scRNA-seq data, especially the biological groupings of cells, or cell types. This limitation may lead to suboptimal performance and poor interpretability at higher levels of cellular division. To address this gap, we present a novel approach to incorporate hierarchical information into the attention-based MIL framework. Specifically, our model applies the attention-based aggregation mechanism over both cells and cell types, thus enforcing a hierarchical structure on the flow of information throughout the model. Across extensive experiments, our proposed approach consistently outperforms existing models and demonstrates robustness in data-constrained scenarios. Moreover, ablation test results show that simply applying the attention mechanism on cell types instead of cells leads to improved performance, underscoring the benefits of incorporating the hierarchical groupings. By identifying the critical cell types that are most relevant for prediction, we show that our model is capable of capturing biologically meaningful associations, thus facilitating biological discoveries.
Locality-aware pooling enhances protein language model performance across varied applications
- Minh Hoang, Princeton University, United States
- Mona Singh, Princeton University, United States
Presentation Overview: Show
Protein language models (PLMs) are amongst the most exciting recent advances for characterizing protein sequences, and have enabled a diverse set of applications including structure determination, functional property prediction, and mutation impact assessment, all from single protein sequences alone. State-of-the-art PLMs leverage transformer architectures originally developed for natural language processing, and are pre-trained on large protein databases to generate contextualized representations of individual amino acids. To harness the power of these PLMs to predict protein-level properties, these per-residue embeddings are typically ``pooled'' to fixed-size vectors that are further utilized in downstream prediction networks. Common pooling strategies include Cls-Pooling and Avg-Pooling, but neither of these approaches can capture the local substructures and long-range interactions observed in proteins. To address these weaknesses in existing PLM pooling strategies, we propose the use of attention pooling, which can naturally capture these important features of proteins.
To make the expensive attention operator (quadratic in length of the input protein) feasible in practice, we introduce bag-of-mer pooling (BoM-Pooling), a locality-aware hierarchical pooling technique that combines windowed average pooling with attention pooling. We empirically demonstrate that both full attention pooling and BoM-Pooling outperform previous pooling strategies on three important, diverse tasks: (1) predicting the activities of two proteins as they are varied; (2) detecting remote homologs; and (3) predicting signaling interactions with peptides. Overall, our work highlights the advantages of biologically inspired pooling techniques in protein sequence modeling and is a step towards more effective adaptations of language models in biological settings.
LoRA-DR-suite: adapted embeddings predict intrinsic and soft disorder from protein sequences
- Gianluca Lombardi, Sorbonne Université, France
- Beatriz Seoane, Universidad Complutense de Madrid, Spain
- Alessandra Carbone, Sorbonne Université, France
Presentation Overview: Show
Intrinsic disorder regions (IDR) and soft disorder regions (SDR) provide crucial information on a protein structure to underpin its functioning, interaction with other molecules and assembly path. Circular dichroism experiments are used to identify intrinsic disorder residues, while SDRs are characterized using B-factors, missing residues, or a combination of both in alternative X-ray crystal structures of the same molecule. These flexible regions in proteins are particularly significant in diverse biological processes and are often implicated in pathological conditions. Accurate computational prediction of these disordered regions is thus essential for advancing protein research and understanding their functional implications. To address this challenge, LoRA-DR-suite employs a simple adapter-based architecture that utilizes protein language models embeddings as protein sequence representations, enabling the precise prediction of IDRs and SDRs directly from primary sequence data. Alongside the fast LoRA-DR-suite implementation, we release SoftDis, a unique soft disorder database constructed for approximately 500,000 PDB chains. SoftDis is designed to facilitate new research, testing, and applications on soft disorder, advancing the study of protein dynamics and interactions.
NEAR: Neural Embeddings for Amino acid Relationships
- Daniel Olson, University of Montana, United States
- Thomas Colligan, University of Arizona, United States
- Daphne Demekas, University of Arizona, United States
- Jack Roddy, University of Arizona, United States
- Ken Youens-Clark, University of Arizona, United States
- Travis Wheeler, University of Arizona, United States
Presentation Overview: Show
Protein language models (PLMs) have recently demonstrated potential to supplant classical protein database search methods based on sequence alignment, but are slower than common alignment-based tools and appear to be prone to a high rate of false labeling.
Here, we present NEAR, a method based on neural representation learning that is designed to improve both speed and accuracy of search for likely homologs in a large protein sequence database.
NEAR’s ResNet embedding model is trained using contrastive learning guided by trusted sequence alignments. It computes per-residue embeddings for target and query protein sequences, and identifies alignment candidates with a pipeline consisting of residue-level k-NN search and a simple neighbor aggregation scheme.
Tests on a benchmark consisting of trusted remote homologs and randomly shuffled decoy sequences reveal that NEAR substantially improves accuracy relative to state-of-the-art PLMs, with lower memory requirements and faster embedding / search speed. While these results suggest that the NEAR model may be useful for standalone homology detection with increased sensitivity over standard alignment-based methods, in this manuscript we focus on a more straightforward analysis of the model's value as a high-speed pre-filter for sensitive annotation. In that context, NEAR is at least 5x faster than the pre-filter currently used in the widely-used profile hidden Markov model (pHMM) search tool, HMMER3, and also outperforms the pre-filter used in our fast pHMM tool, nail.
