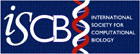
Hosted by
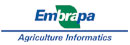
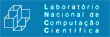
|
|
Hairpins
in a haystack: recognizing microrna precursors in
comparative genomics data
Author(s):
Jana Hertel, Bioinformatics Group, Department
of Computer Science, University of Leipzig, Germany
Peter F. Stadler, Bioinformatics Group, Department
of Computer Science, University of Leipzig, Germany;
Institute for Theoretical Chemistry, University of
Vienna, Austria; Santa Fe Institute, New Mexico, Germany
Recently, genome wide surveys for non-coding RNAs
have provided evidence for tens of thousands of
previously undescribed evolutionary conserved RNAs
with distinctive secondary structures. The annotation
of these putative ncRNAs, however, remains a difficult
problem. Here we describe an SVM-based approach
that, in conjunction with a non-stringent filter
for consensus secondary structures, is capable of
efficiently recognizing microRNA precursors in multiple
sequence alignments. The software was applied to
recent genome-wide RNAz surveys of mammals, urochordates,
and nematodes.
Keywords: miRNA, support vector
machine, non-coding RNA
Hide
Comparative
genomics reveals unusually long motifs in mammalian
genomes
Author(s):
Neil Jones, University of California San Diego,
United States
Pavel Pevzner, University of California San Diego,
United States
Motivation:
The recent discovery of the first small modulatory
RNA (smRNA) presents the challenge of finding other
molecules of similar length and conservation level.
Unlike short interfering RNA (siRNA) and micro-RNA
(miRNA), effective computational and experimental
screening methods are not currently known for this
species of RNA molecule, and the discovery of the
one known example was partly fortuitous because
it happened to be complementary to a well-studied
DNA binding motif (the Neuron Restrictive Silencer
Element).
Results:
The existing comparative genomics approaches (e.g.,
phylogenetic footprinting) rely on alignments of
orthologous regions across multiple genomes. This
approach, while extremely valuable, is not suitable
for finding motifs with highly diverged ``non-alignable''
flanking regions. Here we show that several unusually
long and well conserved motifs can be discovered
de novo through a comparative genomics approach
that does not require an alignment of orthologous
upstream regions. These motifs, including Neuron
Restrictive Silencer Element, were missed in recent
comparative genomics studies that rely on phylogenetic
footprinting. While the functions of these motifs
remain unknown, we argue that some may represent
biologically important sites.
Availability:
Our comparative genomics software, a web-accessible
database of our results and a compilation of experimentally
validated binding sites for NRSE can
be found at http://www.cse.ucsd.edu/groups/bioinformatics.
Contact: ppevzner@cs.ucsd.edu.
Hide
Relative
contributions of structural designability and functional
diversity in fixation of gene duplicates
Author(s):
Boris Shakhnovich, Boston University,
USA
Elucidation of the governing laws or even identifying
predominant trends in gene family or protein evolution
has been a formidable challenge in post-genomic
biology. While the skewed distribution of folds
and families was previously described, the key genetic
mechanisms or family specific characteristics that
influence the generation of this distribution are
as yet unknown. Furthermore, the extent of evolutionary
pressure on duplicate genes, most often credited
with generation of new genetic material and family
members is hotly debated. In this paper we present
evidence that duplicate genes have variable probability
of locus fixation correlated with strength of selection.
In turn evolutionary pressure is influenced by innate
characteristics of structural designability (e.g.
the potential for sequence entropy) of the protein
family. We further show that variability of pseudogene
formation from gene duplicates can be directly tied
to the size and designability of the family to which
the genes belong.
Hide
Automatic
clustering of orthologs and inparalogs shared by multiple
proteomes
Author(s):
Andrey Alexeyenko, Stockholm Bioinformatics Center,
Albanova, Stockholm University, Sweden
Ivica Tamas, Department of Molecular Biology &
Functional Genomics, Stockholm University, Sweden
Gang Liu, Center for Genomics and Bioinformatics,
Karolinska Institutet, Sweden
Erik Sonnhammer, Center for Genomics and Bioinformatics,
Karolinska Institutet, Sweden
The complete sequencing of many genomes has made
it possible to identify orthologous genes descending
from a common ancestor. However, reconstruction
of evolutionary history over long time periods faces
many challenges due to gene duplications and losses.
Identification of orthologous groups shared by multiple
proteomes therefore becomes a clustering problem
in which an optimal compromise between conflicting
evidences needs to be found.
Here we present a new proteome-scale analysis program
called MultiParanoid that can automatically find
orthology relationships between proteins in multiple
proteomes. The software is an extension of the InParanoid
program that identifies orthologs and inparalogs
in pairwise proteome comparisons. MultiParanoid
applies a clustering algorithm to merge multiple
pairwise ortholog groups from InParanoid into multi-species
ortholog groups. To avoid outparalogs in the same
cluster, MultiParanoid only combines species that
share the same last ancestor.
To validate the clustering technique, we compared
the results to a reference set obtained by manual
phylogenetic analysis. We further compared the results
to ortholog groups in KOGs and OrthoMCL, which revealed
that MultiParanoid produces substantially fewer
outparalogs than these resources.
MultiParanoid is a freely available standalone program
that enables efficient orthology analysis much needed
in the post-genomic era. A web-based service providing
access to the original datasets, the resulting groups
of orthologs, and the source code of the program
can be found at http://multiparanoid.cgb.ki.se.
Keywords: orthology, paralogy,
inparalog, outparalog, clustering, algorithm, last
common ancestor, comparative genomics, Homo sapiens,
C. elegans, D. melanogaster.
Hide
A
Sequence-based filtering method for ncRNA identification
and its application to searching for Riboswitch Elements
Author(s):
Shaojie Zhang, Department of Computer Science
and Engineering, University of California, San Diego,
U.S.A.
Ilya Borovok, Department of Molecular Microbiology
and Biotechnology, Tel-Aviv University, Israel
Yair Aharonowitz, Department of Molecular Microbiology
and Biotechnology, Tel-Aviv University, Israel
Roded Sharan, School of Computer Science, Tel-Aviv
University, Israel
Vineet Bafna, Department of Computer Science and
Engineering, University of California, San Diego,
U.S.A.
Recent studies have uncovered an ``RNA world'',
in which non coding RNA (ncRNA) sequences play a
central role in the regulation of gene expression.
Computational studies on ncRNA have been directed
toward developing detection methods for ncRNAs.
State-of-the-art methods for the problem, like covariance
models, suffer from high computational cost, underscoring
the need for efficient filtering approaches that
can identify promising sequence segments and accelerate
the detection process. In this paper we make several
contributions toward this goal. First, we formalize
the concept of a filter and provide figures of merit
that allow comparing between filters. Second, we
design efficient sequence based filters that dominate
the current state-of-the-art HMM filters. Third,
we provide a new formulation of the covariance model
that allows speeding up RNA alignment. We demonstrate
the power of our approach on both synthetic data
and real bacterial genomes. We then apply our algorithm
to the detection of novel riboswitch elements from
the whole bacterial and archaeal genomes. Our results
point to a number of novel riboswitch candidates,
and include genomes that were not previously known
to contain riboswitches.
Hide
Finding
novel genes in bacterial communities isolated from
the environment
Author(s):
Lutz Krause, Bielefeld University, Center for
Biotechnology (CeBiTec), Germany
Naryttza N. Diaz, Bielefeld University, Center
for Biotechnology (CeBiTec), Germany
Daniela Bartels, Bielefeld University, Center
for Biotechnology (CeBiTec) D-33594 Bielefeld
Robert A. Edwards, Fellowship for Interpretation
of Genomes, Burr Ridge IL, United States
Alfred Pühler, Universität Bielefeld,
Lehrstuhl für Genetik, Fakultät für
Biologie D-33594 Bielefeld, Germany
Forest Rohwer, Department of Biology, San Diego
State University, San Diego, CA, United States
Folker Meyer, Bielefeld University, Center for
Biotechnology (CeBiTec), Germany
Jens Stoye, Universität Bielefeld, Technische
Fakultät D-33594 Bielefeld, Germany
Motivation:
Novel sequencing techniques can give access to organisms
that are difficult to cultivate using conventional
methods. For example, the 454 pyrosequencing method
can generate a large amount of data in short time
and at a low cost. When applied to environmental
samples, the data generated has some drawbacks,
e.g. short length of assembled contigs, in-frame
stop codons and frame shifts. Unfortunately, current
gene finders can not circumvent these difficulties.
On the other hand, high throughput methods are needed
to investigate special attributes of microbial communities.
Some metagenomics analyses have already revealed
interesting findings in diversity and evolution
of complex microbial communities. Therefore, the
automated prediction of genes is a prerequisite
for the increasing amount of genomic sequences to
ensure progress in metagenomics.
Results:
We introduce a novel gene finding algorithm that
incorporates features overcoming the short length
of the assembled contigs from environmental data,
in-frame stop codons as well as frame shifts contained
in bacterial sequences. The results show that by
searching for sequence similarities in an environmental
sample our algorithm is capable of detecting a high
fraction of its gene content, depending on the species
composition and the overall size of the sample.
Therefore, the method is valuable for hunting novel
unknown genes that may be specific for the habitat
where the sample is taken. Finally, we show that
our algorithm can even exploit the limited information
contained in the short reads generated by the 454
technology for the prediction of protein coding
genes.
Hide
Top
|
|
An
experimental metagenome data management and analysis
system
Author(s):
Victor Markowitz, Biological Data Management and
Technology Center, Lawrence Berkeley National Lab,
USA
Natalia Ivanova, Genome Biology Program, Joint
Genome Institute, USA
Krishna Palaniappan, Biological Data Management
and Technology Center, Lawrence Berkeley National
Lab, USA
Ernest Szeto, Biological Data Management and Technology
Center, Lawrence Berkeley National Lab, USA
Frank Korzeniewski, Biological Data Management
and Technology Center, Lawrence Berkeley National
Lab, USA
Athanasios Lykidis, Genome Biology Program, Joint
Genome Institute, USA
Iain Anderson, Genome Biology Program, Joint Genome
Institute, USA
Konstantinos Mavrommatis, Genome Biology Program,
Joint Genome Institute, USA
Victor Kunin, Microbial Ecology Program, Joint
Genome Institute, USA
Hector Garcia Martin, Microbial Ecology Program,
Joint Genome Institute, USA
Inna Dubchak, Genomics Division, Lawrence Berkeley
National Lab, USA
Phil Hugenholtz, Microbial Ecology Program, Joint
Genome Institute, USA
Nikos Kyrpides, Genome Biology Program, Joint
Genome Institute, USA
The application of shotgun sequencing to environmental
samples has revealed a new universe of microbial
community genomes (metagenomes) involving previously
uncultured organisms. Metagenome analysis, which
is expected to provide a comprehensive picture of
the gene functions and metabolic capacity of microbial
community, needs to be conducted in the context
of a comprehensive data management and analysis
system. We present in this paper IMG/M, an experimental
metagenome data management and analysis system that
is based on the Integrated Microbial Genomes (IMG)
system. IMG/M provides tools and viewers for analyzing
both metagenomes and isolate genomes individually
or in a comparative context.
Hide
Distance
based algorithms for small biomolecule classification
and structural similarity search
Author(s):
Emre Karakoc, Simon Fraser University, Canada
Artem Cherkasov, University of British Columbia,
Canada
S. Cenk Sahinalp, Simon Fraser University, Canada
Structural similarity search among small molecules
is a standard tool used in molecular classification
and in-silico drug discovery. The effectiveness
of this general approach depends on how well the
following problems are addressed.
The notion of similarity should be chosen for providing
the highest level of discrimination of compounds
wrt the bioactivity of interest. The data structure
for performing search should be very efficient as
the molecular databases of interest include several
millions of compounds.
In this paper we focus on the k-nearest-neighbor
search method, which, until recently was not considered
for small molecule classification. The few recent
applications of k-nn to compound classification
focus on selecting the most relevant set of chemical
descriptors which are then compared under standard
Minkowski distance L_p. Here we show how to computationally
design the optimal "weighted" Minkowski
distance wL_p for maximizing the discrimination
between active and inactive compounds wrt bioactivities
of interest. We then show how to construct pruning
based k-nn search data structures for any wL_p distance
that minimizes similarity search time.
The accuracy achieved by our classifier is better
than the alternative LDA and MLR approaches and
is comparable to the ANN methods. In terms of running
time, our classifier is considerably faster than
the ANN approach especially when large data sets
are used. Furthermore, our classifier quantifies
the level of bioactivity rather than returning a
binary decision and thus is more informative than
the ANN approach.
