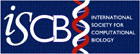
Hosted by
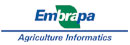
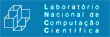
|
Poster
In silico identification and analysis of new Artemis/Artemis-like sequences from fungal and metazoan species
Authors:
Diego Bonatto (Universidade de Caxias do Sul)
Martin Brendel (Universidade Estadual de Santa Cruz)
João Antonio Pêgas Henriques (Universidade de Caxias do Sul)
Short Abstract: The mammalian Artemis proteins have important functions in the repair of DNA double-strand breaks and in the V(D)J recombination. We have characterized new Artemis/Artemis-like sequences from the genomes of fungi and non-mammalian metazoan species using an in-depth phylogenetic analysis coupled to hydrophobic cluster analysis and three-dimensional modeling of selected sequences.
Long Abstract:
Eukaryotic chromatin is a relatively easy target for reactive chemical and physical agents, including cross-linking substances and ionizing radiation, respectively. Both DNA and the nucleoproteins that compose chromatin can be irreversibly modified by these agents, resulting in chromosomal rearrangements, deletions and other genetic alterations. As chromosomal DNA contains most of an organism’s genetic information, modifications introduced in this molecule are potentially lethal if not repaired. Amongst all of these DNA lesions the double strand breaks (DSB) are the most dangerous lesions. Interestingly, the generation of DSB in genomic DNA is a common process in eukaryotic cells, occurring during certain stages of the life cycle, e.g. in meiosis or in DNA re-arrangements for antibody production in B cells. During evolution, eukaryotic cells have developed a complex network of proteins that, by sensing all types of DNA-damage and inducing the appropriate response, maintain the genome’s integrity. This network can be sub-divided into different DNA repair pathways, each controlled by cell cycle, damage types and substrate requirements. DSBs are primarily repaired by homologous recombination (HR) and/or by non-homologous end joining recombination (NHEJ). In the case of HR, the presence of a DSB elicits a genomic search for similar (homologous) sequences and the repair involves base pairing of long stretches of matched base pairs. In contrast, NHEJ is a mechanism able to join DNA ends with no, or minimal, homology. In addition, NHEJ is also used to repair DSBs that arise during early lymphocyte development in the context of V(D)J recombination. The NHEJ pathway contains six protein members namely Ku70, Ku80, XRCC4, DNA ligase 4 (Lig4), DNA-dependent protein kinase catalytic subunit (DNA-PKcs), and Artemis. Many proteins that participate in NHEJ or V(D)J recombination share a high homology, from yeasts to plants and animals, indicating the essentiality of this mechanism for cellular well-being. Artemis is a group of proteins that belongs to the beta-CASP family, a member of the metallo-beta-lactamase superfamily. Artemis has 5’ to 3’ exonucleolytic activity with single-strand DNA specificity and, when associated with DNA-PKcs, forms a phosphorylated complex with endonucleolytic activity on both 5’ and 3’ DNA overhangs. Furthermore, it can cleave hairpins generated by the Rag-1/Rag-2 proteins in V(D)J recombination. Artemis cooperates with p53 to suppress chromosomal translocation and tumor development in mice and, therefore, can be considered a tumor suppressor. Like other NHEJ/p53 doubly-deficient mice, most Artemis-deficient mice succumb to pro-B cell lymphomas at the age of 11–12 weeks. Moreover, Artemis interacts with the checkpoint kinase ataxia telangiectasia mutated protein (ATM) and ATM-/Rad3-related proteins (ATR) after exposure of cells to ionizing radiation (IR) or UV irradiation, respectively. These findings indicate that Artemis is required for the maintenance of a normal DNA damage-induced G2/M cell cycle arrest. However, despite the data obtained with mammalian cells on Artemis, little is known about how and when Artemis protein is recruited for DNA repair. Due to intrinsic difficulties in constructing mammalian cell lines with more than one knockout or knockdown gene, an alternative biological model allowing the study of Artemis in DNA repair would be welcome. Yeasts, especially the conventional species Saccharomyces cerevisiae and Schizosaccharomyces pombe, have many advantages as model organisms when compared to plants or metazoans. A large number of yeast mutant strains for many metabolic pathways and cellular components can be easily isolated, using a combination of sophisticated genetic and biochemical analyses. Also, yeast cells can grow rapidly in defined or complete culture media, their cell cycle can be synchronized, and many mutant strains can be tested for different phenotypes at the same time. An Artemis-like protein has not been discovered in conventional yeast species until now. But fungi, plants and metazoans contain an Artemis orthologue protein known as Pso2p/Snm1p. The family of Pso2p/Snm1p is divided in two groups: A and B, both associated with the recombinational repair of DSBs induced by chemical agents. Artemis and Pso2p/Snm1p have low aa sequence homology, indicating that both proteins possibly have different functions in DNA repair in metazoan cells. In this work, we have identified and characterized new members of the Artemis protein family, by searching in eukaryotic genomic databases using sensitive methods of phylogenetic analysis. Additional hydrophobic cluster analysis (HCA) allowed us to refine the results obtained from phylogeny and to map conserved domains in these new Artemis/Artemis-like proteins. HCA data was further confirmed by three-dimensional sequence modeling. The results indicates that Artemis probably belongs to an ancient DNA recombination mechanism that diversified with the evolution of multi-cellular eukaryotic lineage.
Poster
Improved membrane protein topology prediction by domain assignments
Authors:
Andreas Bernsel (Stockholm Bioinformatics Center, Stockholm University)
Gunnar von Heijne (Department of Biochemistry and Biophysics, Stockholm University)
Short Abstract: We have identified a set of domains that, when found in soluble proteins, have conpartment-specific localization of a kind relevant for membrane protein topology prediction. Using these domains as prediction constraints, we are able to provide high-quality topology models for 11% of the membrane proteins extracted from 38 eukaryotic genomes.
Long Abstract:
Alpha-helical transmembrane proteins constitute about 20% of all proteins encoded by most genomes (Krogh et al. 2001) and are responsible for several vital processes in the cell. In addition, the medical importance of membrane bound receptors, channels, and pumps as targets for drugs is well established. Still, for the large majority of membrane proteins, the structure or even the topology, i.e., the positions and in/out-orientations of all transmembrane helices, is not known experimentally. The continuously growing amount of sequence data, in combination with the limited amount of structural data available, highlight the need for better and more accurate theoretical structure prediction methods, particularly for the annotation of membrane proteins. <br><br> Protein domains are modular, independently evolving, and structurally similar amino acid segments, which may exist alone in single-domain proteins, or may combine to form multi-domain proteins. Although covalent combinations between transmembrane domains, i.e., domains with one or more membrane spanning regions, rarely occurs, covalent combinations between soluble domains and transmembrane domains are observed frequently (Liu et al. 2004). Moreover, domains are often compartment-specific, and information about domain occurrence can be used to predict the subcellular localization of soluble proteins (Mott et al. 2002). <br><br> Here, we explore the possibility that the presence of compartment-specific extra-membaneous protein domains in transmembrane protein sequences might be used as a constraint in a subsequent topology prediction step, in much the same way that experimentally determined “anchor points” have been used to constrain topology predictions (Kim et al. 2003; Rapp et al. 2004; Daley et al. 2005). Unconstrained topology predictions are correct for only ~55-60% of all membrane proteins (Melén et al. 2003), while compartment-specific domains that are always located on just one side of a membrane (facing, e.g., the extracellular space or the cytosol) can be identified with high reliability. If such a domain is found in a membrane protein, that particular segment in the protein sequence can be fixed to the corresponding side of the membrane before applying a sequence-based topology prediction algorithm on the rest of the sequence. Here, we show that domains of this kind are found in at least 11% of many eukaryotic proteomes, and that a significant improvement in topology prediction can be achieved by using these domains as prediction constraints. <br><br> Our basic approach consists of three steps: <br><br> - Domain selection. Identify compartment-specific domains that always reside on either the in- or outside of the membrane. Each domain is represented by a profile Hidden Markov Model (HMM). In general, we considered domains annotated as “extracellular” in the SMART 4.0 domain database to reside outside of the membrane (i.e. on the non-cytoplasmic side), and domains annotated as “signaling” to reside on the inside of the membrane (i.e. on the cytoplasmic side), which is in agreement with e.g. (Mott et al. 2002). In an attempt to assess the validity of this assumption, the domains were assigned to 297 homology reduced sequences of membrane proteins with experimentally known topologies. This resulted in 48 domain hits, contained in 29 (10%) of the sequences. Out of all domain hits, 47 (98%) were in agreement with the topology. One domain was in conflict with a known topology, and was thus removed from the domain collection. Although the test set is small, we consider our domain collection as highly reliable. <br><br> - Domain assignment. The final domain list used for placing constraints on the topology predictions consisted of 367 domains, of which 146 were “IN-domains” (i.e. appear only on the cytoplasmic side of the membrane), and 221 were “OUT-domains” (i.e. appear only on the non-cytoplasmic side of the membrane). For each query sequence, we now try to find one or more of the domains and fix those residues to the corresponding side of the membrane. <br><br> - Topology prediction. In the last step, we use a sequence-based method to predict the topology of the remaining part of the protein sequence, with the domain(s) found in the previous step constrained to either the in- or outside of the membrane. <br><br> Based on the constrained predictions, the topologies of all proteins containing at least one soluble domain were analyzed. 66% of those were single-spanning proteins, compared to just 37% in the complete set of predicted membrane proteins, suggesting that our method will have particular impact on single-spanning proteins. Single-spanning proteins are often mis-predicted by the current topology prediction methods, mostly due to an inversion of the predicted topology such that the TM-segment is correctly located but the overall orientation is wrong. Large extra-membraneous domains carry little or no orientational information in the current predictors, and our domain-based method thus solves a major weakness in these methods. <br><br> References: <br><br> Krogh, A., Larsson, B., von Heijne, G., and Sonnhammer, E. (2001) Predicting transmembrane protein topology with a hidden Markov model. Application to complete genomes. J Mol Biol 305: 567-580. <br><br> Liu, Y., Gerstein, M., and Engelman, D.M. (2004) Transmembrane protein domains rarely use covalent domain recombination as an evolutionary mechanism. Proc Natl Acad Sci U S A 101: 3495-3497. <br><br> Mott, R., Schultz, J., Bork, P., and Ponting, C.P. (2002) Predicting protein cellular localization using a domain projection method. Genome Res. 12: 1168-1174. <br><br> Kim, H., Melén, K., and von Heijne, G. (2003) Topology models for 37 Saccharomyces cerevisiae membrane proteins based on C-terminal reporter fusions and prediction. J Biol Chem 278: 10208-10213. <br><br> Rapp, M., Drew, D.E., Daley, D.O., Nilsson, J., Carvalho, T., Melén, K., de Gier, J.W., and von Heijne, G. (2004) Experimentally based topology models for E. coli inner membrane proteins. Prot Sci 13: 937-945. <br><br> Daley, D.O., Rapp, M., Granseth, E., Melén, K., Drew, D., and von Heijne, G. (2005) Global topology analysis of the Escherichia coli inner membrane proteome. Science: 308:1321-1323. <br><br> Melén, K., Krogh, A., and von Heijne, G. (2003) Reliability measures for membrane protein topology prediction algorithms. J Mol Biol 327: 735-744.