Recovering Time-Varying Networks From Single-Cell Data
- Euxhen Hasanaj, Carnegie Mellon University, United States
- Barnabás Póczos, Carnegie Mellon University, United States
- Ziv Bar-Joseph, Carnegie Mellon University, United States
Presentation Overview: Show
Gene regulation is a dynamic process that underlies all aspects of human development, disease response, and other key biological processes. The reconstruction of temporal gene regulatory networks has conventionally relied on regression analysis, graphical models, or other types of relevance networks. With the large increase in time series single-cell data, new approaches are needed to address the unique scale and nature of this data for reconstructing such networks. Here, we develop a deep neural network, Marlene, to infer dynamic graphs from time series single-cell gene expression data. Marlene constructs directed gene networks using a self-attention mechanism where the weights evolve over time using recurrent units. By employing meta learning, the model is able to recover accurate temporal networks even for rare cell types. In addition, Marlene can identify gene interactions relevant to specific biological responses, including COVID-19 immune response, fibrosis, and aging, paving the way for potential treatments. The code use to train Marlene is available at https://github.com/euxhenh/Marlene.
TCR-epiDiff: Solving Dual Challenges of TCR Generation and Binding Prediction
- Se Yeon Seo, Soongsil University, South Korea
- Je-Keun Rhee, Soongsil University, South Korea
Presentation Overview: Show
Motivation: T-cell receptors (TCRs) are fundamental components of the adaptive immune system, recognizing specific antigens for targeted immune responses. Understanding their sequence patterns for designing effective vaccines and immunotherapies. However, the vast diversity of TCR sequences and complex binding mechanisms pose significant challenges in generating TCRs that are specific to a particular epitope.
Results: Here, we propose TCR-epiDiff, a diffusion-based deep learning model for generating epitope-specific TCRs and predicting TCR-epitope binding. TCR-epiDiff integrates epitope information during TCR sequence embedding using ProtT5-XL and employs a denoising diffusion probabilistic model for sequence generation. Using external validation datasets, we demonstrate the ability to generate biologically plausible, epitope-specific TCRs. Furthermore, we leverage the model's encoder to develop a TCR-epitope binding predictor that shows robust performance on the external validation data. Our approach provides a comprehensive solution for both de novo generation of epitope-specific TCRs and TCR-epitope binding prediction. This capability provides valuable insights into immune diversity and has the potential to advance targeted immunotherapies.
Availability and implementation: The data and source codes for our experiments are available at https://github.com/seoseyeon/TCR-epiDiff
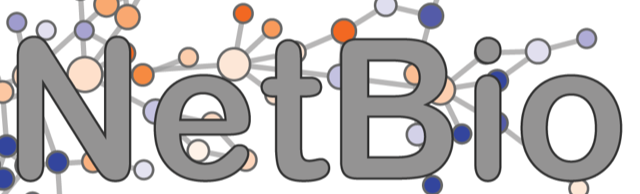
NetBio
GRACKLE: An interpretable matrix factorization approach for biomedical representation learning
- Lucas Gillenwater, University of Colorado Anschutz Medical Campus, United States
- Lawrence Hunter, University of Chicago, United States
- James Costello, University of Colorado Anschutz Medical Campus, United States
Presentation Overview: Show
Motivation: Disruption in normal gene expression can contribute to the development of diseases and chronic conditions. However, identifying disease-specific gene signatures can be challenging due to the presence of multiple co-occurring conditions and limited sample sizes. Unsupervised representation learning methods, such as matrix decomposition and deep learning, simplify high-dimensional data into understandable patterns, but often do not provide clear biological explana-tions. Incorporating prior biological knowledge directly can enhance understanding and address small sample sizes. Nevertheless, current models do not jointly consider prior knowledge of mo-lecular interactions and sample labels.
Results: We present GRACKLE, a novel non-negative matrix factorization approach that applies Graph Regularization Across Contextual KnowLedgE. GRACKLE integrates sample similarity and gene similarity matrices based on sample metadata and molecular relationships, respectively. Sim-ulation studies show GRACKLE outperformed other NMF algorithms, especially with increased background noise. GRACKLE effectively stratified breast tumor samples and identified condition-enriched subgroups in individuals with Down syndrome. The model's latent representations aligned with known biological patterns, such as autoimmune conditions and sleep apnea in Down syn-drome. GRACKLE's flexibility allows application to various data modalities, offering a robust solution for identifying context-specific molecular mechanisms in biomedical research.
Availability and implementation: GRACKLE is available at: https://github.com/lagillenwater/GRACKLE
MixingDTA: Improved Drug-Target Affinity Prediction by Extending Mixup with Guilt-By-Association
- Youngoh Kim, Seoul National University, South Korea
- Dongmin Bang, Seoul National University, South Korea
- Bonil Koo, Seoul National University, South Korea
- Jungseob Yi, Seoul National University, South Korea
- Changyun Cho, Seoul National University, South Korea
- Jeonguk Choi, Seoul National University, South Korea
- Sun Kim, Seoul National University, South Korea
Presentation Overview: Show
Drug–Target Affinity (DTA) prediction is an important regression task for drug discovery, which can provide richer information than traditional drug-target interaction prediction as a binary prediction task. To achieve accurate DTA prediction, quite large amount of data is required for each drug, which is not available as of now. Thus, data scarcity and sparsity is a major challenge. Another important task is `cold-start' DTA prediction for unseen drug or protein. In this work, we introduce MixingDTA, a novel framework to tackle data scarcity by incorporating domain-specific pre-trained language models for molecules and proteins with our MEETA (MolFormer and ESM-based Efficient aggregation Transformer for Affinity) model. We further address the label sparsity and cold-start challenges through a novel data augmentation strategy named GBA-Mixup, which interpolates embeddings of neighboring entities based on the Guilt-By-Association (GBA) principle, to improve prediction accuracy even in sparse regions of DTA space. Our experiments on benchmark datasets demonstrate that the MEETA backbone alone provides up to a 19% improvement of mean squared error over current state-of-the-art baseline, and the addition of GBA-Mixup contributes a further 8.4% improvement. Importantly, GBA-Mixup is model-agnostic, delivering performance gains across all tested backbone models of up to 16.9%. Case studies shows how MixingDTA interpolates between drugs and targets in the embedding space, demonstrating generalizability for unseen drug–target pairs while effectively focusing on functionally critical residues. These results highlight MixingDTA’s potential to accelerate drug discovery by offering accurate, scalable, and biologically informed DTA predictions. The code for MixingDTA is available at https://github.com/rokieplayer20/MixingDTA.