Hide
springScape:
Visualisation of microarray and contextual bioinformatic
data using spring embedding and an information landscape
Author(s):
Timothy Ebbels, Department of Computer Science,
University College London, UK
Bernard Buxton, Department of Computer Science,
University College London, UK
David Jones, Department of Computer Science, University
College London, UK
The interpretation of microarray and other high-throughput
data is highly dependent on the biological context
of experiments. However, standard analysis packages
are poor at simultaneously presenting both the array
and related bioinformatic data. We have addressed
this challenge by developing a system springScape
based on ‘spring embedding' and an ‘information
landscape' allowing several related data sources
to be dynamically combined while highlighting one
particular feature.
Each data source is represented as a network of
nodes con-nected by weighted edges. The networks
are combined and embedded in the 2-D plane by spring
embedding such that nodes with a high similarity
are drawn close together. Complex relationships
can be discovered by varying the weight of each
data source and observing the dynamic response of
the spring network. By modifying Procrustes analysis,
we find that the visualizations have an acceptable
degree of reproducibility. The ‘information
landscape' highlights one particular data source,
displaying it as a smooth surface whose height is
proportional to both the information being viewed
and the density of nodes. The algorithm is demonstrated
using several microarray data sets in combination
with protein-protein interaction data and GO annotations.
Among the features revealed are the spatio-temporal
profile of gene expression and the identification
of GO terms correlated with gene expression and
protein interactions. The power of this combined
display lies in its interactive feedback and exploitation
of human visual pattern recognition. Overall, springScape
shows promise as a tool for the interpretation of
microarray data in the context of relevant bioinformatic
information.
Hide
SNP
Function Portal: a web database for exploring the
function implication of SNP alleles
Author(s):
Pinglang Wang, University of Michigan, United
States
Manhong Dai, University of Michigan, United States
Weijian Xuan, University of Michigan, United States
Richard C McEachin, University of Michigan, United
States
Anne U Jackson, University of Michigan, United
States
Laura J Scott, University of Michigan, United
States
Brian Athey, University of Michigan, United States
Stanley J. Watson, University of Michigan, United
States
Fan Meng, University of Michigan, United States
Motivation:
Finding the potential functional significance of
SNPs is a major bottleneck in the understanding
genome-wide SNP scanning results, as the related
functional data are distributed across many different
databases. The SNP Function Portal is designed to
be a clearing house for all public domain SNP function
annotation data, as well as in-house functional
annotations derived from data from different sources.
It currently contains SNP function annotations in
six major categories including genomic elements,
transcription regulation, protein function, pathway,
disease and population genetics. Besides extensive
SNP function annotatns, the SNP Function Portal
includes a powerful search engine that accepts different
types of genetic markers as input and identifies
all genetically related SNPs based on HapMap II
data as well as the relationship of different markers
to known genes. As a result, our system allows users
to search the potential biological impact of any
genetic marker(s), investigate complex relationships
among genetic markers and genes, and greatly facilitates
the understanding of genome-wide SNP scanning results.
Availability:
http://brainarray.mbni.med.umich.edu/Brainarray/Database/SearchSNP/snpfunc.aspx
Contact: mengf@umich.edu
Hide
Integrating
structured biological data by kernel Maximum Mean
Discrepancy
Author(s):
Karsten Borgwardt, University of Munich, Germany
Arthur Gretton, MPI Tuebingen, Germany
Malte Rasch, TU Graz, Austria
Hans-Peter Kriegel, University of Munich, Germany
Bernhard Schoelkopf, MPI Tuebingen, Germany
Alex Smola, National ICT Australia, Canberra,
Australia
Motivation:
Many problems in data integration in bioinformatics
can be posed as one common question: Are two sets
of observations generated by the same distribution?
We propose a kernel-based statistical test for this
problem, based on the fact that two distributions
are different if and only if there exists at least
one function having different expectation on the
two distributions. Consequently we use the maximum
discrepancy between function means as the basis
of a test statistic. The Maximum Mean Discrepancy
(MMD) can take advantage of the kernel trick, which
allows us to apply it not only to vectors, but strings,
sequences, graphs, and other common structured data
types arising in molecular biology.
Results:
We study the practical feasibility of an MMD-based
test on three central data integration tasks: Testing
cross-platform comparability of microarray data,
cancer diagnosis, and data-content based schema
matching for two different protein function classification
schemas. In all of these experiments, including
high-dimensional ones, MMD is very accurate in finding
samples that were generated from the same distribution,
and outperforms or is as good as its best competitors.
Conclusions:
We have defined a novel statistical test of whether
two samples are from the same distribution, compatible
with both multivariate and structured data, that
is fast, easy to implement, and works well, as confirmed
by our experiments.
Availability: http://www.dbs.ifi.lmu.de/~borgward/MMD
Hide
Top
|
|
Constructing
near-perfect phylogenies with multiple homoplasy events
Author(s):
Ravi Vijaya Satya, School of EECS, University
of central Florida Orlando FL, USA
Amar Mukherjee, School of EECS, University
of central Florida Orlando FL, USA
Gabriela Alexe, IBM T.J. Watson rsearch Center,
YorkTown Heights, NY, USA
Laxmi Parida, IBM T.J. Watson rsearch Center,
YorkTown Heights, NY, USA
Gyan Bhanot, IBM T.J. Watson rsearch Center,
YorkTown Heights, NY, USA
Motivation:
We explore the problem of constructing near-perfect
phylogenies on bi-allelic haplotypes, where the
deviation from perfect phylogeny is entirely due
to homoplasy events. We present polynomial-time
algorithms for restricted versions of the problem.
We show that these algorithms can be extended to
genotype data, in which case the problem is called
the near-perfect phylogeny haplotyping (NPPH) problem.
We present a near-optimal algorithm for the H1-NPPH
problem, which is to determine if a given set of
genotypes admit a phylogeny with a single homoplasy
event. The time-complexity of our algorithm for
the H1-NPPH problem is O(m2(n+m)), where
n is the number of genotypes and $m$ is the number
of SNP sites. This is a significant improvement
over the earlier O(n4) algorithm.
We also introduce generalized versions of the problem.
The H(1,q)-NPPH problem is to determine if a given
set of genotypes admit a phylogeny with q homoplasy
events, so that all the homoplasy events occur in
a single site. We present an O(mq+1(n+m))
algorithm for the H(1,q)-NPPH problem.
Results:
We present results on simulated data, which demonstrate
that the accuracy of our algorithm for the H1-NPPH
problem is comparable to that of the existing methods,
while being orders of magnitude faster.
Availability:
The implementation of our algorithm for the H1-NPPH
problem is available upon request.
Contact: rvijaya@cs.ucf.edu
Hide
BNTagger:
Improved tagging snp selection using bayesian networks
Author(s):
Phil Hyoun Lee, School of Computing, Queen's University,
Canada
Hagit Shatkay, School of Computing, Queen's University,
Canada
Genetic variation analysis holds much promise as
a basis for disease-gene association. However, due
to the tremendous number of candidate single nucleotide
polymorphisms (SNPs), there is a clear need to expedite
genotyping by selecting and considering only a subset
of all SNPs. This process is known as tagging SNP
selection. Several methods for tagging SNP selection
have been proposed, and have shown promising results.
However, most of them rely on strong assumptions
such as prior block-partitioning, bi-allelic SNPs,
or a fixed number or location of tagging SNPs.
We introduce BNTagger, a new method for tagging
SNP selection, based on conditional independencies
among SNPs. Using the formalism of Bayesian networks
(BNs), our system aims to select a subset of independent
and highly predictive SNPs. Similar to previous
prediction-based methods, we aim to maximize the
prediction accuracy of tagging SNPs, but unlike
them, we neither fix the number or the location
of predictive tagging SNPs, nor require SNPs to
be bi-allelic. In addition, for newly-genotyped
samples, BNTagger directly uses genotype data as
input, while producing as output haplotype data
of all SNPs.
Using three public data sets, we compare the prediction
performance of our method to that of three state-of-the-art
tagging SNP selection methods. The results demonstrate
that our method consistently improves upon previous
methods in terms of prediction accuracy. Moreover,
our method retains its good performance even when
a very small number of tagging SNPs are used.
Hide
Mutation
parameters from DNA sequence data using graph theoretic
measures on lineage trees
Author(s):
Reuma Magori Cohen, Bar Ilan University, Israel
Yoram Louzoun, Bar Ilan University, Israel
Steven Kleinstein, Princeton University, USA
Motivation:
B cells responding to antigenic stimulation can
fine-tune their binding properties through a process
of affinity maturation, composed of somatic hypermutation,
affinity-selection and clonal expansion. The mutation
rate of the B cell receptor DNA sequence, and the
effect of these mutations on affinity and specificity
are of critical importance for understanding immune
and autoimmune processes. Unbiased estimates of
these properties are currently lacking due to the
short time-scales involved and the small numbers
of sequences available.
Results:
We have developed a bioinformatic method based on
a maximum likelihood analysis of phylogenetic lineage
trees to estimate the parameters of a B cell clonal
expansion model, which includes somatic hypermutation
with the possibility of lethal mutations. Lineage
trees are created from clonally related B cell receptor
DNA sequences. Important links between tree shapes
and underlying model parameters are identified using
mutual information. Parameters are estimated using
a likelihood function based on the joint distribution
of several tree shapes, without requiring a priori
knowledge of the number of generations in the clone
(which is not available for rapidly dividing populations
in vivo). A systematic validation on synthetic trees
produced by a mutating birth-death process simulation
shows that our estimates are precise and robust
to several underlying assumptions. These methods
are applied to experimental data from autoimmune
mice to demonstrate the existence of hyper!
mutating B cells in an unexpected location in the
spleen.
Hide
Top
|
|
Predicting
the prognosis of breast cancer by integrating clinical
and microarray data with Bayesian networks
Author(s):
Olivier Gevaert, Department of Electrical Engineering
ESAT-SCD Katholieke Universiteit Leuven, Belgium
Frank De Smet, Department of Electrical Engineering
ESAT-SCD Katholieke Universiteit Leuven, Belgium
Dirk Timmerman, Department of obstetrics and gynecology,
University Hospital Gasthuisberg, Katholieke Universiteit
Leuven, Belgium
Yves Moreau, Department of Electrical Engineering
ESAT-SCD Katholieke Universiteit Leuven, Belgium
Bart De Moor, Department of Electrical Engineering
ESAT-SCD Katholieke Universiteit Leuven, Belgium
Motivation:
Clinical data, such as patient history, laboratory
analysis, ultrasound parameters - which are the
basis of day-to-day clinical decision support -
are often neglected to guide the clinical management
of cancer in the presence of microarray data. We
propose a strategy based on Bayesian networks to
treat clinical and microarray data on an equal footing.
The main advantage of this probabilistic model is
that it allows to integrate these data sources in
several ways and that it allows to investigate and
understand the model structure and parameters. Furthermore
using the concept of a Markov Blanket we can identify
all the variables that shield off the class variable
from the influence of the remaining network. Therefore
Bayesian networks automatically perform feature
selection by identifying the (in)dependency relationships
with the class variable.
Results:
We evaluated three methods for integrating clinical
and microarray data: decision integration, partial
integration and full integration and used them to
classify publicly available breast cancer patients
into a poor and a good prognosis group. The partial
integration method is most promising and has an
independent test set area under the ROC curve of
0.845. After choosing an operating point the classification
performance is better than frequently used indices.
Hide
AClAP,
Autonomous hierarchical agglomerative Cluster Analysis
based Protocol to partition conformational datasets
Author(s):
Giovanni Bottegoni, Dept. of Pharmaceutical Sciences
- University of Bologna, Italy
Walter Rocchia, NEST - Scuola Normale Superiore
of Pisa, Italy
Maurizio Recanatini, Dept. of Pharmaceutical Sciences
- University of Bologna, Italy
Andrea Cavalli, Dept. of Pharmaceutical Sciences
- University of Bologna, Italy
Motivation:
Sampling the conformational space is a fundamental
step for both ligand- and structure-based drug design.
However, the rational organization of different
molecular conformations still remains a challenge.
In fact, for drug design applications, the sampling
process provides a redundant conformation set whose
thorough analysis can be intensive, or even prohibitive.
We propose a statistical approach based on cluster
analysis aimed at rationalizing the output of methods
such as Monte Carlo, genetic, and reconstruction
algorithms. Although some software already implements
clustering procedures, at present, a universally
accepted protocol is still missing.