Poster
Align-GVGD: a Novel Sequence Analysis Tool for Predicting the Function of Missense Mutations
Authors:
Ewy Mathe (Laboratory of Human Carcinogenesis, NIH)
Magali Olivier (Group of Molecular Carcinogenesis, IARC)
Shunsuke Kato (Department of Clinical Oncology, Institute of Development Aging and Cancer)
Chikashi Ishioka (Department of Clinical Oncology, Institute of Development Aging and Cancer)
Catherine Voegele (Genetic Susceptibility Group, IARC)
Pierre Hainaut (Group of Molecular Carcinogenesis, IARC)
Sean Tavtigian (Genetic Susceptibility Group, IARC)
Short Abstract: Align-GVGD, available online at http://agvgd.iarc.fr, aims to predict the functional impact of missense mutations using user-input multiple sequence alignments. The functional outcome of 1514 missense substitutions in TP53 were predicted using Align-GVGD and the results were compared against experimentally measured transactivation activity (yeast assays). Our predictions, comparable to those obtained by SIFT, yielded ~88% specificity and 67.9 to 71.2% sensitivity.
Long Abstract:
Because missense substitutions are oftentimes associated with disease susceptibility genes, the accurate prediction of their functional effect is of particular interest. A novel sequence analysis tool, Align-GVGD, was recently implemented to predict whether missense mutations are enriched deleterious, enriched neutral, or unknown. Based on a user-input multiple sequence alignment, the following two conservation scores are computed to make the predictions: (1) Grantham Variation (GV) reflects the degree of biochemical variation among amino acids found at a given position in the MSA, (2) Grantham Deviation (GD) reflects the “biochemical distance” of the mutant amino acid from the observed amino acid variation at a particular position (given by GV). GV and GD cutoff values, based on biophysical reasoning, are applied to make the predictions. The tool had previously been successfully applied to BRCA1and BRCA2, to predict the impact of missense mutants in these susceptibility genes. To further validate the algorithm, we applied Align-GVGD to 1514 missense substitutions in TP53, the most frequently mutated gene in human cancers, and compared our predictions against experimentally measured transactivation activities (in yeast assays). The GV values were compared with the TP53 mutation frequencies (derived from the IARC TP53 database), which reflect the association of a mutation with cancer. The comparison reveals concordance between conserved residues (low GV value) and high mutation frequencies, suggesting that conserved positions are targeted for mutation during tumorigenesis. When mapping the GV values onto the 3D structure of the DNA binding domain of p53, one notices that residues with low GV values are those that have an important functional impact, such as those in the DNA binding domain, zinc binding domain, and in the core domain (important for maintaining stability). The predictions using Align-GVGD resulted in a high prediction accuracy for mutants that showed a loss of transactivation (specificity of ~88%) and in a lower prediction accuracy for mutants that showed a similar transactivation to the wild-type (67.9 to 71.2% sensitivity). Importantly, the prediction accuracies are highly dependant on the input MSA. As more distantly related sequences were added to the MSA, the sensitivity increased while the specificity remained nearly the same. This result suggests that using a more informative MSA with both closely and distantly related sequences as input will yield more accurate predictions. Finally, the efficiency of Align-GVGD with respect to other available tools was assessed by comparing our prediction accuracies with those obtained by the well-known mutant prediction tool SIFT (Sorting Intolerant From Tolerant) and Dayhoff’s classification. The Align-GVGD predictions were comparable to those obtained using SIFT (the same MSA used for Align-GVGD were input into SIFT), which yielded 88.3 to 90.6% and 67.4 to 70.3% specificity and sensitivity, respectively. One advantage of SIFT is that it can automatically generate an MSA. However, the prediction accuracies obtained from SIFT are not dependant on the input MSA, such that adding more distantly related sequences to the MSA did not clearly impact the prediction outcomes. Furthermore, with the measures of biochemical variation provided by Align-GVGD, one can easily explore the features of amino acids relevant to a specific mutation to help explain the weak or strong correlations between GV, GD, and function. Both SIFT and Align-GVGD outperformed the prediction accuracies resulting from Dayhoff’s classification. Overall, Align-GVGD provides a new means for predicting the functional impact of missense substitutions and provides comparable results to SIFT. We expect the algorithm to be applicable to other proteins which have a sufficient amount of sequences to provide an informative MSA as input. If possible, multiple MSAs should be tried to find an optimal combination of sensitivity and specificity. Importantly, the GV and GD cutoff values applied to make the predictions are not derived from any optimization over a dataset. This property implies that the cutoff values are not over-fitted to any particular type of protein. The program is freely available online at http://agvgd.iarc.fr.
Poster
Plant Transcription Factors
Authors:
Diego Mauricio Riaño Pachón (Department of Molecular Biology, Institute of Biochemistry and Biology, University of Potsdam)
Ingo Dreyer (Department of Molecular Biology, Institute of Biochemistry and Biology, University of Potsdam)
Slobodan Ruzicic (Cooperative Research Group, Max Planck Institute of Molecular Plant Physiology)
Bernd Mueller-Roeber (Department of Molecular Biology, Institute of Biochemistry and Biology, University of Potsdam)
Short Abstract: Transcription factors(TF) are proteins that play a central role in the regulation of gene expression, usually they are members of gene families. Here we have identified the members of up to 53 TF families of plant whose genome sequences are available (Oryza sativa, Arabidopsis thaliana, Chlamydomonas reinhardtii, Ostreococcus tauri).
Long Abstract:
A list of protein domains involved in plant transcriptional regulation was built through keyword searches at the PFAM’s website and from literature. PFAM (Bateman et al. 2004) global profile-HMMs for those domains, together with HMMER package (Eddy 1998), were employed to search the complete protein set from Oryza sativa (latest genome annotation from TIGR, 2006), Arabidopsis thaliana (TIGR, 2004), Chlamydomonas reinhardtii (JGI/DOE, 2006) and Ostreococcus tauri (University of Gent, 2006). In a few cases, when no appropriate domain models were found in PFAM, we created our own profile-HMMs (e. g. G2-like, NF-YB, NF-YC, CCAAT-Dr1, Trihelix) based on published multiple alignments. A total of 56 domains were employed. Proteins bearing those domains are regarded as putative transcription factors. Proteins were clustered into 52 families, based on their domain architecture, mainly following Riechmann (2002), and one orphan family. A set of rules were devised in the form: Family X = Domain A AND Domain B AND (Domain D OR NOT Domain D) AND NOT Domain C The boolean operator “AND” specifies a required relation. Both domains (A and B) must be present in a protein to be assigned to Family X. The operator “AND (… OR NOT …)” specifies an optional relationship, the domain (D) is not required, though it could appear in some members of the family. The operator “AND NOT” specifies that a domain (C) should not be present in any member of the family. Following this procedure the families obtained are completely exclusive. Putative transcription factors classified into families with their accessory domains are available at: http://ricetfdb.bio.uni-potsdam.de, http://arabtfdb.bio.uni-potsdam.de, http://chlamytfdb.bio.uni-potsdam.de/ and http://ostreotfdb.bio.uni.potsdam.de/. In most cases, upstream, gene, coding and protein sequences are also available. Multiple alignments are available at the domain level for each family. For 32 families the presence of a single distinctive domain is the only requirement to assign membership. The remaining 20 families have different combinations (presence/absence) of 32 different domains. A graph was created to visualize the rules that lead to the definition of each family. This is a bipartite graph, in which one set of vertices represents the transcription factor families, and the other set represents protein domains. There are two types of edges, one type represents a REQUIRED relationship, the other type represents a FORBIDDEN relationship. From this graph the complete set of rules can be easily reconstructed (graph available at: http://ricetfdb.bio.uni-potsdam.de/v2.0/rules.php). In total, 2856 protein models (53 families) harbor domains involved in transcriptional regulation, and are regarded as putative transcription factors in O. sativa, 2462 (53 families) in A. thaliana, 229 (33 families) in C. reinhardtii and 193 (29 families) in O. tauri. We assume that the number of proteins found is a lower bound for the total number of transcription factors in the studied species. RiceTFDB has been publicly available since June 5, 2004, and it has served more than 6000 different hosts, from around the world. As of 1st March 2006, a new version (v2.0) of the RiceTFDB is publicly available. From 1st of April ChlamyTFDB, ArabTFDB and OstreoTFDB will be as well. References Bateman A, Coin L, Durbin R, Finn RD, Hollich V, Griffiths-Jones S, Khanna A, Marshall M, Moxon S, Sonnhammer EL, Studholme DJ, Yeats C, Eddy SR. (2004). The Pfam protein families database. Nucleic Acids Res. 32:D138-41. Eddy, S. R. (1998). Profile hidden Markov models. Bioinformatics, 14:755-763. Riechmann, J.L. (2002). Transcriptional Regulation: A Genomic Overview. pp:1-46. In: Somerville, C. & Meyerowitz, E. (Eds.) The Arabidopsis Book. American Society of Plant Biologists, Rockville, MD. USA. 2002.
Poster
Protein Recognition Processes: The
Authors:
Anna Feldman-Salit (EML Research)
Domantas Motiejunas (EML Research)
Dr. Razif Gabdoulline (EML Research)
Dr. Markus Wirtz (Heidelberg Institute for Plant Science)
Dr. Rüdiger Hell (Heidelberg Institute for Plant Science)
Dr. Rebecca Wade (EML Research)
Short Abstract: Cysteine biosynthesis in plants and bacteria involves a bienzyme
Long Abstract:
Plants and bacteria can assimilate and incorporate inorganic sulfur into organic compounds such as the amino acid, cysteine. Producing cysteine plants make sulfur available to animals and humans where it is required for the synthesis of essential compounds, including vitamins and metal clusters. Cysteine biosynthesis in plants and bacteria is a dual-step pathway, which involves a bienzyme complex formation, the
Poster
Self-organizing Maps - the Heuristic Approach for Understanding Ligand-protein Interactions in Vitamin D Receptor.
Authors:
Ferdinand Molnár (Department of Biochemistry, University of Kuopio)
Short Abstract: Since ligand-docking is more dynamic process than crystallography can monitor, we analyzed the action of different agonists using molecular-dynamics simulations, self-organizing maps and in-vitro assays. The combined movement of 40 residues results in the ligand-binding pocket modulation and may serve as an important parameter in smart drug design.