Prediction of Gene Regulatory Connections with Joint Single-Cell Foundation Models and Graph-Based Learning
- Sindhura Kommu, Virginia Tech, United States
- Yizhi Wang, Virginia Tech, United States
- Yue Wang, Virginia Tech, United States
- Xuan Wang, Virginia Tech, United States
Presentation Overview: Show
Motivation: Single-cell RNA sequencing (scRNA-seq) data offers unprecedented opportunities to infer gene regulatory networks (GRNs) at a fine-grained resolution, shedding light on cellular phenotypes at the molecular level. However, the high sparsity, noise, and dropout events inherent in scRNA-seq data pose significant challenges for accurate and reliable GRN inference. The rapid growth in experimentally validated transcription factor-DNA binding data has enabled supervised machine learning methods, which rely on known regulatory interactions to learn patterns, and achieve high accuracy in GRN inference by framing it as a gene regulatory link prediction task. This study addresses the gene regulatory link prediction problem by learning vectorized representations at the gene level to predict missing regulatory interactions. However, a higher performance of supervised learning methods requires a large amount of known TF-DNA binding data, which is often experimentally expensive and therefore limited in amount. Advances in large-scale pre-training and transfer learning provide a transformative opportunity to address this challenge. In this study, we leverage large-scale pre-trained models, trained on extensive scRNA-seq datasets and known as single-cell foundation models (scFMs). These models are combined with joint graph-based learning to establish a robust foundation for gene regulatory link prediction.
Results: We propose scRegNet, a novel and effective framework that leverages scFMs with joint graph-based learning for gene regulatory link prediction. scRegNet achieves state-of-the-art results in comparison with nine baseline methods on seven scRNA-seq benchmark datasets. Additionally, scRegNet is more robust than the baseline methods on noisy training data.
Availability: The source code is available at https://github.com/sindhura-cs/scRegNet
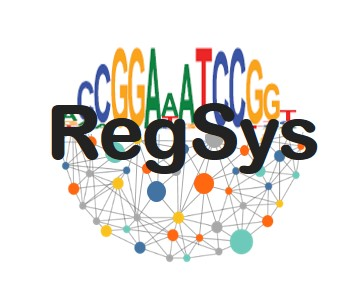
RegSys
Anomaly Detection in Spatial Transcriptomics via Spatially Localized Density Comparison
- Gary Hu, Princeton University, United States
- Julian Gold, Princeton University, United States
- Uthsav Chitra, Broad Institute of MIT and Harvard, United States
- Sunay Joshi, University of Pennsylvania, United States
- Benjamin Raphael, Princeton University, United States
Presentation Overview: Show
Motivation
Perturbations in biological tissues – e.g. due to inflammation, disease, or drug treatment – alter the composition of cell types and cell states in the tissue. These alterations are often spatially localized in different regions of a tissue, and can be measured using spatial transcriptomics technologies. However, current methods to analyze differential abundance in cell types or cell states, either do not incorporate spatial information – and thus cannot identify spatially localized alterations – or use heuristic and inaccurate approaches.
Results
We introduce Spatial Anomaly Region Detection in Expression Manifolds (Sardine), a method to estimate spatially localized changes in spatial transcriptomics data obtained from tissue slices from two or more conditions. Sardine estimates the probability of a cell state being at the same (relative) spatial location between different conditions using spatially localized density estimation. On simulated data, Sardine recapitulates the spatial patterning of expression changes more accurately than existing approaches. On a Visium dataset of the mouse cerebral cortex before and after injury response, as well as on a Visium dataset of a mouse spinal cord undergoing electrotherapy, Sardine identifies regions of spatially localized expression changes that are more biologically plausible than alternative approaches.
Detection of Cell-type-specific Differentially Methylated Regions in Epigenome-Wide Association Studies
- Ruofan Jia, The Chinese University of Hong Kong, Hong Kong
- Yingying Wei, The Chinese University of Hong Kong, Hong Kong
Presentation Overview: Show
DNA methylation at cytosine-phosphate-guanine (CpG) sites is one of the most important epigenetic markers. Therefore, epidemiologists are interested in investigating DNA methylation in large cohorts through epigenome-wide association studies (EWAS). However, the observed EWAS data are bulk data with signals aggregated from distinct cell types. Deconvolution of cell-type-specific signals from EWAS data is challenging because phenotypes can affect both cell-type proportions and cell-type-specific methylation levels. Recently, there has been active research on detecting cell-type-specific risk CpG sites for EWAS data. However, since existing methods all assume that the methylation levels of different CpG sites are independent and perform association detection for each CpG site separately, although they significantly improve the detection at the aggregated-level−identifying a CpG site as a risk CpG site as long as it is associated with the phenotype in any cell type, they have low power in detecting cell-type-specific associations for EWAS with typical sample sizes. Here, we develop a new method, Fine-scale inference for Differentially Methylated Regions (FineDMR), to borrow strengths of nearby CpG sites to improve the cell-type-specific association detection. Via a Bayesian hierarchical model built upon Gaussian process functional regression, FineDMR takes advantage of the spatial dependencies between CpG sites. FineDMR can provide cell-type-specific association detection as well as output subject-specific and cell-type-specific methylation profiles for each subject. Simulation studies and real data analysis show that FineDMR substantially improves the power in detecting cell-type-specific associations for EWAS data. FineDMR is freely available at https://github.com/JiaRuofan/Detection-of-Cell-type-specific-DMRs-in-EWAS.