Results:
We integrated hierarchical agglomerative cluster
analysis with a clusterability assessment method
and a user independent cutting rule, to form a global
protocol that we implemented in a MATLAB metalanguage
program (AClAP). We tested it on the conformational
space of a quite diverse set of drugs generated
via Metropolis Monte Carlo simulation, and on the
poses we obtained by reiterated docking runs performed
by four widespread programs. In our tests, AClAP
proved to remarkably reduce the dimensionality of
the original datasets at a negligible computational
cost. Moreover, when applied to the outcomes of
many docking programs together, it was able to point
to the crystallographic pose.
Availability:
AClAP is available at the “AClAP” section
of the website http://www.scfarm.unibo.it
Contact: andrea.cavalli@unibo.it
Supplementary Information:
The complete series of AClAP results is available
in the "services” section of the website
http://www.scfarm.unibo.it.
Hide
Integrating
copy number polymorphisms into array CGH analysis
using a robust HMM
Author(s):
Sohrab Shah, University of British Columbia, Canada
Xiang Xuan, University of British Columbia, Canada
Ron DeLeeuw, British Columbia Cancer Research
Centre, Canada
Mehrnoush Khojasteh, British Columbia Cancer Research
Centre, Canada
Wan Lam, British Columbia Cancer Research Centre,
Canada
Raymond Ng, University of British Columbia, Canada
Kevin Murphy, University of British Columbia,
Canada
Array comparative genomic hybridization (aCGH)
is a pervasive technique used to identify chromosomal
aberrations in human diseases, including cancer.
Aberrations are defined as regions of increased
or decreased copy number, relative to a normal sample.
Accurately identifying the locations of these aberrations
has many important medical applications. Unfortunately,
the observed copy number changes are often corrupted
by various sources of noise, making the boundaries
hard to detect. One popular current technique uses
hidden Markov models (HMMs) to segment the signal
into regions of constant copy number; a subsequent
classification phase labels each region as a gain,
a loss or neutral. Unfortunately, standard HMMs
are sensitive to outliers, causing oversegmentation.
We propose a simple modification that makes the
HMM more robust to such single clone outliers. More
importantly, this modification allows us to exploit
prior knowledge about the likely location of such
``outliers'', which are often due to copy number
polymorphisms (CNPs). By ``explaining away'' these
outliers, we can focus attention on more interesting
aberrated regions. We show significant improvements
over the current state of the art technique (DNAcopy
with MergeLevels) on some previously used synthetic
data, augmented with outliers. We also show modest
gains on the well-studied H526 lung cancer cell
line data, and argue why we expect more substantial
gains on other data sets in the future.
Source code written in Matlab is available from
http://www.cs.ubc.ca/~sshah/acgh
Hide
Decoding
non-unique oligonucleotide hybridization experiments
of targets related by a phylogenetic tree
Author(s):
Alexander Schliep, Max Planck Institute for Molecular
Genetics, Germany
Sven Rahmann, Bielefeld University, Germany
Motivation:
The reliable identification of presence or absence
of biological agents ("targets"), such
as viruses or bacteria, is crucial for many applications
from health care to biodiversity. If genomic sequences
of targets are known, hybridization reactions between
oligonucleotide probes and targets performed on
suitable DNA microarrays will allow to infer presence
or absence from the observed pattern of hybridization.
Targets, for example all known strains of HIV, are
often closely related and finding unique probes
becomes impossible. The use of non-unique oligonucleotides
with more advanced decoding techniques from statistical
group testing allows to detect known targets with
great success. Of great relevance, however, is the
problem of identifying the presence of previously
unknown targets or of targets that evolve rapidly.
Results:
We present the first approach to decode hybridization
experiments using non-unique probes when targets
are related by a phylogenetic tree. By use of a
Bayesian framework and a Markov chain Monte Carlo
approach we are able to identify over 95% of known
targets and assign up to 70% of unknown targets
to their correct clade in hybridization simulations
on biological and simulated data.
Availability:
Software implementing the method described in this
paper and datasets are available from http://algorithmics.molgen.mpg.de/probetrees.
Keywords: virus detection, probe
design, phylogenie, MCMC
Hide
Top
|
|
DynaPred:
A structure and sequence based method for the prediction
of MHC class I binding peptide sequences and conformations
Author(s):
Iris Antes, MPI fuer Informatik, Stuhlsatzenhausweg
85, D-66123 Saarbruecken, Germany
Shirley Siu, Universitaet des Saarlandes, D-66041
Saarbruecken, Germany
Thomas Lengauer, MPI fuer Informatik, Stuhlsatzenhausweg
85, D-66123 Saarbruecken, Germany
We developed a SVM-trained, quantitative matrix-based
method for the prediction of MHC class I binding
peptides, in which the features of the scoring matrix
are energy terms retrieved from molecular dynamics
simulations. At the same time we use the equilibrated
structures obtained by the same simulations in a
simple and efficient docking procedure. Our method
consists of two steps: First, we predict potential
binders from sequence data alone and second, we
construct protein-peptide complexes for the predicted
binders. So far, we tested our approach on the HLA-A0201
allele. We constructed two prediction models, using
local, position-dependent (DynaPredPOS) and global,
position-independent (DynaPred) features. The former
model outperformed two sequence-based methods used
in the evaluation; the latter showed a slightly
lower performance (5% less accuracy), but a much
higher generalizability towards other alleles than
the position-dependent models. The constructed peptide
conformations can be refined within seconds to structures
with an average RMSD from the corresponding experimental
structures of 1.53 Å for the peptide backbone
and 1.1 Å for buried side chain atoms.
Hide
Top
|
|
A
top-level ontology of functions and its application
in the Open Biomedical Ontologies
Author(s):
Patryk Burek, University of Leipzig, Germany
Robert Hoehndorf, University of Leipzig, Max Planck
Institute for Evolutionary Anthropology, Germany
Frank Loebe, University of Leipzig, Germany
Johann Visagie, Max Planck Institute for Evolutionary
Anthropology, Germany
Heinrich Herre, University of Leipzig, Germany
Janet Kelso, Max Planck Institute for Evolutionary
Anthropology, Germany
Motivation:
A clear understanding of functions in biology is
a key component in accurate modelling of molecular,
cellular and organismal biology. Using the existing
biomedical ontologies it has been impossible to
capture the complexity of the community's knowledge
about biological functions.
Results:
We present here a top-level ontological framework
for representing knowledge about biological functions.
This framework lends greater accuracy, power and
expressiveness to biomedical ontologies by providing
a means to capture existing functional knowledge
in a more formal manner. An initial major application
of the ontology of functions is the provision of
a principled way in which to curate functional knowledge
and annotations in biomedical ontologies. Further
potential applications include the facilitation
of ontology interoperability and automated reasoning.
A major advantage of the proposed implementation
is that it is an extension to existing biomedical
ontologies, and can be applied without substantial
changes to these domain ontologies.
Availability:
The Ontology of Functions (OF) can be downloaded
in OWL format from http://onto.eva.mpg.de/.
Additionally, a UML profile and supplementary information
and guides for using the OF can be accessed from
the same website.
Contact: bioonto@lists.informatik.uni-leipzig.de
Keywords: knowledge representation,
ontology, top-level ontology, biological function
Hide
An
ontology for a robot scientist
Author(s):
Larisa Soldatova, The University of Wales, Aberystwyth,
UK
Amanda Clare, The University of Wales, Aberystwyth,
UK
Andrew Sparkes, The University of Wales, Aberystwyth,
UK
Ross King, The University of Wales, Aberystwyth,
UK
Motivation:
A Robot Scientist is a physically implemented robotic
system that can automatically carry out cycles of
scientific experimentation. We are commissioning
a new Robot Scientist designed to investigate gene
function in S. cerevisiae. This Robot Scientist
will be capable of initiating >1,000 experiments,
and making >200,000 observations a day. Robot
Scientists provide a unique test bed for the development
of methodologies for the curation and annotation
of scientific experiments: for as the experiments
are conceived and executed automatically by computer,
it is possible to completely capture and digitally
curate all aspects of the scientific process. This
new ability brings with it significant technical
challenges. To meet these we apply an ontology driven
approach to the representation of all the Robot
Scientist's data and metadata.
Results:
We demonstrate the utility of developing an ontology
for the new Robot Scientist. This ontology is based
on a general ontology of experiments. The ontology
aids the curation and annotating of: the experimental
data and metadata, the equipment metadata, and supports
the design of database systems to hold the data
and metadata.
Availability:
EXPO in XML and OWL formats is at: http://sourceforge.net/projects/expo/.
All materials about the Robot Scientist project
are available at: www.aber.ac.uk/compsci/Research/bio/robotsci/.
Hide
Protein
classification using ontology classification
Author(s):
Katherine Wolstencroft, University of Manchester,
UK
Phillip Lord, University of Newcastle, UK
Lydia Tabernero, University of Manchester, UK
Andy Brass, University of Manchester, UK
Robert Stevens, University of Manchester, UK
Motivation:
The classification of proteins expressed by an organism
is an important step in understanding the molecu-lar
biology of that organism. Traditionally, this classifica-tion
has been performed by human experts. Human knowl-edge
can recognise the functional properties that are
suffi-cient to place an individual gene product
into a particular protein family group. Automation
of this task usually fails to meet the ‘gold
standard' of the human annotator because of the
difficult recognition stage. The growing number
of genomes, the rapid changes in knowledge and the
central role of classification in the annotation
process, however, motivates the need to automate
this process.
Results:
We capture human understanding of how to rec-ognise
members of the protein phosphatases family by do-main
architecture as an ontology. By describing protein
instances in terms of the domains they contain,
it is possible to use description logic reasoners
and our ontology to as-sign those proteins to a
protein family class. We have tested our system
on classifying the protein phos-phatases of the
human and Aspergillus fumigatus genomes and found
that our knowledge-based, automatic classifica-tion
matches, and sometimes surpasses, that of the human
annotators. We have made the classification process
fast and reproducible and, where appropriate knowledge
is available, the method can potentially be generalised
for use with any protein family.
Hide
Top
|
|
Semi-Supervised
LC/MS alignment for differential proteomics
Author(s):
Bernd Fischer, ETH Zurich / Institute of Computational
Science, Switzerland
Jonas Grossmann, ETH Zurich / Institute of Plant
Biotechnology, Switzerland
Volker Roth, ETH Zurich / Institute of Computational
Science, Switzerland
Wilhelm Gruissem, ETH Zurich / Institute of Plant
Biotechnology, Switzerland
Sacha Baginsky, ETH Zurich / Institute of Plant
Biotechnology, Switzerland
Joachim M. Buhmann, ETH Zurich / Institute of
Computational Science, Switzerland
Motivation:
Mass spectrometry (MS) combined with highperformance
liquid chromatography (LC) has received considerable
attention for high-throughput analysis of the proteome.
Isotopic labeling techniques such as ICAT have been
successfully applied to derive differential quantitative
information for two protein samples, however at
the price of significantly increased complexity
of the experimental setup. To overcome these limitations,
we consider a label-free setting where correspondences
between elements of two samples have to be established
prior to the comparative analysis. The alignment
between samples is achieved by nonlinear robust
ridge regression. The correspondence estimates are
guided in a semi-supervised fashion by prior information
which is derived from sequenced tandem mass spectra.
Results:
The semi-supervised method for finding correspondences
is successfully applied to aligning highly complex
protein samples, even if they exhibit large variations
due to different experimental conditions. A large-scale
experiment clearly demonstrates that the proposed
method bridges the gap between statistical data
analysis and label-free quantitative differential
proteomics.
Keywords: Semi-Supervised Learning,
Alignment, Differential Proteomics
Hide
Annotating
proteins by mining protein interaction networks
Author(s):
Mustafa Kirac, Case Western Reserve University,
US
Gultekin Ozsoyoglu, Case Western Reserve University,
US
Jiong Yang, Case Western Reserve University, US
Motivation:
In general, most accurate gene/protein annotations
are provided by curators. Despite having lesser
evidence strengths, it is inevitable to use computational
methods for fast and a priori discovery of protein
function annotations. This paper considers the problem
of assigning Gene Ontology (GO) annotations to partially
annotated or newly discovered proteins.
Results:
We present a data mining technique that computes
the probabilistic relationships between GO annotations
of proteins on protein-protein interaction data,
and assigns highly correlated GO terms of annotated
proteins to non-annotated proteins in the target
set. In comparison with other techniques, probabilistic
suffix tree and correlation mining techniques produce
the highest prediction accuracy of 81% precision
with the recall at 45%.