Long Abstract:
INTRODUCTION. Existing crystal structure data has indicated that 1apha,25-dihydroxyvitamin D3 (1apha,25(OH)2D3) and its analogues bind the ligand-binding pocket (LBP) of the human vitamin D receptor (VDR) in a very similar fashion. Since docking of a ligand into the LBP is a more flexible process than crystallography can monitor, we analyzed 1apha,25(OH)2D3, its 20-epi derivative MC1288, the two-side-chain analogues Gemini and Ro43-83582 (a hexafluoro-derivative) by molecular dynamics simulations in a complex with the VDR ligand-binding domain and a co-activator peptide. METHODS. To group the amino acids forming the LBP of VDR with similar movement patterns we used a data-mining clustering algorithm of self-organizing maps (SOMs). SOMs is an artificial neural network algorithm in the unsupervised learning category which is useful in the visualization and interpretation of large high-dimensional data sets. A map consists of a regular grid of processing units,
Poster
Application of Computational Biology Methods to Predict how Mutations Can Influence Protein Function: the Case of GALT.
Authors:
Anna Marabotti (Institute of Food Science, CNR, Avellino (Italy))
Andrew C.R. Martin (Dept. of Biochemistry and Molecular Biology, University College London)
Angelo Facchiano (Institute of Food Science, CNR, Avellino (Italy))
Short Abstract: The aim of the present work is to offer an interpretation, using biocomputational tools, of how every known single point mutations can affect structure, function and stability of the enzyme galactose-1-phosphate uridylyltransferase (GALT). Mutations in this enzyme are responsible for the genetic disease called “classical galactosemia”.
Long Abstract:
The genetic disease “classical galactosemia” (OMIM: 230400) is caused by more than 150 mutations of the gene codifying for the enzyme galactose-1-phosphate uridylyltransferase (GALT), which is involved in the metabolism of galactose. More than 60% of known mutations are missense mutations, and they can typically be associated with geographic distribution, or ethnic groups. The effects of this disease can range from mild to extremely severe, and although an early dietary galactose restriction can avoid or revert symptoms, in several cases dysfunctions can persist permanently. Several biochemical characterizations have been made on human GALT and on a very small number of mutants expressed in yeast, as well as on the bacterial enzyme from E. coli, which shares about 46% sequence identity with the human one. Studies of the structural features of GALT were performed especially on the bacterial enzyme. Recently, a theoretical model of the human GALT enzyme obtained by homology modelling was created by us. Starting from this structure, a complete analysis of the influence of the most frequent mutation in the european population, Q188R, was made to highlight its effect on structure, function and interchain relationships. The availability of the 3D structure of the human enzyme allowed us to predict the effects, at a molecular level, of all known GALT missense mutations with the aid of computational biology tools. We simulated each mutant of the GALT enzyme by replacing the wild type residue with the corresponding mutation(s). Since this is a dimeric enzyme, we inserted each mutation in both subunits, thus creating homodimeric mutants. The mutant residue and the amino acids with at least one atom at a distance of no more than 5 Å from the mutation site were then allowed to optimize their conformation. Then, we evaluated the impact of the new side chain on the neighbours, the addition or the loss of stabilizing interactions, the impact on substrate binding and on interchain relationships. Finally, we estimated the impact of the mutation on the stability of the overall structure using predictors available online. From our results, it is possible to group the mutations into 5 main classes: 1. mutations with impact on the substrate binding and/or enzymatic reaction, 2. mutations affecting interchain relationships, 3. mutations involving global stability of the protein, 4. mutations influencing secondary structure formation and 5. mutations with no apparent effects on the structure and function of the GALT enzyme. A possible explanation for this last category could be that several rare mutations falling into this class are identified in combined heterozygous patients, and therefore a simple polymorphism with no negative effects on the protein activity, can have a negative impact on GALT activity if associated with a severe mutation. Other possible explanations can also be associated with in vivo phenomena, such as the anomalous compartmentalization of the protein in subcellular organelles, or the premature degradation of the enzyme. Several mutations have an evident impact on substrate binding and catalysis either directly, or indirectly with the perturbation of side chain conformations of the residues in the active site. The analysis of the residue conservation shows that, among the residues affected by galactosemia-related mutations, only four are conserved in all currently known GALT sequences from prokaryotic and eukaryotic organisms (W154, G179, H184 and Q188). All of these are in direct contact with the substrate; simulations confirm that their mutation clearly alters the relationships between the enzyme and the substrate. Intersubunit perturbations are classically caused by the loss of H-bonds or salt bridges due to the mutation of one of the two partner side-chains. Several intersubunit perturbations also impact on the active site, since the two monomer chains of GALT come together to form the active sites. Generally these mutations can alter deeply the local structure of the protein, and probably also its overall stability and dimerization. Mutations affecting intrachain relationships and/or backbone stability, or other features like metal binding, probably have a limited impact on the activity of the protein. However it is not possible to exclude the possibility that the presence of a local perturbation can have long range influence on other parts of the enzyme. Overall, we hope that our current analysis will help all people involved in biochemical, structural and clinical studies of this genetic disease. Since the biochemical characterization of each single mutation would require a massive effort, and the determination of the 3D structure of each mutant would require decades, we hope that the results from our studies could either aid in suggesting possible interpretations for the impact of mutations both at molecular and at clinical levels, as a guide for future experiments to clarify or confirm deductions obtained from this study. Our future goal will be the characterization of heterozygous single and combined mutants, together with the idea of allowing new interpretations of the impact of mutations at a molecular level. Finally, it is our intention to add all these results to our newly created database (GALTProt database) to share them with the entire Internet community.
Poster
Protein function prediction and classification using uncertainty
Authors:
Chris Needham (University of Leeds)
James Bradford (University of Leeds)
Andrew Bulpitt (University of Leeds)
David Westhead (University of Leeds)
Short Abstract: We use Bayesian networks to integrate numerous data sources including sequence homology, protein-protein interaction and gene expression data to assign Gene Ontology functional categories to proteins of Arabidopsis thaliana.
Long Abstract:
High-throughput sequencing and structure elucidation of proteins has led to a vast amount of proteomic data. In order to exploit this information we use Bayesian networks to integrate numerous data sources including sequence homology, protein-protein interaction and gene expression data to assign function to proteins of Arabidopsis thaliana. Our functional annotations are based on the gene ontology (GO) classification scheme. The representation of the GO as a directed acyclic graph (DAG) allows its implementation as a Bayesian network in order to handle uncertain data and relate functional categories.
Poster
Evolution of Death-domain-superfamily in apoptosis pathway
Authors:
Juilee Thakar (Department of Bioinformatics, University of Wuerzburg and Department of Physics, Pennsylvania state)
Yogeshwar Kelkar (Integrated biosciences,Department of Biology, Pennsylvania state university)
Christoph Borner (Center for biochemistry and molecular cell biology, University of Freiburg)
Thomas Dandekar (Department of Bioinformatics, Wuerzburg)
Short Abstract: Apoptosis takes place during normal development and pathological conditions. Proteins with domains from death-domain-superfamily are involved in regulation of apoptosis pathway at many instances. We performed sequence, phylogenetic and structural analysis on domains from this family to look for conserved elements to comment on their role in signaling cascades.
Long Abstract:
Introduction: Apoptosis is necessary for maintaining homeostasis of the cell. It takes place during normal development and pathological conditions. Apoptosis signaling cascade is misregulated during many diseases. Proteins with domains from death-domain-superfamily are involved in regulation of apoptosis pathway at many instances. The death-domain-superfamily includes three domains: death domain (DD), death effector domain (DED) and caspase-recruitment domain (CARD). All these domains have conserved structural elements that constitutes of six anti-parallel helix bundles or as sandwiches with Greek key connectivity. One face is formed by helices 1, 4 and 6 and the opposite face by helices 2, 3, and 5. These domains are involved in homotypic interactions.
DDs are present in receptor and adaptor proteins and are involved in decision making interactions during apoptosis signaling. The sequence analysis has shown that DDs of adaptors are more diverged than DDs of receptors*. Our previous studies in DD propose the possibility of the presence of sub-domains (in the previous study we found structural sub-domains in a DD. We extend our analysis to see the availability of such domains in the death-domain-superfamily). Further the phylogenetic analysis also shows that DDs of pro-apoptotic proteins and anti-apoptotic proteins fall in different groups*. We have proposed two exclusive conformation of complexes one of which leads to the death of the cell and another conformation leads to survival/ proliferation.
Methods: We curated domain sequences in fasta format from SMART database version 4. Then we performed phylogenetic analysis using PHYLIP 3.62 which uses a neighbor joining matrix for generating a phylogenetic tree. We used SWISS MODEL to model unknown structures of domains. Further docking program, 3D Dock was used to analyze new interacting surfaces. The results proposed the availability of sub-domains in DD.
In the next step we decided to map and compare the differences in substitution rates and mutations in three domains to analyse sub-domains. Phylogenetic analysis was used to understand their functions. We used CLUSTAL W and seaview for sequence analysis. Higher level of analysis was performed using HyPhy. We used Jones substitution matrix to calculate site specific substitution rates. The substitution rates (SRs) of sites which include more than 40% gaps in multiple sequence alignment were omitted.
Results and Discussion: We started the analysis with DDs involved in TNF receptor I (TNFRI) signaling as TNFRI transduce death and survival signals. Since we concentrated on one signaling pathway we could classify DDs and perform structural analysis on few proteins, results of which showed the presence of sub-domains in DDs. We then proposed the model to elucidate the role of DD in the decision making between apoptosis and survival during TNFRI induced signaling. The results of docking studies performed on five DD-DD complexes show that DD sub-domains of RIP can interact in two exclusive conformation with TRADD leading to either recruitment of CRADD (leading to apoptosis mediated by caspase-2) or NFkB (leading to survival/ proliferation)*.
As DDs are involved in many homotypic interactions we asked how the specificity between interacting partners is maintained. Our previous studies show that DD of adaptor sequences are more diverged than receptor sequence*. We believe that divergence in the sequences is necessary for maintaining (and probably increasing) specificity of interacting partners in such a complicated pathway. Divergence in adaptor DD sequences indicates their important role in maintaining the specificity during interactions. In the apoptosis signaling DD containing adaptor proteins might play decisive role in the recognition of the next protein in the cascade. Whereas less divergence in receptor DDs suggest that DD containing protein which interact with receptor is possibly held in proximity to receptor to interact with it upon activation of the receptor. These interesting results in DDs lead us to extend the analysis to all other members of death-domain-superfamily. The preliminary results in humans show that DED sequences are more conserved than DD and CARD sequences. The average pairwise score (APS) of multiple sequence alignment can be ordered in decreasing APS as: DED (31.53) > CARD (25.89) > DD (19.05) in humans. DD and DED containing proteins contribute in the upper half of the apoptosis pathway and are involved in many decision making interactions during apoptosis signaling. It is interesting that though DED is involved in decision making interactions it has the higher APS than CARD. The first CARD containing proteins that are activated during apoptosis signaling are recognized through DED or other domains. Thus we expected to have highest APS for CARD so we performed more analysis. Phylogenetic analysis shows that CARD (in an unrooted tree 95% bootstrap values are greater than 80 (out of 100)) domains have better defined ancestral sequence than DDs (very low boostrap values) and DEDs (very low boostrap values). This led us to analyze substitution rates and their distribution in these three domains.