GASTON-Mix: a unified model of spatial gradients and domains using spatial mixture-of-experts
- Uthsav Chitra, Princeton University, United States
- Shu Dan, Princeton University, United States
- Fenna Krienen, Princeton University, United States
- Ben Raphael, Princeton University, United States
Presentation Overview: Show
Motivation: Gene expression varies across a tissue due to both the organization of the tissue into spatial domains, i.e. discrete regions of a tissue with distinct cell type composition, and continuous spatial gradients of gene expression within different spatial domains. Spatially resolved transcriptomics (SRT) technologies provide high-throughput measurements of gene expression in a tissue slice, enabling the characterization of spatial gradients and domains. However, existing computational methods for quantifying spatial variation in gene expression either model only spatial domains – and do not account for continuous gradients of expression – or require restrictive geometric assumptions on the spatial domains and spatial gradients that do not hold for many complex tissues.
Results: We introduce GASTON-Mix, a machine learning algorithm to identify both spatial domains and spatial gradients within each domain from SRT data. GASTON-Mix extends the mixture-of-experts (MoE) deep learning framework to a spatial MoE model, combining the clustering component of the MoE model with a neural field model that learns a separate 1-D coordinate (“isodepth”) within each domain. The spatial MoE is capable of representing any geometric arrangement of spatial domains in a tissue, and the isodepth coordinates define continuous gradients of gene expression within each domain. We show using simulations and real data that GASTON-Mix identifies spatial domains and spatial gradients of gene expression more accurately than existing methods. GASTON-Mix reveals spatial gradients in the striatum and lateral septum that regulate complex social behavior, and GASTON-Mix reveals localized spatial gradients of hypoxia and TNF-$alpha$ signaling in the tumor microenvironment.
Leveraging Transcription Factor Physical Proximity for Enhancing Gene Regulation Inference
- Xiaoqing Huang, Department of Biostatistics and Health Data Science School of Medicine, Indiana University, United States
- Aamir Raza Muneer Ahemad Hullur, School of Informatics and Computing, Indiana University, Bloomington, IN 47408, United States
- Elham Jafari, INDIANA UNIVERSITY, United States
- Kaushik Shridhar, School of Informatics and Computing, Indiana University, Bloomington, IN 47408, United States
- Mu Zhou, Rutgers University, United States
- Kenneth Mackie, Indiana University Bloomington, United States
- Kun Huang, Indiana University School of Medicine, United States
- Yijie Wang, School of Informatics and Computing, Indiana University, Bloomington, IN 47408, United States
Presentation Overview: Show
Motivation: Gene regulation inference, a key challenge in systems biology, is crucial for understanding cell function, as it governs processes such as differentiation, cell state maintenance, signal transduction, and stress response. Leading methods utilize gene expression, chromatin accessibility, Transcription Factor (TF) DNA binding motifs, and prior knowledge. However, they overlook the fact that TFs must be in physical proximity to facilitate transcriptional gene regulation.
Results: To fill the gap, we develop GRIP – Gene Regulation Inference by considering TF Proximity – a gene regulation inference method that directly considers the physical proximity between regulating TFs. Specifically, we use the distance in a protein-protein interaction (PPI) network to estimate the physical proximity between TFs. We design a novel Boolean convex program, which can identify TFs that not only can explain the gene expression of target genes (TGs) but also stay close in the PPI network. We propose an efficient algorithm to solve the Boolean relaxation of the proposed model with a theoretical tightness guarantee. We compare our GRIP with state-of-the-art methods (SCENIC+, DirectNet, Pando, and CellOracle) on inferring cell-type-specific (CD4, CD8, and CD 14) gene regulation using the PBMC 3k scMultiome-seq data and demonstrate its out-performance in terms of the predictive power of the inferred TFs, the physical distance between the inferred TFs, and the agreement between the inferred gene regulation and PCHiC ground-truth data.
miRBench: novel benchmark datasets for microRNA binding site prediction that mitigate against prevalent microRNA Frequency Class Bias
- Stephanie Sammut, University of Malta, Malta
- Katarina Gresova, Masaryk University, Czechia
- Dimosthenis Tzimotoudis, University of Malta, Malta
- Eva Marsalkova, Masaryk University, Czechia
- David Cechak, Masaryk University, Czechia
- Panagiotis Alexiou, University of Malta, Malta
Presentation Overview: Show
Motivation: MicroRNAs (miRNAs) are crucial regulators of gene expression, but the precise mechanisms governing their binding to target sites remain unclear. A major contributing factor to this is the lack of unbiased experimental datasets for training accurate prediction models. While recent experimental advances have provided numerous miRNA-target interactions, these are solely positive interactions. Generating negative examples in silico is challenging and prone to introducing biases, such as the miRNA frequency class bias identified in this work. Biases within datasets can compromise model generalization, leading models to learn dataset-specific artifacts rather than true biological patterns.