Availability:
Code is available upon request. Results and used
materials are available online at http://kirac.case.edu/PROTAN
Hide
A
model-based approach for mining membrane protein crystallization
trials
Author(s):
Sitaram Asur, Department of Computer Science,
Ohio State University, USA
Srinivasan Parthasarathy, Department of Computer
Science, Ohio State University, USA
Pichai Raman, Department of Biophysics, Ohio State
University, USA
Matthew Eric Otey, Department of Computer Science,
Ohio State University, USA
Crystallization has been proven to be an essential
step in macromolecular
structure verification. Unfortunately, the bottleneck
is that the crystallization
process is quite complex. It can take any time from
weeks to years to obtain diffraction-quality crystals,
even under the right conditions. Other issues include
the time and cost involved in taking trials and
the presence of very few positive samples in a wide
and largely undetermined parameter space.
Any help in directing scientists' attention to
the hot spots in the conceptual crystallization
space would lead to increased efficiency in crystallization
trials. This work is an application case study on
mining membrane protein crystallization trials to
predict novel conditions with a high likelihood
of leading to crystallization. We use suitable supervised
learning algorithms to model the data-space and
predict a novel set of crystallization conditions.
Our preliminary wet laboratory results are very
encouraging and we believe this work shows great
promise. We conclude with a view of the crystallization
space, based on our results, which should prove
useful for future studies
in this area.
Hide
Peptide
sequence tag-based blind identification of post-translational
modification with point process model
Author(s):
Chunmei Liu, University of Georgia, USA
Bo Yan, University of Georgia, USA
Yinglei Song, University of Georgia, USA
Ying Xu, University of Georgia, USA
Liming Cai, University of Georgia, USA
An important but difficult problem in proteomics
is the identification of post-translational modifications
(PTMs) in a protein. In general, the process of
PTM identification by aligning experimental spectra
with theoretical spectra from peptides in a peptide
database is very time consuming and may lead to
high false positive rate. In this paper, we introduce
a new approach that is both efficient and effective
for blind PTM identification. Our work consists
of the following phases. First, we develop a novel
tree decomposition based algorithm that can efficiently
generate peptide sequence tags from an extended
spectrum graph. Sequence tags are selected from
all maximum weighted antisymmetric paths in the
graph and their reliabilities are evaluated with
a score function. An efficient deterministic finite
automaton (DFA) based model is then developed to
search a peptide database for candidate peptides
by using the generated sequence tags. Finally, a
point process model -- an efficient blind search
approach for PTM identification, is applied to report
the correct peptide and PTMs if there are any. Our
tests on 2657 experimental tandem mass spectra and
2620 experimental spectra with one artificially
added PTM show that, in addition to high efficiency,
our ab-initio sequence tag selection algorithm achieves
better or comparable accuracy to other approaches.
Database search results show that the sequence tags
of lengths 3 and 4 filter out more than 98.3% and
99.8% peptides respectively when applied to a yeast
peptide database. With the dramatically reduced
search space, the point process model achieves significant
improvement in accuracy as well.
Keywords: post-translational
modification (PTM), blind PTM identification, database
search, sequence tag generation, spectrum graphs,
weighted antisymmetric path, tree decomposition.
Hide
Rapid
knot detection and application to protein structure
prediction
Author(s):
Firas Khatib, Department of Biomolecular Engineering,
UCSC, USA
Matt Weirauch, Department of Biomolecular Engineering,
UCSC, USA
Carol Rohl, Department of Biomolecular Engineering,
UCSC, USA
Knots in polypeptide chains have been found in
very few proteins, and consequently should be generally
avoided in protein structure prediction methods.
Most effective structure prediction methods do not
model the protein folding process itself, but rather
seek only to correctly obtain the final native state.
Consequently, the mechanisms that prevent knots
from occurring in native proteins are not relevant
to the modeling process, and as a result, knots
may occur with significantly higher frequency in
protein models. Here we describe a simple algorithm
for knot detection that is fast enough for structure
prediction, where tens or hundreds of thousands
of conformations may be sampled during the course
of a prediction. We have used this algorithm to
characterize knots in large populations of decoy
structures generated for targets in CASP 5 and CASP
6 using the Rosetta homology-based modeling method.
Analysis of CASP5 models suggested several possible
avenues for introduction of knots into these models,
and these insights were applied to structure prediction
in CASP 6, resulting in a significant decrease in
the proportion of knotted decoys generated. Additionally,
using the knot detection algorithm on structures
in the Protein Data Bank, a previously unreported
deep trefoil knot was found in acetylornithine transcarbamolase.
Hide
A
computational approach toward label-free protein quantification
using predicted peptide detectability
Author(s):
Haixu Tang, Indiana University, USA
Randy Arnold, Indiana University, USA
Pedro Alves, Indiana University, USA
Zhiyin Xun, Indiana University, USA
David Clemmer, Indiana University, USA
Milos Novotny, Indiana University, USA
James Reilly, Indiana University, USA
Predrag Radivojac, Indiana University, USA
We propose here a new concept of peptide detectability
which could be an important factor in explaining
the relationship between a protein's quantity and
the peptides identified from it in a high-throughput
proteomics experiment. We define peptide detectability
as the probability of observing a peptide in a standard
sample analyzed by a standard proteomics routine
and argue that it is an intrinsic property of the
peptide sequence and nearby regions in the parent
protein. To test this hypothesis we first used publicly
available data and data from our own synthetic samples
in which quantities of model proteins were controlled.
We then applied machine learning approaches to demonstrate
that peptide detectability can be predicted from
its sequence and the neighboring regions in the
parent protein with satisfactory accuracy. The utility
of this approach for protein quantification is demonstrated
by peptides with higher detectability generally
being identified at lower concentrations over those
with lower detectability in the synthetic protein
mixtures. These results establish a direct link
between protein concentration and peptide detectability.
We show that, for each protein, there exists a level
of peptide detectability above which peptides are
detected and below which peptides are not detected
in an experiment. We call this level the minimum
acceptable detectability for identified peptides
(MDIP) which can be calibrated to predict protein
concentration. Triplicate analysis of a biological
sample showed that these MDIP values are consistent
among the three data sets.
Keywords: mass spectrometry, protein
quantification, peptide detectability
Hide
Top
|
|
Indel
seeds for homology search
Author(s):
Denise Mak, Boston University, USA
Yevgeniy Gelfand, Boston University, USA
Gary Benson, Boston University, USA
We are interested in detecting homologous genomic
DNA sequences with the goal of locating approximate
inverted, interspersed, and tandem repeats. Standard
search techniques start by detecting small matching
parts, called seeds, between a query sequence and
database sequences. Contiguous seed models have
existed for many years. Recently, spaced seeds were
shown to be more sensitive than contiguous seeds
without increasing the random hit rate. To determine
the superiority of one seed model over another,
a model of homologous sequence alignment must be
chosen. Previous studies evaluating spaced and contiguous
seeds have assumed that matches and mismatches occur
within these alignments, but not insertions and
deletions (indels). This is perhaps appropriate
when searching for protein coding sequences (<5%
of the human genome), but is inappropriate when
looking for repeats in the majority of genomic sequence
where indels are common. In this paper, we assume
a model of homologous sequence alignment which includes
indels and we describe a new seed model, called
indel seeds, which explicitly allows indels. We
present a waiting time formula for computing the
sensitivity of an indel seed and show that indel
seeds significantly outperform contiguous and spaced
seeds when homologies include indels. We discuss
the practical aspect of using indel seeds and finally
we present results from a search for inverted repeats
in the dog genome using both indel and spaced seeds.
Hide
Interpreting
anonymous DNA samples from mass disasters --- probabilistic
forensic inference using genetic markers
Author(s):
Tien-ho Lin, School of Computer Science, Carnegie
Mellon University, U.S.A.
Eugene W. Myers, Computer Science Division, University
of California, Berkeley, U.S.A.
Eric P. Xing, School of Computer Science, Carnegie
Mellon University, U.S.A.
Motivation:
The problem of identifying victims in a mass disaster
using DNA fingerprints involves a scale of computation
that requires efficient and accurate algorithms.
In a typical scenario there are hundreds of samples
taken from remains that must be matched to the pedigrees
of the alleged victim's surviving relatives. Moreover
the samples are often degraded due to heat and exposure.
To develop a competent method for this type of forensic
inference problem, the complicated quality issues
of DNA typing need to be handled appropriately,
the matches between every sample and every family
must be considered, and the confidence of matches
need to be provided.
Results:
We present a unified probabilistic framework that
efficiently clusters samples, conservatively eliminates
implausible sample-pedigree pairings, and handles
both degraded samples (missing values) and experimental
errors in producing and/or reading a genotype. We
present a method that confidently exclude forensically
unambiguous sample-family matches from the large
hypothesis space of candidate matches, based on
posterior inference. Due to the high confidentiality
of disaster DNA data, simulation experiments are
commonly performed and used here for validation.
The framework is shown to be robust to these errors
at levels typical in real applications. Furthermore,
the flexibility in the probabilistic models makes
it possible to extend this framework to include
other biological factors such as interdependent
markers, mitochondrial sequences, and blood type.
Hide
BaCelLo:
a balanced subcellular localization predictor
Author(s):
Andrea Pierleoni, Biocomputing Group, Dept. of
Biology, University of Bologna, Italy
Pier Luigi Martelli, Biocomputing Group, Dept.
of Biology, University of Bologna, Italy
Piero Fariselli, Biocomputing Group, Dept. of
Biology, University of Bologna, Italy
Rita Casadio, Biocomputing Group, Dept. of Biology,
University of Bologna, Italy
Motivation:
The knowledge of the subcellular localization of
a protein is fundamental for elucidating its function.
Up to date it is difficult to determine the subcellular
location for eukaryotic cells with experimental
high-throughput procedures. Computational procedures
are then needed for annotating the subcellular location
of proteins in large scale genomic projects.
Results:
BaCelLo is a predictor for five classes of subcellular
localization (secretory way, cytoplasm, nucleus,
mitochondrion and chloroplast) and it is based on
different SVMs organized in a decision tree. The
system exploits the information deriving from the
residue sequence and from alignment profiles. It
analyzes the whole sequence composition and the
compositions of both the N- and C-termini. The training
set is curated in order to avoid redundancy. For
the first time a balancing procedure is introduced
in order to mitigate the effect of biased training
sets. Three kingdom-specific predictors are implemented:
for animals, plants and fungi, respectively. When
distributing the proteins from animals and fungi
into four classes, the performances of BaCelLo reach
74% and 76%, respectively; a score of 67% is obtained
when proteins from plants are distributed into five
classes. BaCelLo outperforms the other presently
available methods for the same task and gives more
balanced accuracy and coverage values for each class.
We also predict the subcellular localization of
five proteomes, Homo sapiens, Mus musculus, Caenorhabditis
elegans, Saccharomyces cerevisiae and Arabidopsis
thaliana, comparing the protein content in each
different compartment.
Availability:
BaCelLo can be accessed at http://www.biocomp.unibo.it/bacello/
Hide
On
counting position weight matrix matches in a sequence,
with application to discriminative motif finding
Author(s):
Saurabh Sinha , University of Illinois, United
States
The position weight matrix (PWM) is a popular method
to model transcription factor binding sites. A fundamental
problem in cis-regulatory analysis is to ``count''
the occurrences of a PWM in a DNA sequence. We propose
a novel probabilistic score to solve this problem
of counting PWM occurrences. The proposed score
has two important properties: (1) It gives appropriate
weights to both strong and weak occurrences of the
PWM, without using thresholds. (2) For any given
PWM, this score can be computed while allowing for
occurrences of other, a priori known PWMs, in a
statistically sound framework. Additionally, the
score is efficiently differentiable with respect
to the PWM parameters, which has important consequences
for designing search algorithms.
The second problem we address is to find, ab initio,
PWMs that have high counts in one set of sequences,
and low counts in another. We develop a novel algorithm
to solve this ``discriminative motif-finding problem'',
using the proposed score for counting a PWM in the
sequences. The algorithm is a local search technique
that exploits derivative information on an objective
function to enhance speed and performance. It is
extensively tested on synthetic data, and shown
to perform better than a discriminative and a non-discriminative
PWM finding algorithm. It is then applied to cis-regulatory
modules involved in development of the fruitfly
embryo, to elicit known and novel motifs. We finally
use the algorithm on genes predictive of social
behavior in the honey bee, and find interesting
motifs.
Hide
Finding
regulatory motifs with maximum density subgraph
Author(s):
Eugene Fratkin, Stanford University, USA
Brian Naughton, Stanford University, USA
Douglas Brutlag, Stanford University, USA
Serafim Botzoglou, Stanford University, USA
Motivation:
DNA motif finding is an important problem in computational
biology, for which several probabilistic and discrete
approaches have been developed. Most existing methods
formulate motif finding as an intractable optimization
problem and rely either on expectation maximization
(EM) or on local heuristic searches. Another challenge
is the choice of motif model: simpler models such
as the position-specific scoring matrix (PSSM) impose
biologically unrealistic assumptions such as independence
of the motif positions, while more involved models
are harder to parametrize and learn.