Substitution rate calculation shows variation across six helices in DD, DED and CARD. We observed the highest substitution rate (39.76) at position 21 in DD (1st alpha helix), this site was also involved in many DD interactions during docking studies that we performed*. SRs in DED were less than DD SRs, the highest SRs were found at positions 16 and 27 (9.88) (in alpha helix 1 and 2 respectively). We believe that sites that have high substitution rates play important role in the recognition of downstream protein. Standard deviation of SRs can be ordered in decreasing order as: DD (17.60) > DED (2.53) > CARD (0.67). We assumed the SRs of CARD are the basal SRs present in death-domain-superfamily. SRs in CARD domain do not vary a lot which can be explained as CARD domain is not involved in decision making interaction in apoptosis cascade. We could say that sites with SRs greater than basal SRs are involved in the recognition. Further the distribution of SRs strengthens the presence of sub-domains.
Conclusions: Results show that domains involved in decision making interactions in apoptosis signaling cascade have higher variation in substitution rates. Lowest APS and highest standard deviation in DDs in humans shows that these domains are critical for recognition during signaling. This can also be supported by an observation that DD containing proteins are not only present in survival and death signaling cascades but also involved in decision making steps.
* Thakar J, Schleinkofer K, Borner C, Dandekar T. RIP death domain structural interactions implicated in TNF-mediated proliferation and survival. Proteins. 2006; 63(3): 413-423
Poster
dummy2
Authors:
Vera S. Garcia (Unicamp/Embrapa)
Paula Kuser Falcão (Embrapa)
Short Abstract: Basically all biological processes require protein-ligand identifications. One major challenge to biophysics-chemistry is try to predict these interactions. One step towards solving this mystery is analyzing the structural features involved in the complex formation. A good biological model to start these studies is the cystein-protease family.
Long Abstract:
Many fundamental biological processes require protein/ligand identifications to fulfill their biological function. This process is termed biological recognition. One major challenge to biophysics-chemistry is to try to predict these interactions (recognition) between proteins and their ligands (Shager, 2003). Being able to predict the protein recognition methods using structural data would be valuable in understanding the structural-functional relationship and also would facilitate the drug design procedure. One step toward acquiring this knowledge is to analyze all the structural features involved in the complex formation, aiming to understand the structural interactions with their complex pattern of recognition and specificity, and trying to map those features responsible for the enzyme interactions with a specific substrate (Fersht, 1999; Neshich et al., 2005).
For this work, it was established that a good biological model should have: a well described catalytic mechanism; a good number of structurally solved representatives in the Protein Data Bank (Bermann et al., 2000), including the isolated enzyme and in complex with different substrates; being best if found in all live kingdoms and the possibility to raise a grater interest (for example being a drug target). One of the families meeting the criteria mentioned above is the cystein-protease family.
Cystein-protease constitutes an important class of enzymes involved in the formation and hydrolysis of peptide bonds. Besides its obvious vital role in cell homeostasis, it is involved in apoptosis, Parkinson disease, muscle dystrophy, osteoporosis, is a potential target for parasitic treatment, and more (Podgorski & Sloane, 2003; Driessen et al., 1999). It includes plant proteases as papain and actinidin, mammalian lysosomal proteases (cathepsins B, C, H, K, X, etc.) cytosolic calcium activated proteases (calpain), parasitic proteases (cruzain), viral proteases (picornain and adenain) and many others (McGrath et al., 1999; Brocklehurst, 1998). This large family of enzymes has a characteristic molecular topology, and all have in common the catalytic triad Cys, His, Asn (or Asp). Briefly the catalytic mechanism involves the deprotonation of the cystein sulfhydryl by the adjacent histidine, followed by the nucleophilic attack of the sulfur on the peptide carbonyl carbon (Papamichael et al., 2004).
Initially, we selected the protein structures of the members of this family using different data bases. Since the study requires protein structures solved with good resolution, only experimentally determined structures properly validated with resolution 2.9 Å or higher were selected. Both, search methods and manual analysis were used to exclude the “false positive” family members. Since the proteins grouped into families have in common evolutionary relationship and enzymatic activities and the investigation is based, primarily, in structural features, structural analysis were done to create a data bank of similar structures for these family members, forming a structural cluster inside the family. This data bank excluded proteins which the overall structure were easily identified as being different from every other members.
With those structural clusters the alignment experiments were performed and the differences analyzed. In the next step we will perform docking experiments with the structural available substrates. With these we hope to be able to infer some structural parameters that are crucial for the interaction and complex formation.
References:
Berman, H. M., Westbrook, J., Feng, Z., Gilliland, G., Bhat, T. N., Weissig, H., Shindyalov, I. N. & Bourne, P. E (2000). The Protein Data Bank. Nucleic Acids Res. 28, 235-242.
Brocklehurst, K., Watts, A.B., Patel, M, Thomas, E.W. (1998), in Comprehensive biological catalysis, vol. 1, M. Sinnott, ed., Academic Press, 381.
Driessen, C., Bryant, R. A. R., Lennon-Duménil, A. M., Villadangos, J. A., Bryant, P. W., Shi, G. P., Chapman, H. A., Ploegh, H. L. (1999). Cathepsin S controls the trafficking and maturation of MHC class II molecules in dendritic cells. J. Cell Biol., 147, 775-790.
Fersht, A. (1999). Structure and mechanism in protein Science: A Guide to Enzyme Catalysis and Protein Folding. W.H. Freeman and company, New York.
McGrath, M. E. (1999). The lysosomal cysteine proteases. Annu. Rev. Biophys. Biomol. Struct., 28, 181-204.
Neshich G., Borro L C., Higa R. H., Kuser P. R., Yamagishi M. E., Franco E. H., Krauchenco J. N., Fileto R., Ribeiro A. A., Bezerra G. B., Velludo T. M., Jimenez T. S., Furukawa N., Teshima H., Kitajima K., Bava A., Sarai A., Togawa R. C., Mancini A. L. (2005) The Diamond STING server.
Nucleic Acids Res., 33, W29-35.
Papamichael, E. M., Theodorou, L. G., Bieth, J. G. (2004) Insight into catalytic mechanism of papain-like cysteine proteinases: the case of D158. Appl. Biochem. Biotechnol., 118, 171-175.
Podgorski, I., Sloane, B. F. (2003). Cathepsin B and its role(s) in cancer progression. Biochem. Soc. Symp., 70, 263-276.
Shager, J. (2003). Fiction and Function. Bioinformatics, 19(15), 1934-1936.
Financial support: CAPES
Poster
Classifiers of the protein interactions with their substrate/inhibitors
Authors:
Vera S. Garcia (Unicamp/Embrapa)
Paula Kuser Falcão (Embrapa)
Short Abstract: Basically all biological processes require protein-ligand identifications. One major challenge to biophysics-chemistry is try to predict these interactions. One step towards solving this mystery is analyzing the structural features involved in the complex formation. A good biological model to start these studies is the cystein-protease family.
Long Abstract:
Many fundamental biological processes require protein/ligand identifications to fulfill their biological function. This process is termed biological recognition. One major challenge to biophysics-chemistry is to try to predict these interactions (recognition) between proteins and their ligands (Shager, 2003). Being able to predict the protein recognition methods using structural data would be valuable in understanding the structural-functional relationship and also would facilitate the drug design procedure. One step toward acquiring this knowledge is to analyze all the structural features involved in the complex formation, aiming to understand the structural interactions with their complex pattern of recognition and specificity, and trying to map those features responsible for the enzyme interactions with a specific substrate (Fersht, 1999; Neshich et al., 2005).
For this work, it was established that a good biological model should have: a well described catalytic mechanism; a good number of structurally solved representatives in the Protein Data Bank (Bermann et al., 2000), including the isolated enzyme and in complex with different substrates; being best if found in all live kingdoms and the possibility to raise a grater interest (for example being a drug target). One of the families meeting the criteria mentioned above is the cystein-protease family.
Cystein-protease constitutes an important class of enzymes involved in the formation and hydrolysis of peptide bonds. Besides its obvious vital role in cell homeostasis, it is involved in apoptosis, Parkinson disease, muscle dystrophy, osteoporosis, is a potential target for parasitic treatment, and more (Podgorski & Sloane, 2003; Driessen et al., 1999). It includes plant proteases as papain and actinidin, mammalian lysosomal proteases (cathepsins B, C, H, K, X, etc.) cytosolic calcium activated proteases (calpain), parasitic proteases (cruzain), viral proteases (picornain and adenain) and many others (McGrath et al., 1999; Brocklehurst, 1998). This large family of enzymes has a characteristic molecular topology, and all have in common the catalytic triad Cys, His, Asn (or Asp). Briefly the catalytic mechanism involves the deprotonation of the cystein sulfhydryl by the adjacent histidine, followed by the nucleophilic attack of the sulfur on the peptide carbonyl carbon (Papamichael et al., 2004).
Initially, we selected the protein structures of the members of this family using different data bases. Since the study requires protein structures solved with good resolution, only experimentally determined structures properly validated with resolution 2.9 Å or higher were selected. Both, search methods and manual analysis were used to exclude the “false positive” family members. Since the proteins grouped into families have in common evolutionary relationship and enzymatic activities and the investigation is based, primarily, in structural features, structural analysis were done to create a data bank of similar structures for these family members, forming a structural cluster inside the family. This data bank excluded proteins which the overall structure were easily identified as being different from every other members.
With those structural clusters the alignment experiments were performed and the differences analyzed. In the next step we will perform docking experiments with the structural available substrates. With these we hope to be able to infer some structural parameters that are crucial for the interaction and complex formation.
References:
Berman, H. M., Westbrook, J., Feng, Z., Gilliland, G., Bhat, T. N., Weissig, H., Shindyalov, I. N. & Bourne, P. E (2000). The Protein Data Bank. Nucleic Acids Res. 28, 235-242.
Brocklehurst, K., Watts, A.B., Patel, M, Thomas, E.W. (1998), in Comprehensive biological catalysis, vol. 1, M. Sinnott, ed., Academic Press, 381.