Results: We introduce a novel methodology for negative sample generation that effectively mitigates the miRNA frequency class bias. Using this methodology, we curate several new, extensive datasets and benchmark several state-of-the-art methods on them. We find that a simple convolutional neural network model, retrained on some of these datasets, is able to outperform state-of-the-art methods. This highlights the potential for leveraging unbiased datasets to achieve improved performance in miRNA binding site prediction. To facilitate further research and lower the barrier to entry for machine learning researchers, we provide an easily accessible Python package, miRBench, for dataset retrieval, sequence encoding, and the execution of state-of-the-art models.
Availability: The miRBench Python Package is accessible at https://github.com/katarinagresova/miRBench/releases/tag/v1.0.0
Contact: panagiotis.alexiou@um.edu.mt
MutBERT: Probabilistic Genome Representation Improves Genomics Foundation Models
- Weicai Long, Hong Kong University of Science and Technology (Guangzhou), China
- Houcheng Su, Hong Kong University of Science and Technology (Guangzhou), China
- Jiaqi Xiong, Hong Kong University of Science and Technology (Guangzhou), China
- Yanlin Zhang, Hong Kong University of Science and Technology (Guangzhou), China
Presentation Overview: Show
Motivation: Understanding the genomic foundation of human diversity and disease requires models that effectively capture sequence variation, such as single nucleotide polymorphisms (SNPs). While recent genomic foundation models have scaled to larger datasets and multi-species inputs, they often fail to account for the sparsity and redundancy inherent in human population data, such as those in the 1000 Genomes Project. SNPs are rare in humans, and current masked language models (MLMs) trained directly on whole-genome sequences may struggle to efficiently learn these variations. Additionally, training on the entire dataset without prioritizing regions of genetic variation results in inefficiencies and negligible gains in performance.
Results: We present MutBERT, a probabilistic genome-based masked language model that efficiently utilizes SNP information from population-scale genomic data. By representing the entire genome as a probabilistic distribution over observed allele frequencies, MutBERT focuses on informative genomic variations while maintaining computational efficiency. We evaluated MutBERT against DNABERT-2, various versions of Nucleotide Transformer, and modified versions of MutBERT across multiple downstream prediction tasks. MutBERT consistently ranked as one of the top-performing models, demonstrating that this novel representation strategy enables better utilization of biobank-scale genomic data in building pretrained genomic foundation models.
Availability: https://github.com/ai4nucleome/mutBERT
Contact: yanlinzhang@hkust-gz.edu.cn
Refinement Strategies for Tangram for Reliable Single-Cell to Spatial Mapping
- Merle Stahl, Data Science in Systems Biology, TUM School of Life Sciences, Technical University of Munich, Freising, Germany, Germany
- Lena J. Straßer, Data Science in Systems Biology, TUM School of Life Sciences, Technical University of Munich, Freising, Germany, Germany
- Chit Tong Lio, Data Science in Systems Biology, TUM School of Life Sciences, Technical University of Munich, Freising, Germany, Germany
- Judith Bernett, Data Science in Systems Biology, TUM School of Life Sciences, Technical University of Munich, Freising, Germany, Germany
- Richard Röttger, Department of Mathematics and Computer Science, University of Southern Denmark, Odense, Denmark, Germany
- Markus List, Data Science in Systems Biology, TUM School of Life Sciences, Technical University of Munich, Freising, Germany, Germany
Presentation Overview: Show
Motivation: Single-cell RNA sequencing (scRNA-seq) provides comprehensive gene expression data at a
single-cell level but lacks spatial context. In contrast, spatial transcriptomics captures both spatial and
transcriptional information but is limited by resolution, sensitivity, or feasibility. No single technology combines
both the high spatial resolution and deep transcriptomic profiling at the single-cell level without trade-offs.
Spatial mapping tools that integrate scRNA-seq and spatial transcriptomics data are crucial to bridge this gap.
However, we found that Tangram, one of the most prominent spatial mapping tools, provides inconsistent
results over repeated runs.
Results: We refine Tangram to achieve more consistent cell mappings and investigate the challenges that
arise from data characteristics. We find that the mapping quality depends on the gene expression sparsity.
To address this, we (1) train the model on an informative gene subset, (2) apply cell filtering, (3) introduce
several forms of regularization, and (4) incorporate neighborhood information. Evaluations on real and
simulated mouse datasets demonstrate that this approach improves both gene expression prediction and cell
mapping. Consistent cell mapping strengthens the reliability of the projection of cell annotations and features
into space, gene imputation, and correction of low-quality measurements. Our pipeline, which includes gene
set and hyperparameter selection, can serve as guidance for applying Tangram on other datasets, while our
benchmarking framework with data simulation and inconsistency metrics is useful for evaluating other tools
or Tangram modifications.
Availability: The refinements for Tangram and our benchmarking pipeline are available at https://github.
com/daisybio/Tangram_Refinement_Strategies.
Soffritto: a deep-learning model for predicting high-resolution replication timing
- Dante Bolzan, La Jolla Institute for Immunology, United States
- Ferhat Ay, La Jolla Institute for Immunology, United States
Presentation Overview: Show
Motivation: Replication Timing (RT) refers to the order by which DNA loci are replicated during S phase. RT is cell-type specific and implicated in cellular processes including transcription, differentiation, and disease. RT is typically quantified genome-wide using two-fraction assays (e.g., Repli-Seq) which sort cells into early and late S phase fractions followed by DNA sequencing yielding a ratio as the RT signal. While two-fraction RT data is widely available in multiple cell lines, it is limited in its ability to capture high-resolution RT features. To address this, high-resolution Repli-Seq, which quantifies RT across 16 fractions, was developed, but it is costly and technically challenging with very limited data generated to date.