Results:
We present MotifCut, a non-parametric, graph-theoretic
approach to motif finding leading to a convex optimization
problem with a polynomial time solution. We build
a graph where the vertices represent all k-mers
in the input sequences, and edges represent pairwise
k-mer similarity. In this graph, we search for a
motif as the maximum density subgraph, which is
a set of k-mers that exhibit a large number of pairwise
similarities. Our formulation does not make biological
assumptions regarding the structure of the motif.
We benchmark MotifCut on both synthetic and real
yeast motifs, and find that it compares favorably
to existing popular methods. The ability of MotifCut
to detect motifs appears to scale well with increasing
input size. Moreover, the motifs we discover are
different from those discovered by the other methods.
Hide
Apples
to apples: improving the performance of motif finders
and their significance analysis in the Twilight Zone
Author(s):
Patrick Ng, Computer Science Department, Cornell
University, USA
Niranjan Nagarajan, Computer Science Department,
Cornell University, USA
Neil Jones, Department of Computer Science and
Engineering, University of Califronia San Diego, USA
Uri Keich, Computer Science Department, Cornell
University, USA
Motivation:
Effective algorithms for finding relatively weak
motifs are an important practical necessity while
scanning long DNA sequences for regulatory elements.
The success of such an algorithm hinges on the ability
of its scoring function combined with a significance
analysis test to discern real motifs from random
noise.
Results:
In the first half of the paper we show that the
paradigm of relying on entropy scores and their
E-values can lead to undesirable results when searching
for weak motifs and we offer alternate approaches
to analyzing the significance of these motifs. In
the second half of the paper we reintroduce a scoring
function and present a motif-finder
that optimizes it that are more effective in finding
relatively weak motifs than other tools.
Availability:
The GibbsILR motif finder is available at http://www.cs.cornell.edu/~keich
Contact: Uri Keich, keich@cs.cornell.edu
Hide
CONTRAfold:
RNA secondary structure prediction without physics-based
models
Author(s):
Chuong Do, Stanford University, USA
Daniel Woods, Stanford University, USA
Serafim Batzoglou, Stanford University, USA
For several decades, free energy minimization methods
have been the dominant strategy for single-sequence
RNA secondary structure prediction. More recently,
stochastic context-free grammars (SCFGs) have emerged
as an alternative probabilistic methodology for
modeling RNA structure. Unlike physics-based methods,
which rely on thousands of experimentally-measured
thermodynamic parameters, SCFGs use fully-automated
statistical learning algorithms to derive model
parameters. Despite this advantage, however, probabilistic
methods have not replaced free energy minimization
methods as the tool of choice for secondary structure
prediction, as the accuracies of the best current
SCFGs have yet to match those of the best physics-based
models.
In this paper, we present CONTRAfold, a novel secondary
structure prediction method based on conditional
log-linear models (CLLMs), a flexible class of probabilistic
models which generalize upon SCFGs by using discriminative
training and feature-rich scoring models. In a series
of cross-validation experiments, we show that grammar-based
secondary structured prediction methods formulated
as CLLMs consistently outperform their SCFG analogs.
Furthermore, CONTRAfold, a CLLM incorporating most
of the features found in typical thermodynamic models,
achieves the highest single-sequence prediction
accuracies to date, outperforming currently available
probabilistic and physics-based techniques. Our
result thus closes the gap between probabilistic
and thermodynamic models, demonstrating that statistical
learning procedures provide an effective alternative
to empirical measurement of thermodynamic parameters
for RNA secondary structure prediction.
Hide
Context-specific
independence mixture modeling for positional weight
matrices
Author(s):
Benjamin Georgi, Max-Planck Institute for Molecular
Genetics, Germany
Alexander Schliep, Max-Planck Institute for Molecular
Genetics, Germany
Motivation:
A positional weight matrix (PWM) is a statistical
representation of the binding pattern of a transcription
factor estimated from known binding site sequences.
Previous studies showed that for factors which bind
to divergent binding sites, mixtures of multiple
PWMs increase performance. However, estimating a
conventional mixture distribution for each position
will in many cases cause overfitting.
Results:
We propose a context-specific independence (CSI)
mixture model and a learning algorithm based on
a Bayesian approach. The CSI model adjusts complexity
to fit the amount of variation observed on the sequence
level in each position of a site. This not only
yields a more parsimonious description of binding
patterns, which improves parameter estimates, it
also increases robustness as the model automatically
adapts the number of components to fit the data.
Evaluation of the CSI model on simulated data showed
favorable results compared to conventional mixtures.
We demonstrate its adaptive properties in a classical
model selection setup. The increased parsimony of
the CSI model was shown for the transcription factor
Leu3 where two binding-energy subgroups were distinguished
equally well as with a conventional mixture but
requiring 30% less parameters. Analysis of the human-mouse
conservation of predicted binding sites of 64 JASPAR
TFs showed that CSI was as good or better than a
conventional mixture for 89% of the TFs and for
70% for a single PWM model.
Availability:
http://algorithmics.molgen.mpg.de/mixture
Contact: georgi@molgen.mpg.de;
schliep@molgen.mpg.de
Hide
ARTS:
Accurate recognition of transcription starts in human
Author(s):
Soeren Sonnenburg, Fraunhofer Institute FIRST,
Germany
Alexander Zien, Max Planck Institute for Biological
Cybernetics, Germany
Gunnar Raetsch, Friedrich Miescher Laboratory
of the Max Planck Society, Germany
We develop new methods for finding transcription
start sites (TSS) of RNA Polymerase II binding genes
in genomic DNA sequences. Employing Support Vector
Machines with advanced sequence kernels, we achieve
drastically higher prediction accuracies than state-of-the-art
methods.
Motivation:
One of the most important features of genomic DNA
are the genes that encode proteins. While it is
of great value to identify those genes and the encoded
proteins, it is also crucial to understand how their
transcription is regulated. To this end one has
to identify the corresponding promoters and the
contained transcription factor binding
sites. TSS finders can be used to locate potential
promoters. They may also be used in combination
with other signal and content detectors to resolve
entire gene structures.
Results:
We have developed a novel kernel based method -
called ARTS -
that accurately recognizes transcription start sites
in human. The application of otherwise too computationally
expensive Support Vector Machines was made possible
due to the use of efficient training and evaluation
techniques using suffix tries. In a carefully designed
experimental study, we compare our TSS finder to
state-of-the-art
methods from the literature: McPromoter, Eponine
and FirstEF. For given false positive rates within
a reasonable range, we consistently achieve considerably
higher true positive rates. For instance, ARTS finds
about 24% true positives at a false positive rate
of 1/1000, where the other methods find less than
half (10.5%).
Hide
Informative
priors based on transcription factor structural class
improve de novo motif discovery
Author(s):
Leelavati Narlikar, Duke University, USA
Raluca Gordan, Duke University, USA
Uwe Ohler, Duke University, USA
Alexander Hartemink, Duke University, USA
An important problem in molecular biology is to
identify the locations at which a transcription
factor (TF) binds to DNA, given a set of DNA sequences
believed to be bound by that TF. In previous work,
we showed that information in the DNA sequence of
a binding site is alone sufficient to predict the
structural class of the TF that binds it. In particular,
this suggests that we can predict which locations
in any DNA sequence are more likely to be bound
by certain classes of TFs than others. Here, we
argue that traditional methods for de novo motif
finding can be significantly improved by adopting
an informative prior probability that a TF binding
site occurs at each sequence location. To demonstrate
the utility of such an approach, we present PRIORITY,
a powerful new de novo motif finding algorithm based
on a Gibbs sampling strategy. Using data from TRANSFAC,
we train three classifiers to recognize binding
sites of basic leucine zipper, forkhead, and basic
helix loop helix proteins. These classifiers are
used to equip PRIORITY with three class-specific
priors, in addition to a default prior to handle
TFs of other classes. We apply PRIORITY and a number
of popular motif finding programs to sets of yeast
intergenic regions that are reported by ChIP-chip
to be bound by particular TFs. PRIORITY identifies
motifs the other methods fail to identify, and correctly
predicts the structural class of the TF binding
to the identified binding sites.
Hide
ProfilePSTMM:
capturing tree-structure motifs in carbohydrate sugar
chains
Author(s):
Kiyoko Aoki-Kinoshita, Bioinformatics Center,
Institute for Chemical Research, Kyoto University,
Japan
Nobuhisa Ueda, Bioinformatics Center, Institute
for Chemical Research, Kyoto University, Japan
Hiroshi Mamitsuka, Bioinformatics Center, Institute
for Chemical Research, Kyoto University, Japan
Minoru Kanehisa, Bioinformatics Center, Institute
for Chemical Research, Kyoto University, Japan
Carbohydrate sugar chains, or glycans, are considered
the third major class of biomolecules after DNA
and proteins. They consist of branching monosaccharides,
starting from a single monosaccharide. They are
extremely vital to the development and functioning
of multicellular organisms because they are recognized
by various proteins to allow them to perform specific
functions. Our motivation is to study this recognition
mechanism using informatics techniques from the
data available. Previously, we introduced a probabilistic
sibling-dependent tree Markov model (PSTMM), which
we showed could be efficiently trained on sibling-dependent
tree structures and return the most likely state
paths. However, it had some limitations in that
the extra dependency between siblings caused overfitting
problems. The retrieval of the patterns from the
trained model also involved manually extracting
the patterns from most likely state paths. Thus
we introduce a profilePSTMM model which avoids these
problems, incorporating a novel concept of different
types of state transitions to handle parent-child
and sibling dependencies differently. Our new algorithms
are also more efficient and able to extract the
patterns more easily. We tested the profilePSTMM
model on both synthetic (controlled) data as well
as glycan data from the KEGG GLYCAN database. Additionally,
we tested it on glycans which are known to be recognized
and bound to proteins at various binding affinities,
and we show that our results correlate with results
published in the literature.
Hide
Top
|
|
ZPRED:
Predicting the distance to the membrane center for
residues in alpha-helical membrane proteins
Author(s):
Erik Granseth, Stockholm Bioinformatics Center,
Sweden
Håkan Viklund, Stockholm Bioinformatics
Center, Sweden
Arne Elofsson, Stockholm Bioinformatics Center,
Sweden
Prediction methods are of great importance for
membrane proteins as experimental information is
harder to obtain than for globular proteins. Methods
to predict the topology of membrane proteins have
reached an accuracy of 66%. As more membrane protein
structures are solved it is clear that topology
information provides a simplified picture of a membrane
protein. The proteins also contain secondary structure
elements such as re-entrant helices and interface
helices.
Here, we describe a novel challenge for the prediction
of alpha-helical membrane proteins: to predict the
distance from the center of the membrane to a residue,
a measure we define as the Z-coordinate. Even though
the traditional way of depicting membrane protein
topology is useful, it is advantageous to have a
measure that is based on a more "physical"
property such as the Z-coordinate, since it implicitly
contains information about re-entrant helices, interfacial
helices, the tilt of a transmembrane helix and loop
lengths.
We show that the Z-coordinate can be predicted
using either artificial neural networks, hidden
Markov models or combinations of both. The best
method, ZPRED, uses the output from a hidden Markov
model together with a neural network. The average
absolute error of ZPRED is 2.55Å and 68.6%
of the residues are predicted within 3Å of
the target Z-coordinate. ZPRED is also able to predict
the maximum protrusion of a loop to within 3Å
for 78% of the loops in the dataset.
Hide
A
combinatorial pattern discovery approach for the prediction
of membrane dipping (re-entrant) loops
Author(s):
Gorka Lasso, University of Swansea, United Kingdom
John Antoniw, Rothamsted Research, United Kingdom
Jonathan Mullins, University of Swansea, United
Kingdom
Membrane dipping loops are sections of membrane
proteins that reside in the membrane but do not
traverse from one side to the other, rather they
enter and leave the same side of the membrane. We
applied a combinatorial pattern discovery approach
to sets of sequences containing at least one characterised
structure described as possessing a membrane dipping
loop. Discovered patterns were found to be composed
of residues whose biochemical role is known to be
essential for function of the protein, thus validating
our approach.