Driessen, C., Bryant, R. A. R., Lennon-Duménil, A. M., Villadangos, J. A., Bryant, P. W., Shi, G. P., Chapman, H. A., Ploegh, H. L. (1999). Cathepsin S controls the trafficking and maturation of MHC class II molecules in dendritic cells. J. Cell Biol., 147, 775-790.
Fersht, A. (1999). Structure and mechanism in protein Science: A Guide to Enzyme Catalysis and Protein Folding. W.H. Freeman and company, New York.
McGrath, M. E. (1999). The lysosomal cysteine proteases. Annu. Rev. Biophys. Biomol. Struct., 28, 181-204.
Neshich G., Borro L C., Higa R. H., Kuser P. R., Yamagishi M. E., Franco E. H., Krauchenco J. N., Fileto R., Ribeiro A. A., Bezerra G. B., Velludo T. M., Jimenez T. S., Furukawa N., Teshima H., Kitajima K., Bava A., Sarai A., Togawa R. C., Mancini A. L. (2005) The Diamond STING server.
Nucleic Acids Res., 33, W29-35.
Papamichael, E. M., Theodorou, L. G., Bieth, J. G. (2004) Insight into catalytic mechanism of papain-like cysteine proteinases: the case of D158. Appl. Biochem. Biotechnol., 118, 171-175.
Podgorski, I., Sloane, B. F. (2003). Cathepsin B and its role(s) in cancer progression. Biochem. Soc. Symp., 70, 263-276.
Shager, J. (2003). Fiction and Function. Bioinformatics, 19(15), 1934-1936.
Financial support: CAPES
Poster
Prediction of functional residues from Plasmodium falciparum plasmepsins: implications in the antimalarial drugs design.
Authors:
Pedro Alberto Valiente Flores (Centro de Estudios de Proteínas (CEP), Facultad de Biología, Universidad de La Habana, Cuba.)
Amaury Pupo Meriño (Centro de Estudios de Proteínas (CEP), Facultad de Biología, Universidad de La Habana, Cuba.)
Tirso Pons Hernández (Centro de Estudios de Proteínas (CEP), Facultad de Biología, Universidad de La Habana, Cuba.)
Pedro G. Pascutti (Instituo de Biofísica Carlos Chagas Filho, Universidade Federal do Rio de Janeiro, Brasil.)
Short Abstract: Sequence and structural analysis of aspartic proteases allowed us to identify the conserved positions M75, V105, T108, Y115, A219, T221, and L287 (plasmepsin II numbering scheme). The predicted positions are in proximity to the inhibitor’s functional groups and they line the protease binding-cavity. This knowledge may contribute to the development of more selective antimalarial drugs.
Long Abstract:
Introduction
Malaria remains one of the world’s biggest health problems; 500 million people get infected with the disease each year, and well over one million dies [1]. This infectious disease is caused by parasites from the genus Plasmodium, which survives in certain types of mosquitoes. The microbe is transmitted to humans where it causes many problems, but most commonly severe, recurring fever attacks. The increasing resistance of malarial parasites, in particular Plasmodium falciparum, to the existing antimalarial drugs has focused the research to the discovery of more selective and potent inhibitors. Plasmepsins play vital roles at various stages of the parasite life cycle, and they are attractive targets for antimalarial drug development.
Here, we present a sequence and structural analysis of aspartic proteases including plasmepsins from different Plasmodium species, and their homologous proteins: cathepsins, pepsin, rennin, and napsin. Based on this analysis we predicted seven conserved positions, and their equivalent residues in plasmepsins I, II, III and IV, lining the binding-cavity and close to the inhibitor’s functional groups. The positions proposed are different from the active-site residues and have not been studied by site-directed mutagenesis. This knowledge would be useful to develop more selective antimalarial drugs.
Material and Methods
We analyzed 73 amino acid sequences, homologous to Plasmodium falciparum plasmepsin I (PlmI), plasmepsin II (PlmII), histoaspartic protease (HAP), and plasmepsin IV (PlmIV). We also compared 13 crystallographic structures (PDB codes: 1lyw, 1bim, 1f04, 1qdm, 1psn, 1ayf, 1sme, 1qs8, 1ls5, 1fkn, 1lyb, 1xdh, 2bjv) from cathepsin D, pepsin, renin, PlmII, and PlmIV. The following web servers were used: PSI-BLAST (http://www.ncbi.nlm.nih.gov/BLAST) for similarity searches; MC-CE (http://cl.sdsc.edu/) for structural superposition; CONSURF (http://consurf.tau.ac.il) to calculate the amino acid conservation; GENBEE (http://www.genebee.msu.ru) to calculate and draw the phylogenetic tree; CASTP (http://sts.bioengr-uic.edu/castp) to identify cavities and calculate their area and volumes; WHAT IF (http://swift.cmbi.kun.nl/WIWWWI/) to calculate contacts between residues of the binding-cavity and functional groups of the inhibitors. Multiple alignments were obtained with the CLUSTALW program [2]. Finally, the multiple alignment was parsed by analyzing gaps, conserved amino acid regions and the secondary structure information extracted from the PDB files.
Results and Discussion
P. falciparum plasmepsins has unique substrate specificity, which results due to variations in residues lining the active site cavities [3]. Earlier mutagenesis studies on PlmI and PlmII concluded that differences in substrate-cleavage specificity depend more on conformational differences from distant sites than on specific active site variation [4]. Other authors have studied the regions that undergo structural deviations accompanying ligand binding in PlmI, PlmII, HAP, and PlmIV [5]. The seven conserved positions proposed here (M75, V105, T108, Y115, A219, T221, and L287: PlmII numbering scheme), differ from those previously studied, and are specific to plasmepsins. In addition, we observed that the inhibitor pepstatin A shows low selectivity against human cathepsin D, which could be explained because some of the established contacts in cathepsin D-pepstatin A complex are also present in the PlmII-pepstatin A, and PlmIV-pepstatin A complexes. Using comparative protein modeling, docking, and molecular dynamic simulations, we are also building three-dimensional models for PlmI and HAP, and their complexes with reported inhibitors whose crystallographic structures are known [3].
References
[1] 2nd annual Biology and Pathology of the Malaria Parasite (BioMarPar) conference, Heidelberg, april 5, 2006.
[2] Thompson JD, Higgins DG, Gibson TJ (1994) Nucleic Acids Res. 22:4673-4680.
[3] Westling J, Cipullo P, Hung S, Saft H, Dame JB, Dunn BM (1999) Protein Sci. 8:2001-2009.
[4] Siripurkpong P, Yuvaniyama J, Wilairat P, Goldberg DE (2002) J Biol Chem. 277:41009-41013.
[5] Bhargavi R, Sastry GM, Murty US, Sastry GN (2005) Int J Biol Macromol. 37:73-84.
Poster
Probabilistic inference of transcription factor concentrations and gene-specific regulatory activities
Authors:
Guido Sanguinetti (Computer Science, Sheffield University)
Neil Lawrence (Computer Science, Sheffield University)
Magnu Rattray (Computer Science, Manchester University)
Short Abstract: We have developed a state-space model for inferring the activity and effective concentration of active transcription factors by integrating microarray data with information about target genes obtained from ChIP-on-chip experiments or motif data. Bayesian parameter estimation using a variational approximation allows us to attach confidence levels to predictions.
Long Abstract:
Quantitative estimation of the regulatory relationship between transcription factors and genes is a fundamental stepping stone when trying to develop models of cellular processes. Recent experimental high-throughput techniques such as Chromatine Immunoprecipitation provide important information about the architecture of the regulatory networks in the cell. However, it is very difficult to measure the concentration levels of transcription factor proteins and determine their regulatory effect on gene transcription. It is therefore an outstanding computational challenge to infer these quantities using gene expression data and network architecture data.
We have developed a probabilistic state-space model that allows inference of both transcription factor protein concentrations and their effect on the transcription rates of each target gene from microarray data. The effect of transcription factors on their target genes is modeled as a weighted linear combination, where the weights are target gene specific. This allows the same transcription factor to enhance or suppress different targets to different extents. The linear nature of the model is a simplification, but allows Bayesian inference to be carried out efficiently on genome-sized data sets. Bayesian inference is useful in this context because the model is highly parameterized, since the number of parameters scales with the number of genes, and therefore not all of the parameters can be estimated with confidence. This provides us with a tool to determine whether or not a target gene is significantly influence by a particular transcription factor. We use variational inference techniques to learn the model parameters in a computationally efficient way and this allows us to perform posterior inference of protein concentrations and regulatory strengths. The probabilistic nature of the model also means that we can associate credibility intervals to our estimates, as well as providing a tool to detect false positives in the network architecture data. MATLAB code and publications are available from http://www.umber.sbs.man.ac.uk/resources/puma.
We demonstrate our model on artificial data and on two yeast data sets in which the network structure has previously been obtained using Chromatine Immunoprecipitation experiments. We show how predictions from our model are consistent with the underlying biology and offer novel quantitative insights into the regulatory structure of the yeast
cell. Our most recent work is on extensions of the model presented here in order to deal with non-additive regulatory effects applied to more specific sub-systems being studied by biological collaborators. We are also developing Bayesian methods for parameter inference in more realistic systems-biology type models which explicitly model the production and decay of RNA in the cell.
Poster
Structural Analysis of the Protein Twitching Motility
Authors:
José G. Jardine (Embrapa Information Technology)
Goran Neshich (Embrapa Information Technology)
Paula R. Kuser-Falcão (Embrapa Information Technology)
Michel E. B.Yamagishi (Embrapa Information Technology)
Stanley R. M. Oliveira (Embrapa Information Technology)
Fábio D. Vieira (Embrapa Information Technology)
Ivan Mazoni (Embrapa Information Technology)
Edgar H. Santos (Embrapa Information Technology)
Juliana M. Papine (Faculdade de Ciências Farmacêuticas-PUCCampinas)
Mariana S. Vieira (Faculdade de Ciências Farmacêuticas-PUCCampinas)
Short Abstract: We have concentrated our effort in understanding some crucial features of protein Twitching Motility of Xylella fastidiosa. Our study is based on the model of this protein, constructed by homology modeling in which we used the crystallographic structure of a Víbrio cholerae homologous protein as a mold.
Long Abstract:
A laboratory consortium in Sao Paulo, Brazil, has published the X. fastidiosa genome, lineage 9a5c, revealing 2,679,305 pairs of bases in the main chromosome and other two plasmids: one with 51,158 and the other with 1,285 pairs of bases. In total, 2,905 genes were identified. The proteome project of X. fastidiosa revealed over 800 expressed proteins, where 112 of them were identified. Some of the 112 proteins are potentially involved in the pathogenic processes of this bacterium in citrus.