Results: Here we developed Soffritto, a deep learning model that predicts high-resolution RT data using two-fraction RT data, histone ChIP-seq data, GC content, and gene density as input. Soffritto is composed of a Long Short Term Memory (LSTM) module and a prediction module. The LSTM module learns long- and short-range interactions between genomic bins while the prediction module is composed of a fully connected layer that outputs a 16-fraction probability vector for each bin using the LSTM module’s embeddings as input. By performing both within cell line and cross cell line training and testing for five human and mouse cell lines, we show that Soffritto is able to capture experimental 16-fraction RT signals with high accuracy and the predicted signals allow detection of high-resolution RT patterns.
Unicorn: Enhancing Single-Cell Hi-C Data with Blind Super-Resolution for 3D Genome Structure Reconstruction
- Mohan Kumar Chandrashekar, University of Colorado,Colorado Springs, United States
- Rohit Menon, University of Colorado, Colorado Springs, United States
- Samuel Olowofila, University of Colorado, Colorado Springs, United States
- Oluwatosin Oluwadare, University of Colorado, Colorado Springs, United States
Presentation Overview: Show
Motivation: Single-cell Hi-C (scHi-C) data provide critical insights into chromatin interactions at individual cell levels, uncovering unique genomic 3D structures. However, scHi-C datasets are characterized by sparsity and noise, complicating efforts to accurately reconstruct high-resolution chromosomal structures. In this study, we present ScUnicorn, a novel blind Super-Resolution framework for scHi-C data enhancement. ScUnicorn employs an iterative degradation kernel optimization process, unlike traditional Super-resolution approaches, which rely on downsampling, predefined degradation ratios, or constant assumptions about the input data to reconstruct high-resolution interaction matrices. Hence, our approach more reliably preserves critical biological patterns and minimizes noise. Additionally, we propose 3DUnicorn, a maximum likelihood algorithm that leverages the enhanced scHi-C data to infer precise 3D chromosomal structures.
Result: Our evaluation demonstrates that ScUnicorn achieves superior performance over the state-of-the-art methods in terms of Peak Signal-to-Noise Ratio (PSNR), Structural Similarity Index Measure (SSIM), and GenomeDisco scores. Moreover, 3DUnicorn’s reconstructed structures align closely with experimental 3D-FISH data, underscoring its biological relevance. Together, ScUnicorn and 3DUnicorn provide a robust framework for advancing genomic research by enhancing scHi-C data fidelity and enabling accurate 3D genome structure reconstruction.
Code Availability: Unicorn implementation is publicly accessible at https://github.com/OluwadareLab/Unicorn
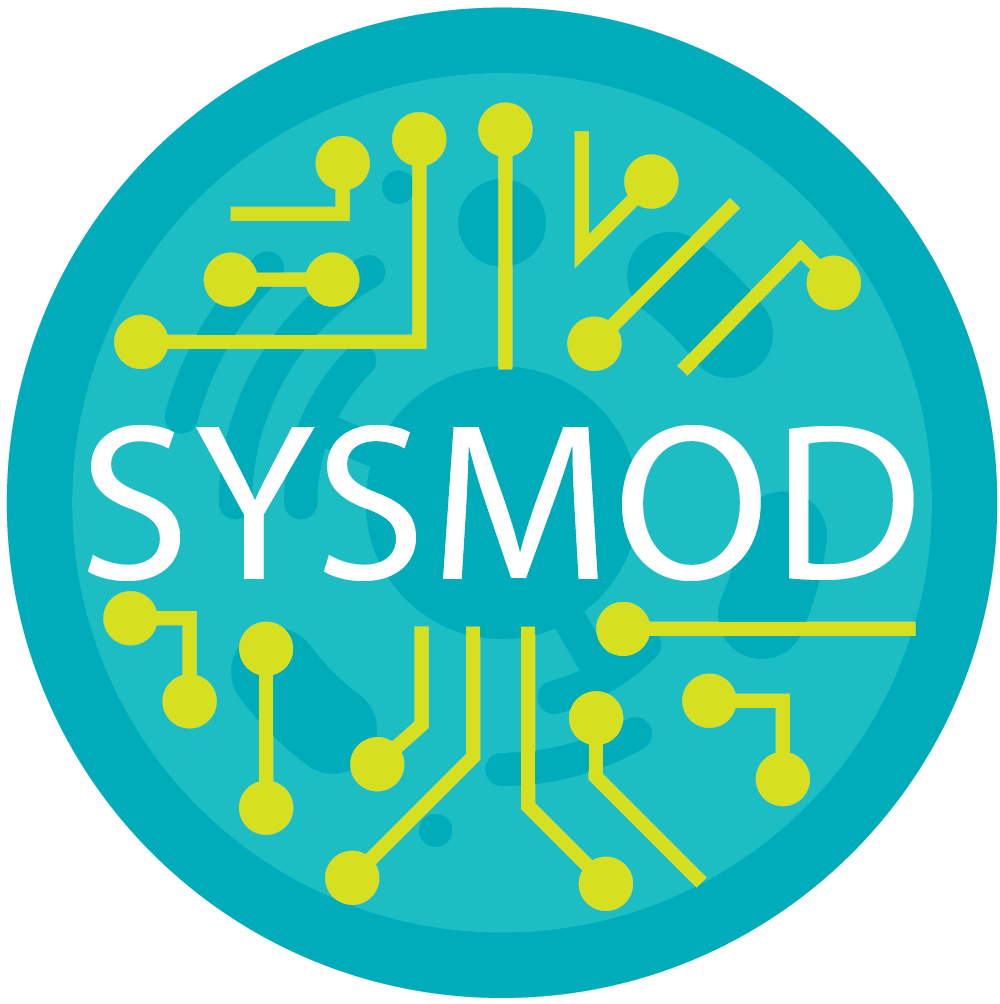
SysMod
ARTEMIS integrates autoencoders and Schrödinger Bridges to predict continuous dynamics of gene expression, cell population and perturbation from time-series single-cell data
- Sayali Anil Alatkar, University of Wisconsin-Madison, United States
- Daifeng Wang, University of Wisconsin - Madison, United States
Presentation Overview: Show
Cellular processes like development, differentiation, and disease progression are highly complex and dynamic (e.g., gene expression). These processes often undergo cell population changes driven by cell birth, proliferation, and death. Single-cell