TMLOOP (http://membraneproteins.swan.ac.uk/TMLOOP)
was implemented to predict membrane dipping loops
in polytopic membrane proteins. TMLOOP applies discovered
patterns as weighted predictive rules in a collective
motif method (a variation of the single motif method),
to avoid inherent limitations of single motif methods
in detecting distantly related proteins. The collective
motif method applies several, partially overlapping
patterns, which pertain to the same sequence region,
allowing proteins containing small variations to
be detected. The approach achieved 92.4% accuracy
in sensitivity and 100% reliability in specificity.
TMLOOP was applied to the Swiss-Prot database, identifying
1392 confirmed membrane dipping loops, 75 plausible
membrane dipping loops hitherto uncharacterised
by topology prediction methods or experimental approaches
and 128 false positives (8.0%).
Hide
Comparative
footprinting of DNA-binding proteins
Author(s):
Bruno Contreras-Moreira, Centro de Ciencias Genómicas,
Universidad Nacional Autónoma de México,
México
Julio Collado-Vides, Centro de Ciencias Genómicas,
Universidad Nacional Autónoma de México,
México
Comparative modelling is a technology used to tackle
a variety of problems in molecular biology and biotechnology.
Traditionally it has been applied to model the structure
of proteins on their own or bound to small ligands,
although more recently it has also been used to
model protein-protein interfaces. This work is the
first to systematically analyze whether comparative
models of protein-DNA complexes could be built and
be useful for predicting DNA binding sites. First,
we describe the structural and evolutionary conservation
of protein-DNA interfaces, and the limits they impose
on modelling accuracy. Second, we find that side-chains
from contacting residues can be reasonably modelled
with SCWRL and therefore used to identify contacting
nucleotides. Third, the DNASITE protocol is implemented
and different parameters are benchmarked on a set
of 85 regulators from Escherichia coli. Results
show that comparative footprinting can make useful
predictions based solely on structural data, depending
primarily on the % of interface identity with respect
to the template used.
Hide
The
iRMSD: A local measure of sequence alignment accuracy
using structural information
Author(s):
Fabrice Armougom, Centre National de la Recherche
Scientifique, France
Sebastien Moretti, Centre National de la Recherche
Scientifique, France
Vladimir Keduas, Centre National de la Recherche
Scientifique, France
Cedric Notredame, Centre National de la Recherche
Scientifique, France
We introduce the iRMSD, a new type of RMSD, independent
from any structure superposition and suitable for
evaluating sequence alignments of proteins with
known structures. We demonstrate that the iRMSD
is equivalent to the standard RMSD although much
simpler to compute and we also show that it is suitable
for comparing sequence alignments and benchmarking
multiple sequence alignment methods. We tested the
iRMSD score on 6 established multiple sequence alignment
packages and found the results to be consistent
with those obtained using an established reference
alignment collection like Prefab. The iRMSD is part
of the T-Coffee package and is distributed as an
open source freeware (http://www.tcoffee.org/).
Hide
Improved
pruning algorithms and divide-and-conquer strategies
for dead-end elimination, with application to protein
design
Author(s):
Ivelin Georgiev, Dartmouth Computer Science Department,
USA
Ryan Lilien, Dartmouth Computer Science Department,
USA
Bruce Donald, Dartmouth Computer Science Department,
USA
Structure-based protein redesign can help engineer
proteins with desired novel function. Improving
computational efficiency while still maintaining
the accuracy of the design predictions has been
a major goal for protein design algorithms. The
combinatorial nature of protein design results both
from allowing residue mutations and from the incorporation
of protein side-chain flexibility. Under the assumption
that a single conformation can model protein folding
and binding, the goal of many algorithms is the
identification of the Global Minimum Energy Conformation
(GMEC). A dominant theorem for the identification
of the GMEC is Dead-End Elimination (DEE). DEE-based
algorithms have proven capable of eliminating the
majority of candidate conformations, while guaranteeing
that only rotamers not belonging to the GMEC are
pruned. However, when the protein design process
incorporates rotameric energy minimization, DEE
is no longer provably-accurate. Hence, with energy
minimization, the minimized-DEE (MinDEE) criterion
must be used instead. In this paper, we present
provably-accurate improvements to both the DEE and
MinDEE criteria. We show that our novel enhancements
result in a speedup of up to a factor of more than
1000 when applied in redesign for three different
proteins: Gramicidin Synthetase A, plastocyanin,
and protein G.
Hide
Modelling
sequential protein folding under kinetic control using
hp lattice models
Author(s):
Fabien Huard, Macquarie University, Australia
Charlotte Deane, University of Oxford, UK
Graham Wood, Macquarie University, Australia
This study presents a novel investigation of the
effect of kinetic control on cotranslational protein
folding. We demonstrate the effect using simple
HP lattice models and show that the cotranslational
folding of proteins under kinetic control has a
significant effect on the final conformation. Differences
arise because nature is not always capable of pushing
a partially folded protein back over a large energy
barrier. For this reason we argue that such constraints
should be incorporated into structure prediction
techniques. We introduce a finite surmountable energy
barrier which allows partially formed chains to
partly unfold, and permits us to enumerate exhaustively
all energy pathways. We compare the ground states
obtained sequentially with the global ground states
of designing sequences (those with a unique ground
state). We find that the sequential ground states
become less numerous and more compact as the surmountable
energy barrier increases. We also introduce a probabilistic
model to describe the distribution of final folds.
We know that the biologically active state of some
proteins (e.g. the recombinant mouse prion protein)
is not the one of lowest energy. Thus we allow partial
settling to the Boltzmann distribution of states
at each stage. Partial settling may occur for two
reasons: first, use of common codons accelerates
translation and second, the ribosome physically
re-stricts the folding space available. As a result,
conformations with the highest probability of final
occurrence are not necessarily the ones of lowest
energy.
Keywords: cotranslational fold,
sequentiality, kinetic control, surmountable energy
barrier
Hide
A
probabilistic approach to protein backbone tracing
in electron density maps
Author(s):
Frank DiMaio, UW-Madison, USA
Jude Shavlik, UW-Madison, USA
George Phillips, UW-Madison, USA
One particularly time-consuming step in protein
crystallography is interpreting the electron density
map; that is, fitting a complete molecular model
of the protein into a 3D image of the protein produced
by the crystallographic process. In poor-quality
electron density maps, the interpretation may require
a significant amount of a crystallographer's time.
Our work investigates automating the time-consuming
initial backbone trace in poor-quality density maps.
We describe ACMI (Automatic Crystallographic Map
Interpreter), which uses a probabilistic model known
as a Markov field to represent the protein. Residues
of the protein are modeled as nodes in a graph,
while edges model pairwise structural interactions.
Modeling the protein in this manner allows the model
to be flexible, considering an almost infinite number
of possible conformations, while rejecting any physically
impossible conformations. Using an efficient algorithm
for approximate inference (belief propagation) allows
the most probable trace of the protein's backbone
through the density map to be determined. We test
ACMI on a set of eight protein density maps (at
2.5 to 4.0 Å resolution), and compare our
results to alternative approaches. At these resolutions,
ACMI offers a more accurate backbone trace than
current approaches.
Hide
Learning
MHC binding
Author(s):
N. Jojic, Microsoft, USA
M. Reyes-Gomez
D. Heckerman
C. Kadie
O. Furman-Schueler
Motivated by the ability of a simple threading
approach to predict MHC-peptide binding, we developed
a new and improved structure-based model for which
parameters can be estimated from additional sources
of data about MHC-peptide binding. In addition to
the known 3D structures of a small number of MHC-peptide
complexes that were used in the original threading
approach, we included three other sources of information
on peptide-MHC binding: (1) MHC class I sequences;
(2) known binding energies for a large number of
MHC-peptide complexes; and (3) an even larger binary
dataset that contains information about strong binders
(epitopes) and non-binders (peptides that have a
low affinity for a particular MHC molecule).
Our model significantly outperforms the standard
threading approach in binding energy prediction.
We used the resulting binding energy predictor to
study viral infections in 246 HIV patients from
the West Australian cohort, and over 1000 sequences
in HIV clade B from Los Alamos National Laboratory
database, capturing the course of HIV evolution
over the last 20 years. Finally, we illustrate short-,
medium-, and long-term adaptation of HIV to the
human immune system.
Hide
Top
|
|
Create
and assess protein networks through molecular characteristics
of individual proteins
Author(s):
Yanay Ofran, Columbia University, USA
Guy Yachdav, Columbia University, USA
Eyal Mozes, Columbia University, USA
Ta-tsen Soong, Columbia University, USA
Rajesh Nair, Columbia University, USA
Burkhard Rost, Columbia University, USA
Motivation:
The study of biological systems, pathways and processes
relies increasingly on the analysis of networks.
Most often, such analyses focus predominantly on
network topology, thereby treating all proteins
or genes as identical, featureless nodes. Integrating
molecular data and insights regarding the qualities
of individual proteins into the analysis may enhance
our ability to decipher biological pathways and
processes.
Results:
Here we introduce a novel platform for data integration
that generates networks on the macro system-level,
analyzes the molecular characteristics of each protein
on the micro level, and then combines the two levels
by using the molecular characteristics to assess
the network. It also annotates the fucntion and
sub cellular localization of each protein and displays
the process on an image of a cell, rendering each
protein in its respective cellular compartment.
By thus visualizing the network in a cellular context
we are able to analyze pathways and processes in
a better way. As an example, we use the system to
analyze proteins implicated in Alzheimer's disease
and show how this integrated view corroborates previous
observations and helps formulate new hypotheses
regarding the molecular underpinnings of the disease.
Hide
Dense
subgraph computation via stochastic search: application
to detect transcriptional modules
Author(s):
Logan Everett, University of Pennsylvania, USA
Li-San Wang, University of Pennsylvania, USA
Sridhar Hannenhalli, University of Pennsylvania,
USA
Motivation:
In a tri-partite biological network of transcription
factors, their putative target genes and the tissues
in which the target genes are differentially expressed,
a tightly inter-connected (dense) subgraph may reveal
knowledge about tissue specific transcription regulation
mediated by a specific set of transcription factors,
ie., a tissue-specific transcriptional module. This
is just one context in which an efficient computation
of dense subgraphs is required.
Result:
Here we report a generic stochastic search based
method to compute dense subgraphs in a graph with
an arbitrary number of partitions and an arbitrary
connectivity among the partitions. We then use the
tool to explore tissue-specific transcriptional
regulation in the human genome. We validate our
findings in Skeletal muscle based on literature.
We could accurately deduce biological processes
for transcription factors via the tri-partite clusters
of transcription factors, genes and the functional
annotation of genes. Additionally, we propose a
few previously unknown TF-pathway associations and
tissue-specific roles for certain pathways. Finally,
our combined analysis of Cardiac, Skeletal, and
Smooth muscle data recapitulates the evolutionary
relationship among the three tissues.
Hide
A
decompositional approach to parameter estimation in
pathway modeling: A case study of the Akt and MAPK
pathways and their crosstalk
Author(s):
Geoffrey Koh, Department of Computer Science,
National University of Singapore, Singapore
Huey Fern Carol Teong, Department of Biochemistry,
National University of Singapore, Singapore
Marie-Veronique Clement, Department of Biochemistry,
National University of Singapore, Singapore
David Hsu, Department of Computer Science, National
University of Singapore, Singapore
P S Thiagarajan, Department of Computer Science,
National University of Singapore, Singapore
Parameter estimation is a critical problem in modeling
biological pathways. It is often difficult because
of the large number of parameters to be estimated
and the limited experimental data available. In
this paper, we propose a decompositional approach
to parameter estimation. It exploits the structure
of a large pathway model to break it into smaller
components, whose parameters can then be estimated
independently. This leads to significant improvement
in computational efficiency. We present our approach
in the context of Hybrid Functional Petri Net modeling
and evolutionary search for parameter value optimization.
However, the approach can be easily extended to
other modeling frameworks and is independent of
the search method used. We have tested our approach
on a detailed model of the Akt and MAPK pathways
with two known and one hypothesized crosstalk mechanisms.
The entire model contains 84 unknown parameters.
Our simulation results exhibit good correlation
with experimental data, and they also yield positive
evidence in support of the hypothesized crosstalk
between the two pathways.
Hide
Bistable
Switching and Excitable Behaviour in the Activation
of Src at Mitosis
Author(s):
Hendrik Fuß, University of Ulster, Coleraine,
Northern Ireland
Werner Dubitzky, University of Ulster, Coleraine,
Northern Ireland
Stephen Downes, University of Ulster, Coleraine,
Northern Ireland
Mary Jo Kurth, University of Ulster, Coleraine,
Northern Ireland
Motivation:
The protein tyrosine kinase Src is involved in a
multitude of biochemical pathways and cellular functions.