The goal of this research is to analyze the tridimensional structure of the PilT protein identified by the proteome, a product of XF1633 gene. Such a protein is associated with adhesion systems and it is a fimbria of type IV, which is supposed to be responsible for the setting of the bacterium in the vascular system of the plant. Our study is based on the model of this protein, constructed by comparative modeling where we used the crystallographic structure of a Víbrio cholerae homologous protein (PDB code: 1p9w) as a template.
Analysis of the model structure using Star Sting software indicates that some amino acids present energy of contacts higher than those on the average. Those residues can have an important role in the stability of this protein when crossreferenced with the residues showing order of cross presence and cross link higher than the usual measures.
In terms of functionality, we are identifying the residue ensemble 19, 21, 64, 67, 82, 84, 99, 181, 183, 185, 200, 206, 208, 231 within the “hot-spots” – the hydrophobic patches at the protein surface. Among those residues, we show that residues 19, 82, 183,206, 208 and 231 have the highest energy of internal contacts found in this protein.
Only the residues 54, 94, 96, 127, 201, 203, 217, 227, 229 satisfy the condition of having a very high order of cross presence (co-location of residues in 3D environment, although they are separated in the primary sequence by the least amino-acids; the order of cross presence/link is a measure of how many times the primary sequence comes back within a given sphere in 3D fold).
We hope that we can put forward a new insight into understanding specificity that PilT protein has associated with adhesion systems supposed to be responsible for the setting of the bacterium in the vascular system of the plant and can help in the design of mutagenesis experiments aimed at elucidating the mechanism of action of the PilT protein associated with adhesion systems and setting of the bacterium.
Poster
Simulating the evolution of metabolic networks: Adaptation to variable environments and its impact on robustness and epistasis
Authors:
Thomas Pfeiffer (Program for Evolutionary Dynamics, Harvard University)
Short Abstract: The relation between phenotype, genotype, and environment is often determined by interactions of cellular compounds in biochemical networks. Mutational robustness and epistasis are key properties to describe this relation. I present an analysis of robustness and epistasis in metabolic networks obtained from simulations of in-silico evolution of metabolism.
Long Abstract:
The relation between the phenotype, genotype, and environment is often determined by the interactions of cellular compounds in biochemical networks. The high complexity of these networks makes it difficult to predict the effect of mutations that affect the individual network compounds. I present an analysis of the patterns of genetic robustness and epistasis in metabolic networks that were obtained from simulations of in-silico evolution of metabolism. To study the impact of environmental robustness on mutational robustness, networks were evolved in constant and in variable environments. In networks that evolved under variable environmental conditions, enzymes emerge that are beneficial in one environment but detrimental in another, leading to selection for regulatory interactions. The evolution of metabolic networks with regulatory interactions is simulated to study the impact of regulation on genetic robustness and epistasis.
Mutational robustness describes how a phenotypic trait is affected by mutations in the genes that are involved in its expression. Explanations for the emergence of mutational robustness fall into three groups: adaptive, congruent, and intrinsic explanations [1]. Adaptive scenarios rely on direct selective advantages of mutational robustness. Mutational robustness may be selected for because it provides a fitness advantage by reducing the effects of deleterious mutations. However, the direct selective forces for mutational robustness are typically weak. Fluctuations in the environment often result in stronger selective forces. In congruent scenarios, mutational robustness is therefore viewed to arise as a byproduct of selection for environmental robustness. Intrinsic explanations for the emergence of mutational robustness are not based on its direct or indirect benefits. Rather, mutational robustness may emerge as an intrinsic property of the evolving system from the way how interactions between genes shape the expression of a trait.
Epistasis quantifies how individual mutations interact, i.e. how much the effect of one mutation depends on the presence of another mutation. Specific cases of epistasic interactions are compensatory mutations (the second mutation buffers the effect of the first mutation) and synthetic lethals (the double mutant is lethal although the corresponding single mutants are viable). Studies on epistatic interactions are receiving increasing interest, because they offer insights into the mechanistic interactions of the mutated compounds [2]. Moreover, they are of relevance for human diseases, because compensatory mutations play a role in the emergence of antibiotic resistance [3], while the identification of synthetic lethal may allow to develop new strategies of treatment against cancer [4]. Finally, epistasic interactions are of fundamental importance for population genetics and evolutionary theory, in particular for theories on the emergence of recombination and sexual reproduction [5]. Similar to mutational robustness, epistatic interactions may be shaped by intrinsic properties of the evolving system as well as by indirect and direct selective advantages. A common definition of epistasis between two mutations is the deviation of the fitness of a double mutant from what would be expected based on the single mutants, i.e., e=wAB-wAwB, where wAB, wA, and wB is the relative fitness of a double mutant, and the correspondingsingle mutants, respectively.
In this study, I analyze patterns of epistasis and mutational robustness in metabolic networks. In a recent study [6], an approach was presented to simulate the the evolution of metabolic networks based on a scenario originally proposed by Kascer and Beeby [7]. In the simulations, metabolic networks evolved to maximize biomass production in a constant environment. The emerging networks had properties similar to natural metabolic networks. Here, I extend this approach to study specific scenarios for the emergence of genetic robustness and epistasis. First, intrinsic patterns of robustness and epistasis are discussed, which arise specifically for metabolic networks. Then, simulations are presented for evolution of metabolic networks in constant and in variable environments. These simulations allow to study the effect of environmental robustness of genetic robustness. For networks that evolve in variable environments enzymes emerge that are beneficial in one but detrimental in another environment. The presence of these enzymes results in selection for regulatory interactions that allow to adjust enzyme activities to the specific environmental conditions. I develop an evolutionary scenario for the emergence of regulatory interactions and study the effect of regulation on mutational robustness and epistatic interactions.
References
1. de Visser, J.A., et al., Perspective: Evolution and detection of genetic robustness. Evolution Int J Org Evolution, 2003. 57(9): p. 1959-72.
2. Tong, A.H., et al., Global mapping of the yeast genetic interaction network. Science, 2004. 303(5659): p. 808-13.
3. Weinreich, D.M., et al., Darwinian evolution can follow only very few mutational paths to fitter proteins. Science, 2006. 312(5770): p. 111-4.
4. Kaelin, W.G., Jr., The concept of synthetic lethality in the context of anticancer therapy. Nat Rev Cancer, 2005. 5(9): p. 689-98.
5. Barton, N.H. and B. Charlesworth, Why sex and recombination? Science, 1998. 281(5385): p. 1986-90.
6. Pfeiffer, T., O.S. Soyer, and S. Bonhoeffer, The evolution of connectivity in metabolic networks. PLoS Biol, 2005. 3(7): p. e228.
7. Kacser, H. and R. Beeby, Evolution of catalytic proteins or on the origin of enzyme species by means of natural selection. J Mol Evol, 1984. 20(1): p. 38-51.
Poster
A Comparative Genomic Analysis of Simple Repeats in Vibrio cholera El Tor
Authors:
Josue Samayoa (Biomolecular Engineering, UC Santa Cruz)
Kevin Karplus (Biomolecular Engineering, UC Santa Cruz)
Fitnat Yildiz (Evironmental Toxicology, UC Santa Cruz)
Short Abstract: We have identified 18 different simple repeats in Vibrio cholerae having significantly different counts than those expected by our null model. We also measured the extent of conservation for these simple repeats across four different Vibrio genomes. We found many instances were the entire repeat was completely conserved.
Long Abstract:
The role of simple repeats as a mechanism for genetic variation has been demonstrated in other organisms, specifically in the genus of bacterium Neisseria.[3, 5, 1, 2] In Neisseria meningitidus several genes involved in host interaction
are thought to be regulated by simple repeats. For example, the expression of the pilin protein is partially regulated by an internal repeated sequence of
guanine (G).[3] Simple repeats cause changes in the expression of genes via slipped-strand mis-pairing. Slipped strand mis-pairing can occur during DNA
replication and transcription. In all cases, the cause is a mis-pairing event between the template nucleic acid and the nascent complementary chain. If the
repeat occurs inside an open reading frame, the slippage event can lead to a shift in reading frame ultimately causing an early termination codon or alternative RNA/protein product. Alternatively, slipped strand mis-pairing can also occur in non-coding regions of the genome. If the repeat is proximal to a transcription factor binding site, the slippage event could alter the distance between the binding site and the transcription start site. Ultimately, this would result in the transcription binding factor being unable to initiate transcription and thus knock out expression of the gene.
The aim of this project was to identify simple repeats in the Vibrio cholerae El Tor genome that are over or under represented and to measure the conser-
vation of those repeats across four different Vibrio genomes (V. vulnificus, V. parahaemolyticus, Vibrio cholerae and V. fischeri). Simple repeats are defined
as those comprised of either single or double nucleotide repeats such as “AAAA” or “ATAT”. This work relies on a null model to calculate the expected number
of occurrences for a given repeat. The null model assumes a background distribution for the number of repeats that follows a binomial distribution. We can
then calculate expected values and variance.
In order to calculate an expected value for the number of a given repeat we need to calculate the probability of that repeat. To do this we use a conditional probability model. For a given word, say “ABCDE”, we calculate the expected number of “ABCDE” via the conditional probability P(ABCDE | BCD). We
approximate P(ABCDE | BCD) using the product of maximum likelihood estimates for P(ABCD | BCD) and P(BCDE | BCD). Our null assumes that the two flanking
residues, "A" and "E", are independent when conditioned on the internal subsequence, "BCD". When this assumption breaks down our observed and expected
values differ and we detect a signal in the form of a Z-score. Finally, we approximate to a normal distribution to convert our Z-score to an
E-value indicating the significance of the observed number of repeats.
Using this null model with all 4 Vibrio genomes, we found 18 different simple repeats having significantly different counts than those expected by the null model. Of the 18 simple-repeats identified, only 4 were over represented. The remaining 14 were all underrepresented. The most under represented simple re-
peat was “TATA”. “TATA” is part of the consensus sequence for the TATA-box binding site which is part of transcription initiation in prokaryotes and eukary-
otes. Although, we find some simple repeats containing “C” or “G”, the list is dominated by simple repeats made of either “A” or “T”. We also measured the
extent of conservation for these simple repeats across the four different genomes. We found many instances were the entire repeat was completely conserved across
all four Vibrio species. We are currently in the process of attempting to identify possible functions for these completely conserved instances of simple repeats.
References
[1] Philip Jordan, Lori A S Snyder, and Nigel J Saunders. Diversity in coding tandem repeats in related Neisseria spp. BMC Microbiol, 3:23, Nov 2003.
[2] Philip W Jordan, Lori A S Snyder, and Nigel J Saunders. Strain-specific differences in Neisseria gonorrhoeae associated with the phase variable gene
repertoire. BMC Microbiol, 5(1):21, Apr 2005.
[3] N J Saunders, A C Jeffries, J F Peden, D W Hood, H Tettelin, R Rappuoli, and E R Moxon. Repeat-associated phase variable genes in the complete genome sequence of Neisseria meningitidis strain MC58. Mol Microbiol,
37(1):207–215, Jul 2000.