sequencing enables gene expression measurement at the cellular resolution, allowing us to decipher cellular and molecular dynamics underlying these processes. However, the high costs and destructive nature of sequencing restrict observations to snapshots of unaligned cells at discrete timepoints, limiting our understanding of these processes and complicating the reconstruction of cellular trajectories. To address this challenge, we propose ARTEMIS, a generative model integrating a variational autoencoder (VAE) with unbalanced Diffusion Schrödinger Bridge (uDSB) to model cellular processes by reconstructing cellular trajectories, reveal gene expression dynamics, and recover cell population changes. The VAE maps input time-series single-cell data to a continuous latent space, where trajectories are reconstructed by solving the Schrödinger bridge problem using forward-backward stochastic differential equations (SDEs). A drift function in the SDEs captures deterministic gene expression trends. An additional neural network estimates time-varying kill rates for single cells along trajectories, enabling recovery of cell population changes. Using three scRNA-seq datasets—pancreatic β-cell differentiation, zebrafish embryogenesis, and epithelial-mesenchymal transition (EMT) in cancer cells—we demonstrate that ARTEMIS: (i) outperforms state-of-art methods to predict held-out timepoints, (ii) recovers relative cell population changes over time, and (iii) identifies “drift” genes driving deterministic expression trends in cell trajectories. Furthermore, in silico perturbations show that these genes influence processes like EMT. The code for ARTEMIS: https://github.com/daifengwanglab/ARTEMIS.
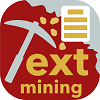
TextMining
Enhancing Biomedical Relation Extraction with Directionality
- Po-Ting Lai, National Center for Biotechnology Information, United States
- Chih-Hsuan Wei, National Center for Biotechnology Information, United States
- Shubo Tian, National Center for Biotechnology Information, United States
- Robert Leaman, National Center for Biotechnology Information, United States
- Zhiyong Lu, NCBI, United States
Presentation Overview: Show
Biological relation networks contain rich information for understanding the biological mechanisms behind the relationship of entities such as genes, proteins, diseases, and chemicals. The vast growth of biomedical literature poses significant challenges updating the network knowledge. The recent Biomedical Relation Extraction Dataset (BioRED) provides valuable manual annotations, facilitating the development of machine-learning and pre-trained language model approaches for automatically identifying novel document-level (inter-sentence context) relation-ships. Nonetheless, its annotations lack directionality (subject/object) for the entity roles, essential for studying complex biological networks. Herein we annotate the entity roles of the relationships in the BioRED corpus and subsequently propose a novel multi-task language model with soft-prompt learning to jointly identify the relationship, novel findings, and entity roles. Our results include an enriched BioRED corpus with 10,864 directionality annotations. Moreover, our proposed method outperforms existing large language models such as the state-of-the-art GPT-4 and Llama-3 on two benchmarking tasks.
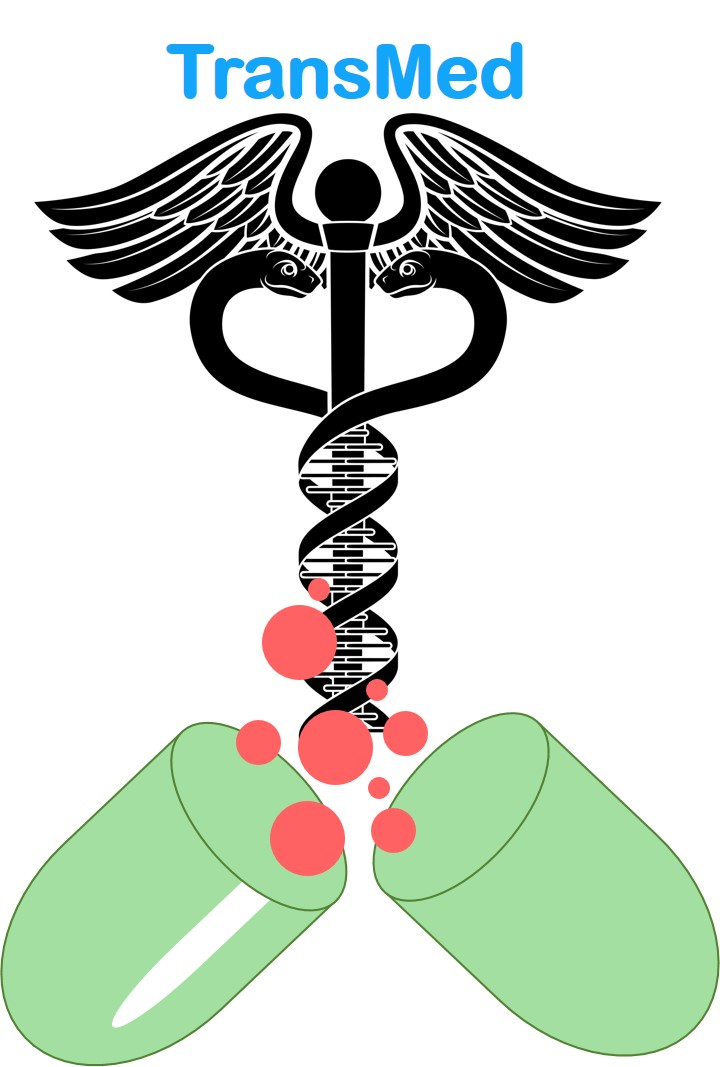
TransMed
Generating Synthetic Genotypes using Diffusion Models
- Philip Kenneweg, Bielefeld University, Germany
- Raghuram Dandinasivara, Bielefeld University, Germany
- Xiao Luo, Hunan University, China
- Barbara Hammer, Bielefeld University, Germany
- Alexander Schönhuth, Bielefeld University, Germany
Presentation Overview: Show
In this paper, we introduce the first diffusion model designed to generate complete synthetic human genotypes, which, by standard protocols, one can straightforwardly expand into full-length, DNA-level genomes.