A complex network of interactions with other kinases
and phosphatases obscures its precise mode of operation.
Results:
We have constructed a semi-quantitative computational
dynamic systems model of the activation of Src at
mitosis from protein interactions described in the
literature. Through numerical simulation and bifurcation
analysis we show that Src regulation involves a
bistable switch, a pattern increasingly recognised
as essential to biochemical signalling. The switch
is operated by the tyrosine kinase CSK, which itself
is involved in a negative feedback loop with Src.
Negative feedback generates an excitable system,
which produces transient activation of Src. One
of the system parameters, which is linked to the
cyclin dependent kinase cdc2, controls excitability
via a second bistable switch. This topology allows
for differentiated responses to a multitude of signals.
The model offers explanations for the existence
of the positive and negative feedback loops involving
protein tyrosine phosphatase alpha PTPalpha and
translocation of CSK and predicts a specific relationship
between Src phosphorylation and activity.
Keywords: Dynamic Systems Modelling,
Bistability, Bifurcation Analysis, Computer Simulation,
pp60/Src Tyrosine Phosphorylation
Hide
Identification
of metabolic units induced by environmental signals
Author(s):
Jose Nacher, Bioinformatics Center, Institute
for Chemical Research, Kyoto University, Japan
Jean-Marc Schwartz, Bioinformatics Center, Institute
for Chemical Research, Kyoto University, Japan
Minoru Kanehisa, Bioinformatics Center, Institute
for Chemical Research, Kyoto University, Japan
Tatsuya Akutsu, Bioinformatics Center, Institute
for Chemical Research, Kyoto University, Japan
Motivation:
Biological cells continually need to adapt the activity
levels of metabolic functions to changes in their
living environment. Although genome-wide transcriptional
data have been gathered in a large variety of environmental
conditions, the connections between the expression
response to external changes and the induction or
repression of specific metabolic functions have
not been investigated at the genome scale.
Results:
We here present a correlation-based analysis for
identifying the expression response of genes involved
in metabolism to specific external signals, and
apply it to analyze the transcriptional response
of Saccharomyces cerevisiae to different stress
conditions. We show that this approach leads to
new insights about the specificity of the genomic
response to given environmental changes, and allows
to identify genes that are particularly sensitive
to a unique condition. We then integrate these signal-induced
expression data with structural data of the yeast
metabolic network and analyze the topological properties
of the induced or repressed subnetworks. They reveal
significant discrepancies from random networks,
and in particular exhibit a high connectivity, allowing
them to be mapped back to complete metabolic routes.
Hide
Inferring
functional pathways from multi-perturbation data
Author(s):
Nir Yosef, School of Computer Science, Tel-Aviv
University, Israel
Alon Kaufman, Center of Neural Computation, Hebrew
University, Israel
Eytan Ruppin, School of Computer Science, School
of Medicine, Tel-Aviv University
Background:
Recently, a conceptually new approach for analyzing
gene networks, the Functional Influence Network
(FIN) was presented. The FIN approach analyzes multiperturbation
(e.g., multi-knockout) experiments studying the
performance of a given cellular function under different
perturbations, to identify the main functional pathways
and interactions underlying its processing. The
FIN was shown to be useful for the analysis of genetic
and neural systems. Here we present and study a
new algorithm; the Functional Influence Network
Extractor (FINE), which is specifically geared towards
the accurate analysis of sparse cellular systems.
We employ it to study a conceptually fundamental
question of practical importance - how well should
we know the system studied (such that we can predict
its performance) so that we can understand its workings
(i.e., chart its underlying functional network)?
Results:
The performance of FINE is studied in both simulated
and biological sparse systems. It successfully obtains
an accurate and compact description of the underlying
functional network even with limited data, and is
shown to achieve markedly superior results in comparison
with its predecessor, the FIN. We provide ballpark
estimates of the levels of predictive knowledge
required for obtaining an accurate FINE reconstruction
of the functional backbone of the system investigated,
as a function of its complexity.
Conclusions:
The FINE algorithm provides a powerful tool for
learning how cellular functions are carried out.
Prior estimates of a system's functional complexity
are instrumental in determining how much predictive
knowledge is required to
accurately describe its function.
Hide
Dynamical
analysis of a generic Boolean model for the control
of the mammalian cell cycle
Author(s):
Adrien Fauré, Institut de Biologie du Développement
de Marseille-Luminy, France
Aurélien Naldi, Institut de Biologie du
Développement de Marseille-Luminy, France
Claudine Chaouiya, Institut de Biologie du Développement
de Marseille-Luminy, France
Denis Thieffry, Institut de Biologie du Développement
de Marseille-Luminy, France
Motivation:
To understand the behaviour of complex biological
regulatory networks, a proper integration of molecular
data into a full-fledge formal dynamical model is
ultimately required. As most available data on regulatory
interactions are qualitative, logical modelling
offers an interesting framework to delineate the
main dynamical properties of the underlying networks.
Results:
Transposing a generic model of the core network
controlling the mammalian cell cycle into the logical
framework, we compare different strategies to explore
its dynamical properties. In particular, we assess
the respective advantages and limits of synchronous
versus asynchronous updating assumptions to delineate
the asymptotical behaviour of regulatory networks.
Furthermore, we propose several intermediate strategies
to optimize the computation of asymptotical properties
depending on available knowledge.
Availability:
The mammalian cell cycle model is available in a
dedicated XML format (GINML) on our website, along
with our logical simulation software GINsim (http://gin.univ-mrs.fr/GINsim).
Higher resolution state transitions graphs are also
found on this web site (Model Repository page).
Keywords: regulatory networks,
cell cycle, dynamical modelling, logical modelling,
simulation
Hide
Computational
inference of the molecular logic for synaptic connectivity
in C. elegans
Author(s):
Vinay Varadan, Columbia University, U.S.A.
David Miller III, Vanderbilt University, U.S.A
Dimitris Anastassiou, Columbia University, U.S.A.
Motivation:
The nematode C. elegans is an ideal model organism
in which to investigate the biomolecular mechanisms
underlying the connectivity of neurons, because
synaptic connections are described in a comprehensive
wiring diagram and methods for defining gene expression
profiles of individual neurons are now available.
Results:
Here we present computational techniques linking
these two types of information. A systems-based
approach (EMBP: Entropy Minimization and Boolean
Parsimony) identifies sets of synergistically interacting
genes whose joint expression predicts neural connectivity.
We introduce an information theoretic measure of
the multivariate synergy, a fundamental concept
in systems biology, connecting the members of these
gene sets. We present and validate our preliminary
results based on publicly available information,
and demonstrate that their synergy is exceptionally
high indicating joint involvement in pathways. Our
strategy provides a robust methodology that will
yield increasingly more accurate results as more
neuron-specific gene expression data emerge. Ultimately,
we expect our approach to provide important clues
for universal mechanisms of neural interconnectivity.
Contact: anastas@ee.columbia.edu.
Supplementary Information:
Expression and connectivity data will be available
and maintained in the future as new results become
available, together with software and additional
clarifying descriptions of our techniques, on www.ee.columbia.edu/~anastas/ismb2006.
Hide
An
integrative approach for causal gene identification
and gene regulatory pathway inference
Author(s):
Zhidong Tu, University of Southern California,
USA
Li Wang, University of Southern California, USA
Michelle Arbeitman, University of Southern California,
USA
Ting Chen, University of Southern California,
USA
Fengzhu Sun, University of Southern California,
USA
Motivation:
Gene expression variation can often be linked to
certain chromosomal regions and are tightly associated
with phenotypic variation such as disease conditions.
Inferring the causal gene for the expression variation
is of great importance but rather challenging as
the linked region normally contains multiple genes.
Even when a single candidate gene is proposed, the
underlying biological mechanism by which the regulation
is enforced remains unknown. Novel approaches are
needed to both infer the causal genes and generate
hypothesis on the underlying regulatory mechanisms.
Results:
We propose a new approach which aims at achieving
the above objectives by integrating genotype information,
gene expres-sion, protein-protein interaction, protein
phosphorylation, and transcription factor (TF)-DNA
binding information. A network based stochastic
algorithm is designed to infer the causal genes
and identify the underlying regulatory pathways.
We first quantitatively verified our method by a
test using data generated by yeast knock-out experiments.
Over 40% of inferred causal genes are correct, which
is significantly better than 10% by random guess.
We then applied our method to a recent genome-wide
expression variation study in yeast. We show that
our method can correctly identify the causal genes
and effectively output experimentally verified pathways.
New potential gene regulatory pathways are generated
and presented as a global network.
Keywords: Gene regulatory pathway,
network, causal gene inference, eQTL
Hide
An
equilibrium partitioning model
connecting gene expression and cis-motif content
Author(s): Joe Mellor, Boston
University, USA
Charles DeLisi, Boston University, USA
Thermodynamic favorability of transcription factor
binding to DNA is a significant factor in the mechanistic
control of eukaryotic gene expression. Theoretical
and in vitro measures link the average equilibrium
energy of protein-DNA binding to the sequence variation
among binding sites across a genome. We investigate
another effect that may influence genomic regulation
- that varying levels of an active protein may regulate
different sets of genes, based on inferred affinities
of sites upstream of those genes. In the context
of both cooperative and noncooperative control of
expression, gene regulation by varying concentrations
of transcription factor is expected to follow patterns
of chemical partitioning on DNA sites of differing
affinity. Based on computational transcription factor
binding site discovery and genome-wide expression
data available in Saccharomyces cerevisiae, we examine
the potential link between the expression dynamics
and motif content for different sets of genes under
conditions with varying concentrations of different
transcription factors. With simple equilibrium model
of TF-gene regulation, we explore several cases
of significant correlation between the level of
intragenomic motif variation and the modeled TF
protein level that actuates the regulation of those
downstream genes. We discuss observed TF motif variants
for several yeast transcription factors, and the
potential biological functions of genes that are
regulated by differential response to high and low
concentrations of particular TFs. These regulatory
effects, when linked to intragenomic motif variation,
suggest that the sequences of transcription factor
binding sites are codependent with equilibrium regulatory
dynamics of different DNA binding proteins.
Hide
Top
|
|
Accessing
Images in Bioscience Literature through User-Interface
Designs and Natural Language Processing
Author(s):
Hong Yu, Columbia University/Department of Biomedical
Informatics, USA
Minsuk Lee, Columbia University/Department of
Biomedical Informatics, USA
Images (i.e., figures or tables) are important
experimental results that are typically reported
in bioscience full-text articles. Biologists need
to access images to validate research facts and
to formulate or to test novel research hypotheses.
On the other hand, biologists live in an age of
information explosion. As thousands of biomedical
articles are published every day, systems that help
biologists efficiently access images in literature
would greatly facilitate biomedical research. We
hypothesize that much of image content reported
in a full-text article can be summarized by the
sentences in the abstract of the article. In our
study, more than one hundred biologists had tested
this hypothesis and more than 40 biologists had
evaluated a novel user-interface BioEx that allows
biologists to access images directly from abstract
sentences. Our results show that 87.8% biologists
were in favor of BioEx over two other baseline user-interfaces.
We further developed systems that explored hierarchical
clustering algorithms to automatically identify
abstract sentences that summarize the images. One
of the systems achieves a precision of 100% that
corresponds to a recall of 4.6%. We implemented
BioEx that is accessible at http://www.dbmi.columbia.edu/~yuh9001/research/BioEx.html,
from which a user can query 17,000 downloaded Proceedings
of the National Academy of Sciences (PNAS) full-text
biological articles.
Hide
Finding
the evidences for protein-protein interactions from
PubMed abstracts
Author(s):
Hyunchul Jang, Electronics and Telecommunications
Research Institute, Korea
Jaesoo Lim, Electronics and Telecommunications
Research Institute, Korea
Joon-Ho Lim, Electronics and Telecommunications
Research Institute, Korea
Soo-Jun Park, Electronics and Telecommunications
Research Institute, Korea
Kyu-Chul Lee, Chungnam National University, Korea
Seon-Hee Park, Electronics and Telecommunications
Research Institute, Korea
Motivation:
Protein-protein interaction plays a critical role
in biological processes and many biologists try
to find or to predict crucial interactions information.
Before verifying in-teractions in biological laboratory
work, validating them from the previous research
is necessary. Although many efforts have been made
to create databases that store verified in-formation
in structured form, much interaction information
is still remained in unstructured text. As the amount
of new publications has increased rapidly, many
researches have been proposed to extract interactions
from the text automati-cally. But there are still
some difficulties to apply automati-cally generated
results into manually annotated databases. In case
of interactions that are not found in manually stored
databases, researchers are willing to try finding
abstracts or full papers by search.