[4] G K Schoolnik, M I Voskuil, D Schnappinger, F H Yildiz, K Meibom, N A Dolganov, M A Wilson, and K H Chong. Whole genome DNA microarray expression analysis of biofilm development by Vibrio cholerae O1 E1 Tor.
Methods Enzymol, 336:3–18, 2001.
[5] L A Snyder, S A Butcher, and N J Saunders. Comparative whole-genome analyses reveal over 100 putative phase-variable genes in the pathogenic
Neisseria spp. Microbiology, 147(Pt 8):2321–2332, Aug 2001.
Poster
Evolution in populations with multiple recombination strategies
Authors:
Oleg Rokhlenko (Computer Science Dept., Technion, Israel)
Ydo Wexler (Computer Science Dept., Technion, Israel)
Short Abstract: Recombination is a primary evolutionary mechanism.
Recently, several evolutionary models suggested a fitness associated recombination (FAR) scheme.
In this study we extend the FAR concept to a continuum of continuous strategies, and show that using multiple recombination strategies is advantageous in terms of the induced fitness.
Long Abstract:
Evolution is the process by which successive generations of organisms change. It is driven by the fact that all organisms have to struggle to survive long enough to reproduce in a competitive environment. Many evolving molecular systems can be described by a rugged fitness landscape, in which ‘peaks’ of superior genetic combinations are separated by ‘valleys’ of inferior combinations (Wright, 1931). In such complex genetic systems the evolutionary pressure promotes the creation of superior combinations.
The tools that nature has available to evolve are limited. Point mutation and recombination provide almost the only mechanisms to introduce genetic variation. While point mutation is an important mechanism, most of the genetic variation and genetic shift is the outcome of recombination which is the primary evolutionary force.
Recombination is the process that forms new combinations of genes on a chromosome as a result of crossing over. Although the evolutionary function of recombination is not fully understood many research works support its long-term effect on the population level (Crow and Kimura 1999, Muller 1964). These works investigate the relationship between the recombination and fitness of an organism, defined as the organism's ability to survive and reproduce in a particular environment. Others, like (Redfield 1988) propose that recombination is negatively associated with fitness, breaking down unfit combinations of genes with a higher probability than fitter ones.
Recently, evolutionary models with different association schemes were proposed. Some of these models were suggested in the scope of evolutionary algorithms, among which we mention the followings: Davis (1989) suggested a method that chooses operators according to their performance as these are reflected in the population. In a work by De-Jong (1975) the SEGA method is presented, which considers a simple elitism of preserving the best current solution in the population such that it appears in the next generation. Biologically speaking, the fittest individual reproduces asexually, thus incurring no crossover event. Thierens (1997) discusses ways to govern the selective pressure by means of dividing the population into sub-groups, named families, in which evolution and elitism selection occurs separately from the rest of the population. Finally, Beker and Hadany (2002) extended De-Jong's idea to an algorithm called gamma-GEGA which prohibits crossover to the top fraction gamma<1 of the population. Although this series of papers show good results, the adaptations suggested there are limited and the correlation between fitness and recombination follows a hard-coded scheme. In addition, algorithms such as gamma-GEGA may lead to premature convergence of the population when the parameter gamma is set too high, and on the other hand, algorithms such as SEGA and the one by Thierens, can often yield high variance in the population and thus poor fitness.
We generalize the elitism concept to a much wider scope of continuous recombination strategies, where the set of all such strategies. This extension allows varying adaptation levels, and we show that a mixture of recombination strategies can be used to present a self-adaptive design that overcome the drawbacks of previously suggested methods.
To be able to quantify the individual's adaptation we give each distinct genome a score based on a pre-defined function and use the term of relative fitness of an individual $i$ with score $s^i$ to be the fraction of population with score lower than or equal to $s^i$. To account for populations with multiple strategies, we consider a strategy gene which exists in every individual and is linked to a certain extent with the rest of the chromosome. This gene determines the recombination strategy of the individual, by which its recombination rate is determined. For fitness associated recombination (FAR) strategies the recombination rate of an individual is reversely proportional to the individual's relative fitness in the population. In particular, one class of strategies that generalizes the strategies used in SEGA and $\gamma$-GEGA algorithms is the set of shifted sigmoid functions.
The primary known disadvantage of FAR strategies is that they increase the probability of premature convergence of evolution to a local optimum. In nature, this is kind of convergence can occur as a result of environmental changes that affect the level of adaptation of population to the new environment. Although adaptive strategies showed to be useful in evolutionary convergence to close to optimal populations in some cases, they have also been proven to lead to sub-optimal convergence in others, mostly due to an excessive selection pressure. The main drawback of these single-strategy scenarios, which has a direct negative effect on their performance, is that the function that governs recombination in the population is fixed. A bi-level optimization scheme, which let evolutionary forces adjust the self-adaptation behavior, overcomes this problem.
Multi-strategy scenarios combine several FAR strategies simultaneously and provide means of evolutionary contest between them. Such competitions were observed in several biological systems. We suggest that multi-strategy scenarios where the association function is polymorphic, are considerably advantageous in terms of population's fitness and adaptation to environmental changes. It has been shown that the domination prospects of the strategies vary not in accordance with the induced fitness on population (Wexler and Rokhlenko 2006). Therefore, a careful selection of strategies is needed. This concern is primarily motivated by the sub-optimality induced on the population by prisoner's dilemma scenarios (Smith 1982).
We ran simulations that mimic evolution in a large population. The genetic information, including the recombination strategy function, followed the one used in (Wexler and Rokhlenko 2006). Through a simulation of 10,000 generations, the fitness of population when using multiple strategies was significantly higher than the fitness when using a single strategy.
In addition, when accounting for complex environmental changes that negatively affect the population's fitness, we observe a fast convergence to a relatively low fitness when a single strategy is used in the simulation. This is most apparent when compared to the slower convergence of multi-strategy populations to higher fitness, overcoming the local changes in the fitness landscape.
Poster
Design of a Combinatorial DNA Microarray for Protein-DNA Interaction Studies
Authors:
Julian Mintseris (Bioinformatics Program, Boston University)
Michael B. Eisen (Department of Molecular and Cell Biology, University of California, Berkeley)
Short Abstract: We present an algorithmic approach to experimental design of a universal microarray that allows for testing full specificity of a transcription factor binding to all possible DNA binding sites of a given length with optimally efficient use of the array.
Long Abstract:
With the human and many other genome sequences complete or nearing completion, we are approaching the goal of identifying all the protein coding genes. However, to understand the function of these genes in different physiological contexts, it is important to understand how their expression is regulated. Mechanisms of gene regulation are varied and complex and unraveling them will require a combination of approaches.[1, 2] Having a catalog of all the transcription factors and being able to characterize their binding specificity at cis-regulatory sites would provide a fruitful starting point.
Recent advances in chromatin immunoprecipitation methods have led to large-scale efforts to determine all protein-DNA binding events in yeast[3, 4] but scaling up such methods for mammalian genomes may prove difficult. Protein-binding microarrays (PBM), initially developed on a small scale by Bulyk et al.[5] showed promise in identifying transcription factor binding specificity with high accuracy and was recently successfully scaled up for the yeast genome by using PBMs with all known yeast intergenic regions. Although an exciting advance in the field, current design of PBMs still leaves some room for uncertainty because some of the intergenic regions may be too long to pinpoint the binding sites with high accuracy. Scaling this method up to mammalian genomes would also require designs spanning multiple arrays, with a new design for each genome.
Given the recent technological innovations allowing programmable synthesis of microarrays as well as new techniques to make the arrays double-stranded[6], here we focus on optimizing experimental design. We present an graph-theoretic approach to experimental design of a microarray that allows for testing full specificity of a transcription factor binding to all possible DNA binding sites of a given length with optimally efficient use of the array. This design is universal, works for any factor that binds a sequence motif and is not species-specific. Furthermore, simulation results show that data produced with the designed arrays is easier to analyze and would result in more precise identification of binding sites. Such a design will prove useful for transcription factor binding site identification and other biological problems.
1. Davidson EH, Rast JP, Oliveri P, Ransick A, Calestani C, Yuh CH, Minokawa T, Amore G, Hinman V, Arenas-Mena C, et al: A genomic regulatory network for development. Science 2002, 295:1669-1678.
2. Bolouri H, Davidson EH: Modeling transcriptional regulatory networks. Bioessays 2002, 24:1118-1129.
3. Lee TI, Rinaldi NJ, Robert F, Odom DT, Bar-Joseph Z, Gerber GK, Hannett NM, Harbison CT, Thompson CM, Simon I, et al: Transcriptional regulatory networks in Saccharomyces cerevisiae. Science 2002, 298:799-804.
4. Harbison CT, Gordon DB, Lee TI, Rinaldi NJ, Macisaac KD, Danford TW, Hannett NM, Tagne JB, Reynolds DB, Yoo J, et al: Transcriptional regulatory code of a eukaryotic genome. Nature 2004, 431:99-104.
5. Bulyk ML, Gentalen E, Lockhart DJ, Church GM: Quantifying DNA-protein interactions by double-stranded DNA arrays. Nat Biotechnol 1999, 17:573-577.
6. Warren CL, Kratochvil NC, Hauschild KE, Foister S, Brezinski ML, Dervan PB, Phillips GN, Jr., Ansari AZ: Defining the sequence-recognition profile of DNA-binding molecules. Proc Natl Acad Sci U S A 2006, 103:867-872.
Poster
Stem Kernels for RNA Sequence Analyses
Authors:
Yasubumi Sakakibara (Keio University)
Kengo Sato (JBIC)
Short Abstract: We propose a novel kernel function, called stem kernels, for detections of functional RNA sequences using SVM.
The stem kernel exhibits a good performance of discriminations even for weaker homologous RNA sequences.
Further, we apply our stem kernel to detect remote homology RNA family in terms of secondary structures.
Long Abstract:
Analyses and detections of functional RNAs are important current topics on both molecular biology and bioinformatics researches.
Since there are a rapidly growing number of RNA sequences, structures and families, computational methods for finding non-protein-coding RNA regions on the genome have much attention to be investigated.
Compared with genefinding problems for protein-coding regions, computationally identifying non-coding RNA regions is essentially harder because non-coding RNA sequences do not have strong statistical signals, and there are as yet no general finding algorithms.
In RNA sequence analyses, it is well known that the secondary structures which RNAs take forms in cell are important features for modeling and detecting RNA sequences.
The folding of an RNA sequence into a functional molecule is largely governed by the formation of the standard Watson-Crick base pairs A-U and C-G as well as the wobble pairs G-U.
Such base pairs constitute the so-called biological palindromes in genome.