The synthetic genotypes mimic real human genotypes without just reproducing known genotypes, in terms of approved metrics. When training biomedically relevant classifiers with synthetic genotypes, accuracy is near-identical to the accuracy achieved when training classifiers with real data. We further demonstrate that augmenting small amounts of real with synthetically generated genotypes drastically improves performance rates. This addresses a significant challenge in translational human genetics: real human genotypes, although emerging in large volumes from genome wide association studies, are sensitive private data, which limits their public availability. Therefore, the integration of additional, insensitive data when striving for rapid sharing of biomedical knowledge of public interest appears imperative.
Predicting fine-grained cell types from histology images through cross-modal learning in spatial transcriptomics
- Chaoyang Yan, Centre for Bioinformatics and Intelligent Medicine, Nankai University, China
- Zhihan Ruan, Centre for Bioinformatics and Intelligent Medicine, Nankai University, China
- Songkang Chen, Centre for Bioinformatics and Intelligent Medicine, Nankai University, China
- Yichen Pan, Centre for Bioinformatics and Intelligent Medicine, Nankai University, China
- Xue Han, Centre for Bioinformatics and Intelligent Medicine, Nankai University, China
- Yuanyu Li, Centre for Bioinformatics and Intelligent Medicine, Nankai University, China
- Jian Liu, Centre for Bioinformatics and Intelligent Medicine, Nankai University, China
Presentation Overview: Show
Motivation: Fine-grained cellular characterization provides critical insights into biological processes, including tissue development, disease progression, and treatment responses. The spatial organization of cells and the interactions among distinct cell types play a pivotal role in shaping the tumor micro-environment, driving heterogeneity, and significantly influencing patient prognosis. While computational pathology can uncover morphological structures from tissue images, conventional methods are often restricted to identifying coarse-grained and limited cell types. In contrast, spatial transcriptomics-based approaches hold promise for pinpointing fine-grained transcriptional cell types using histology data. However, these methods tend to overlook key molecular signatures inherent in gene expression data.
Results: To this end, we propose a cross-modal unified representation learning framework (CUCA) for identifying fine-grained cell types from histology images. CUCA is trained on paired morphology-molecule spatial transcriptomics data, enabling it to infer fine-grained cell types solely from pathology images. Our model aims to harness the cross-modal embedding alignment paradigm to harmonize the embedding spaces of morphological and molecular modalities, bridging the gap between image patterns and molecular expression signatures. Extensive results across three datasets show that CUCA captures molecule-enhanced cross-modal representations and improves the prediction of fine-grained transcriptional cell abundances. Downstream analyses of cellular spatial architectures and intercellular co-localization reveal that CUCA provides insights into tumor biology, offering potential advancements in cancer research.
Top-DTI: Integrating Topological Deep Learning and Large Language Models for Drug Target Interaction Prediction
- Muhammed Talo, University of North Texas, United States
- Serdar Bozdag, University of North Texas, United States
Presentation Overview: Show
Motivation: The accurate prediction of drug–target interactions (DTI) is a crucial step in drug discovery, providing a foundation for identifying novel therapeutics. Traditional drug development is both costly and time-consuming, often spanning over a decade. Computational approaches help narrow the pool of compound candidates, offering significant starting points for experimental validation. In this study, we propose Top-DTI framework for predicting DTI by integrating topological data analysis (TDA) with large language models (LLMs). Top-DTI leverages persistent homology to extract topological features from protein contact maps and drug molecular images. Simultaneously, protein and drug LLMs generate semantically rich embeddings that capture sequential and contextual information from protein sequences and drug SMILES strings. By combining these complementary features, Top-DTI enhances predictive performance and robustness.
Results: Experimental results on the public BioSNAP and Human DTI benchmark datasets demonstrate that the proposed Top-DTI model outperforms state-of-the-art approaches across multiple evaluation metrics, including AUROC, AUPRC, sensitivity, and specificity. Furthermore, the Top-DTI model achieves superior performance in the challenging cold-split scenario, where the test and validation sets contain drugs or targets absent from the training set. This setting simulates real-world scenarios and highlights the robustness of the model. Notably, incorporating topological features alongside LLM embeddings significantly improves predictive performance, underscoring the value of integrating structural and sequence-based representations.
Availability: The data and source code of Top-DTI is available at https://github.com/bozdaglab/Top DTI under Creative Commons Attribution Non-Commercial 4.0 International Public License