Results:
As a result of search for two proteins, PubMed returns
over hundreds of abstracts frequently. We introduce
our method to validate protein-protein interactions
from PubMed abstracts. We generate a query from
given two proteins automatically and collect abstracts
from PubMed. Then we recognize target proteins including
their synonyms and extract their interaction information
from the collection. Conflicts, those are included
false positively, are detected and we resolve conflicted
interactions automatically. 67.37% of interactions
from DIP-PPI corpus are validated by Pub-Med abstracts
when 87.37% of interactions are validated by the
given full texts.
Hide
Novel
unsupervised feature filtering of biological data
Author(s):
Roy Varshavsky, School of Computer Science and
Engineering, The Hebrew University of Jerusalem, Israel
Assaf Gottlieb, School of Physics and Astronomy,
Tel Aviv University, Israel
Michal Linial, Deptartment of Biological Chemistry,
Institute of Life Sciences, The Hebrew University
of Jerusalem, Israel
David Horn, School of Physics and Astronomy, Tel
Aviv University, Israel
Motivation:
Many methods have been developed for selecting small
informative feature subsets in large noisy data.
However, unsupervised methods are scarce. Examples
are using the variance of data collected for each
feature, or the projection of the feature on the
first principal component. We propose a novel unsupervised
criterion, based on SVD-entropy, selecting a feature
according to its contribution to the entropy (CE)
calculated on a leave-one-out basis. This can be
implemented in four ways: simple ranking according
to CE values (SR); forward selection by accumulating
features according to which set produces highest
entropy (FS1); forward selection by accumulating
features through the choice of the best CE out of
the remaining ones (FS2); backward elimination (BE)
of features with the lowest CE.
Results:
We apply our methods to different benchmarks. In
each case we evaluate the success of clustering
the data in the selected feature spaces, by measuring
Jaccard scores with respect to known classifications.
We demonstrate that feature filtering according
to CE outperforms the variance method and gene-shaving.
There are cases where, the analysis based on a small
set of selected features, outperforms the best score
reported when all information was used. Our method
calls for an optimal size of the relevant feature
set. This turns out to be just a few percents of
the number of genes in the two Leukemia datasets
that we have analyzed. Moreover, the most favored
selected genes turn out to have significant GO enrichment
in relevant cellular processes.
Hide
Integrating
image data into biomedical text categorization
Author(s):
Hagit Shatkay, School of Computing, Queen's University,
Kingston, ON, Canada
Nawei Chen, School of Computing, Queen's University,
Kingston, ON, Canada
Dorothea Blostein, School of Computing, Queen's
University, Kingston, ON, Canada
Categorization of biomedical articles is a central
task for supporting various curation efforts. It
can also form the basis for effective biomedical
text mining. Automatic text classification in the
biomedical domain is thus an active research area.
Contests organized by the KDD Cup (2002) and the
TREC Genomics track (since 2003) defined several
annotation tasks that involved document classification,
and provided training and test data sets. So far,
these efforts focused on analyzing only the text
content of documents. However, as was noted in the
KDD'02 text mining contest - where figure-captions
proved to be an invaluable feature for identifying
documents of interest - images often provide curators
with critical information. We examine the possibility
of using information derived directly from image
data, and of integrating it with text-based classification,
for biomedical document categorization. We present
a method for obtaining features from images and
for using them - both alone and in combination with
text - to perform the triage task introduced in
the TREC Genomics track 2004. The task was to determine
which documents are relevant to a given annotation
task performed by the Mouse Genome Database curators.
We show preliminary results, demonstrating that
the method has a strong potential to enhance and
complement traditional text-based categorization
methods.
Hide
Top
|
|
Statistical
mechanical modeling of genome-wide transcription factor
occupancy data by MatrixREDUCE
Author(s):
Barrett Foat, Department of Biological Sciences,
Columbia University, New York, U.S.A.
Alexandre Morozov, Center for Studies in Physics
and Biology, The Rockefeller University, New York,
U.S.A.
Harmen Bussemaker, Department of Biological Sciences,
Columbia University, New York, U.S.A
Regulation of gene expression by a transcription
factor requires physical interaction between the
factor and the DNA, which can be described by a
statistical mechanical model. Based on this model,
we developed the MatrixREDUCE algorithm, which uses
genome-wide occupancy data for a transcription factor
(e.g. ChIP-chip) and associated nucleotide sequences
to discover the sequence-specific binding affinity
of the transcription factor. Advantages of our approach
are that the information for all probes on the microarray
is efficiently utilized because there is no need
to delineate "bound" and "unbound"
sequences, and that, unlike information content-based
methods, it does not require a background sequence
model. We validated the performance of MatrixREDUCE
by inferring the sequence-specific binding affinities
for several transcription factors in S. cerevisiae
and comparing the results with three other independent
sources of transcription factor sequence-specific
affinity information: (i) experimental measurement
of transcription factor binding affinities for specific
oligonucleotides, (ii) reporter gene assays for
promoters with systematically mutated binding sites,
and (iii) relative binding affinities obtained by
modeling transcription factor-DNA interactions based
on co-crystal structures of transcription factors
bound to DNA substrates. We show that transcription
factor binding affinities inferred by MatrixREDUCE
are in good agreement with all three validating
methods.
Hide
Quantification
of transcription factor expression from arabidopsis
images
Author(s):
Daniel Mace, Duke University, USA
Ji-Young Lee, Duke University, USA
Richard Twigg, Duke University, USA
Juliette Colinas, Duke University, USA
Philip Benfey, Duke University, USA
Uwe Ohler, Duke University, USA
Motivation:
Confocal microscopy has long provided qualitative
information for a variety of applications in molecular
biology. Recent advances have led to extensive image
datasets, which can now serve as new data sources
to obtain quantitative gene expression information.
In contrast to microarrays, which usually provide
data for many genes at one time point, these image
data provide us with expression information for
only one gene, but with the advantage of high spatial
and/or temporal resolution, which is often lost
in microarray samples.
Results:
We have developed a prototype for the automatic
analysis of Arabidopsis confocal images, which show
the expression of a single transcription factor
by means of GFP reporter constructs. Using techniques
from image registration, we are able to address
inherent problems of non-rigid transformation and
partial mapping, and obtain relative expression
values for 13 different tissues in Arabidopsis roots.
This provides quantitative information with high
spatial resolution, which accurately represents
the underlying expression values within the organism.
We validate our approach on a data set of 108 images
depicting expression patterns of 32 transcription
factors, both in terms of registration accuracy,
as well as correlation with cell-sorted microarray
data. Approaches like this will be useful to lay
the groundwork to reconstruct regulatory networks
on the level of tissues or even individual cells.
Hide
Identifying
cycling genes by combining sequence homology and expression
data
Author(s):
Yong Lu, Carnegie Mellon University, USA
Roni Rosenfeld, Carnegie Mellon University, USA
Ziv Bar-Joseph, Carnegie Mellon University, USA
Motivation:
The expression of genes during the cell division
process has now been studied in a many different
species. An important goal of these studies is to
identify the set of cycling genes. To date, this
was done independently for each of the species studied.
Due to noise and other data analysis problems, accurately
deriving a set of cycling genes from expression
data is a hard problem. This is especially true
for some of the multicellular organisms, including
humans.
Results:
Here we present the first algorithm that combines
microarray expression data from multiple species
for identifying cycling genes. Our algorithm represents
genes from multiple species as nodes in a graph.
Edges between genes represent sequence similarity.
Starting with the measured expression values for
each species we use Belief Propagation to determine
a posterior score for genes. This posterior is used
to determine a new set of cycling genes for each
species.
We applied our algorithm to improve the identification
of the set of cell cycle genes in budding yeast
and humans. As we show, by incorporating sequence
similarity information we were able to obtain a
more accurate set of genes compared to methods that
rely on expression data alone. Our method was especially
successful for the human dataset indicating that
it can use a high quality dataset from one species
to overcome noise problems in another.
Hide
Analysis
of sample set enrichment scores: assaying the enrichment
of sets of genes for individual samples in genome-wide
expression profiles
Author(s):
Elena Edelman, Duke University, USA
Alessandro Porrello, Duke Univeristy, USA
Justin Guinney, Duke Univeristy, USA
Bala Balakumaran, Duke University, USA
Andrea Bild, Duke University, USA
Phillip Febbo, Duke University, USA
Sayan Mukherjee, Duke University, USA
Motivation:
Gene expression profiling experiments in cell lines
and animal models characterized by specific genetic
or molecular perturbations have yielded sets of
genes “annotated” by the perturbation.
These gene sets can serve as a reference base for
interrogating other expression data sets. For example,
a new data set in which a specific pathway gene
set appears to be enriched, in terms of multiple
genes in that set evidencing expression changes,
can then be annotated by that reference pathway.
We introduce in this paper a formal statistical
method to measure the enrichment of each sample
in an expression data set. This allows us to assay
the natural variation of pathway activity in observed
gene expression data sets from clinical cancer and
other studies.
Results:
Validation of the method and illustrations of biological
insights gleaned are demonstrated on cell line data,
mouse models, and cancer-related datasets. Using
oncogenic pathway signatures, we show that gene
sets built from a model system are indeed enriched
in the model system. We employ ASSESS for the use
of molecular classification by pathways. This provides
an accurate classifier that can be interpreted at
the level of pathways instead of individual genes.
Finally, ASSESS can be used for cross-platform expression
models where data on the same type of cancer are
integrated over different platforms into a space
of enrichment scores.
Availability:
The code is available in Octave and a version with
a graphical user interface is available in Java.
All software can be downloaded at http://people.genome.duke.edu/assess
Contact: sayan@stat.duke.edu
Hide
Efficient
identification of DNA binding partners in a sequence
database
Author(s):
Tobias Mann, Dept. of Genome Sciences, University
of Washington, Seattle Washington, USA
William Noble, Dept. of Genome Sciences, University
of Washington, Seattle Washington, USA
Motivation:
The specific hybridization of complementary DNA
molecules underlies many widely used molecular biology
assays, including the polymerase chain reaction
and various types of microarray analysis. In order
for such an assay to work well, the primer or probe
must bind to its intended target, without also binding
to additional sequences in the reaction mixture.
For any given probe or primer, potential non-specific
binding partners can be identified using state-of-the-art
models of DNA binding stability. Unfortunately,
these models rely on computationally complex dynamic
programming algorithms that are too slow to apply
on a genomic scale.
Results:
We present an algorithm that efficiently scans a
DNA database for short (approximately 20--30 base)
sequences that will bind to a query sequence. We
use a filtering approach, in which a series of increasingly
stringent filters is applied to a set of candidate
$k$-mers. The k-mers that pass all filters are then
located in the sequence database using a precomputed
index, and an accurate model of DNA binding stability
is applied to the sequence surrounding each of the
k-mer occurrences. This approach reduces the time
to identify all binding partners for a given DNA
sequence in human genomic DNA by
approximately three orders of magnitude. Our method
can scan the human genome
for medium strength binding sites to a candidate
PCR primer in an average of 34.5 minutes.
Keywords: DNA Binding Sites, PCR
primer design, microarray probe design
Hide
Semi-supervised
analysis of gene expression profiles for lineage-specific
development in the Caenorhabditis elegans embryo
Author(s):
Yuan Qi, Massachusetts Institute of Technology,
USA
Patrycja Missiuro, Whitehead Institute, USA
Ashish Kapoor, Massachusetts Institute of Technology,
USA
Craig Hunter, Harvard University, USA
Tommi Jaakkola, Massachusetts Institute of Technology,
USA
David Gifford, Massachusetts Institute of Technology,
USA
Hui Ge, Whitehead Institute, USA
Gene expression profiling of mutant animals that
lack or in excess of certain tissues/cell lineages/cell
types is a common way to identify genes that are
important for the development and maintenance of
given tissues/cell lineages/cell types. However,
most of the currently available methods are not
suitable to effectively differentiate relevant gene
expression profiles from random profiles in this
context. A significant amount of false-positives
or false-negatives are introduced in data analysis
by conventional methods. We report a semi-supervised
learning algorithm that accurately captures relevant
expression profiles and identifies genes enriched
in given tissues/cell lineages/cell types. This
algorithm combines the advantages of classification
with the benefits of clustering. We apply this algorithm
to identify genes important for the development
of a specific cell lineage in the C. elegans embryo,
and to further predict the tissues in which these
genes are enriched. Compared to currently available
methods, our algorithm achieve higher sensitivity
and specificity. We confirm some of our predictions
by biological experiments.
Hide
Top
|
|
|
|