The secondary structures of RNAs are in general composed of stems, hairpins, bulges, interior loops, and multi-branches.
A stem is a double stranded (paired) structure region of base-pair stacks.
A hairpin loop is where RNA folds back on itself.
To capture such secondary structure features, stochastic context-free grammars (SCFGs) for RNAs have been proposed and succeeded to model typical secondary structures of RNAs and are used for structural alignments of RNA sequences.
However, one serious drawback of SCFG methods is that the method requires a prior knowledge of a typical secondary structure known for a target RNA family to design the grammars.
Further, stochastic models such as SCFGs and HMMs have some limitations to discriminate member sequences of an RNA family from non-members only according to the probabilistic scores.
Hence, we need more strong discriminative methods to detect and find non-coding RNA sequences.
Recently, the support vector machine (SVM) and kernel function techniques are actively studied and proposed to solve various problems in bioinformatics.
SVMs are trained from positive and negative samples and have strong and accurate discrimination abilities, and hence are more adequate for the discrimination tasks.
For protein sequence analyses, the string kernels have been proposed for the use of SVMs to classify a protein family.
The string kernels have also been proved to work for remote homology detections, that is a superfamily, of protein sequences.
In this paper, we propose a novel kernel function, called stem kernel, that extends the string kernel to measuring the similarity of two RNA sequences from the viewpoint of secondary structures.
We consider the similarity features defined by all possible common base-pairs and stem structures of arbitrary lengths including pseudoknots between two RNA sequences.
The proposed stem kernel calculates the inner product of two vectors in the feature space from two RNA sequences.
That is, the more stem structures two RNA sequences have in common, the more similar they are.
Further, our stem kernel does not assume any prior knowledge about secondary structures of a target RNA family.
We show several experimental results of our stem kernel method for discrimination tasks.
In the tRNA experiments, the stem kernel exhibits a good performance of discriminations even for weaker homologous RNA sequences, while the string kernel is comparable for high similarity sequences and the discrimination performances significantly go down
for weak homologous RNA sequences.
We also use Kernel Principal Component Analysis (KPCA) to measure the classification and separation abilities of each kernel function from a mixed RNA sequence data of three different RNA families.
Further, we demonstrate some potential ability to apply our stem kernel to detect remote homology RNA family in terms of secondary structures, because the string kernel has been proved to work for remote homology detections of protein sequences.
We attempt to detect Tymo_tRNA-like sequences as remote homologies of tRNAs by using SVMs trained from the positive and negative samples of tRNA sequences.
Poster
Cell System Markup Language CSML 3.0 - Basic Concept and Specification -
Authors:
Masao Nagasaki (University of Tokyo)
Atsuhi Doi (University of Tokyo)
Hiroshi Matsuno (Yamaguchi University)
Satoru Miyano (University of Tokyo)
Short Abstract: We developed an XML format Cell System Markup Language CSML 3.0 (http://www.csml.org/). It includes SBML 2.0 and CellML 1.0 as subsets. We also developed programs for converting SBML and CellML to CSML 3.0 automatically. Cell Illustrator 3.0 supports CSML 3.0.
Long Abstract:
We developed an XML format named Cell System Markup Language CSML 3.0 (http://www.csml.org/). Some XML formats are proposed to be a standard format for biopathways. However, all formats provide only a partial solution for the storage and integration of biological data. The aim of CSML 3.0 is to create a really usable XML format for visualizing, modeling and simulating biopathways. For many cases, in vivo/vitro biological experimental results and in silico analyzed results are useful information for biopathway analysis. A successful application is Cytoscape, which can combine in vivo/vitro and in silico analyses into one graphical network. The core application supports a text-based and a GML formats. Plugins for importing XML format are developed. However, the functionality is limited. In addition, the application just visualizes the biopathway related data but dynamic simulation part is missing.
Other XML formats, SBML 2.0 and CellML 1.0 are proposed and developed for dynamic simulation. These formats have become popular for chemical reactions and many applications support them as data exchanging formats. However, these formats do not define any graphical elements, which causes a difficulty to be a powerful data exchange format among biopathway applications. Here, CSML 3.0 is proposed as an integrated/a unified data exchange format which covers widely used data formats and applications, e.g. CellML 1.0, SBML 2.0, BioPAX, and Cytoscape. In CSML 1.9 and CSML 2.0, the main focus was to support Hybrid Functional Petri net (HFPN) based visualization and simulation. CSML 3.0 has focused on Hybrid Functional Petri net with extension (HFPNe) architecture, extended HFPN with object notion, for more advanced biopathway representation. In short, objects that construct biopathways are treated as "generic entity" of HFPNe architecture and any relations among objects are treated as "generic process" on the HFPNe architecture. The format consists of the following and the details of CSML 3.0 is available form http://www.csml.org/:
(i) A model with entity set and relation set on HFPNe.
(ii) Submodels of (i).
(iii) Views for each submodels in (ii).
We also developed automatic conversion programs which convert SBML 2.0 to CSML 3.0 and CellML 1.0 to CSML 3.0 automatically. Cell Illustrator 3.0 fully supports CSML 3.0 as its base XML. Thus every model in SBML 2.0 and CellML 1.0 can be executable on Cell Illustrator 3.0.
Poster
Serine Proteases analysis based on phylogenetic trees constructed from the sequence and structure alignments
Authors:
Cristina Ribeiro (UFMG)
Paula Kuser (EMBRAPA CNPTIA)
Goran Neshich (EMBRAPA CNPTIA)
Marcelo Santoro (UFMG)
Short Abstract: We compared two approaches to construct phylogenetic trees from a set of serine proteases with deciphered 3D_structure. The first approach: sequence alignment generates a phylogenetic tree; the seccond approach is based on a sequence alignment strictly following the structure alignment. The differences b/w the two is discussed.
Long Abstract:
Almost one-third of all proteases can be classified as serine proteases, named for the nucleophilic Ser residue at the active site. This mechanistic class was originally distinguished by the presence of the Asp-His-Ser “charge relay” system or “catalytic triad”.The Asp-His-Ser triad can be found in at least four different structural contexts, indicating that this catalytic machinery has evolved on at least four separate occasions. These four clans of serine proteases are typified by chymotrypsin, subtilisin, carboxypeptidase Y, and Clp protease (MEROPS nomenclature). Serine proteases with the classic Asp-His-Ser triad are the largest class of proteases, including digestive enzymes with minimal especificity and processing enzymes with exquisite substrate recognition. These proteases can be found in eukaryotes, prokaryotes, archae, and viruses. Chymotrypsin-like proteases are involved in many critical physiological processes, including digestion, hemostasis, apoptosis, signal transduction, reproduction, and the immune response.
Objectives:
The main objective of this work is to compare two different approaches to construct phylogenetic trees from a set of non-redundant serine proteases with deciphered 3D structure. The first one the sequence alignment generates a phylogenetic tree, and the other one is based on a sequence alignment strictly following the structure alignment. The differences b/w the two is discussed.
Work interest
To our knowledge, there are very few papers where the authors have tried to generate phylogenetic approaches from both sequence alignment and structural homology and compared them in a systematic way to evaluate the results.
Methodology:
Database construction:
The first step to the construction of a non-redundant database of crystallized serine proteases was a query for all serine proteases in Protein Data Bank (http://www.rcsb.org/pdb/Welcome.do), followed by extensive manual curation to remove duplicates and single-amino acid mutants. After that we removed all sequences that didn’t have the classical two barrel folding of serine proteases and the subtilisin subfamily, since it has a great sequence divergence from the traditional members of this family. After that we ended up with the list of 82 non-redundant serine protease structures ready for further analysis.
3D conserved residues based on structural alignment
The PrISM (Protein Informatics System for Modeling, Yang, 1999) is a protein analysis tool that allows, among other things, for a structural alignment. We analyzed our set of non-redundant serine proteases with PrISM software, generating a subset of the original full-length sequences containing only the regions structurally shared by them.
Resulting alignments (the sequence and structure based one) are then used to generate the two phylogenetic trees and those are discussed in details in this work.
Yang AS, Honig B. Sequence to structure alignment in comparative modeling using PrISM. Proteins. 1999;Suppl 3:66-72.
Poster
InterMine - a biological data warehouse system
Authors:
R. Smith (Department of Genetics, Cambridge University)
F. Guillier (Department of Genetics, Cambridge University)
H. Janssens (Department of Genetics, Cambridge University)
W. Ji (Department of Genetics, Cambridge University)
R. Lyne (Department of Genetics, Cambridge University)
P. McLaren (Department of Genetics, Cambridge University)
T. Riley (Department of Genetics, Cambridge University)
K. Rutherford (Department of Genetics, Cambridge University)
M. Wakeling (Department of Genetics, Cambridge University)
X. Watkins (Department of Genetics, Cambridge University)
F. Reisinger (Department of Genetics, Cambridge University)
G. Micklem (Department of Genetics, Cambridge University)
Short Abstract: InterMine (www.intermine.org) is an open source data warehouse system developed to enable FlyMine (www.flymine.org). It provides simple, configurable integration of data from many common biological formats and a framework to add other sources. A sophisticted web application allows users to create complex queries, run pre-defined queries and operate on lists.
Long Abstract:
InterMine (www.intermine.org) is an open source data warehouse system developed to enable FlyMine (www.flymine.org). It is able to integrate several common biological formats and provides a framework for adding other sources. Data is imported into a query-optimised data warehouse and is accessible via a powerful web interface.
Data from Ensembl, UniProt, InterPro, InParanoid (orthologues), PSI (protein interactions) and GO annotation can be imported simply by specifying the organism/experiment. GFF3, FASTA and MAGE can be added with some additional configuration. InterMine also provides a framework for adding new data sources. The data model (the core of which is derived from the sequence ontology) is defined by an XML file and is easily extendable. All model specific parts of the system are generated from this XML so it is easy to incorporate new types of data.
A model-independent web application is designed to be a powerful query tool giving users the ability to build complex queries and not be constrained by fill-in-the-blanks forms. It is also possible to create and publish 'templates' to make common queries easy to run. All queries can be executed on lists of data, either uploaded by users or created from results of other queries. Queries and lists can be saved between sessions and shared with other users. The web application provides a platform for integration of visualisation and analysis tools, GBrowse and Cytoscape are already available for viewing genome annotation and protein interaction networks.
A generic query optimisation system means that InterMine can turn any model into a query optimised data warehouse. Incoming queries are automatically analysed and re-written to use pre-computed tables in the underlying database. Generation of pre-computed tables is configurable, thus the system can be optimised for any user query and performance can be adapted to actual usage. Template queries are pre-computed once created so will always return results fast.
InterMine is able to operate on any data model so could be used to provide a data warehouse and query interface for an existing data management system. Extensive use of automatic code generation means little development is required.
InterMine is written in Java with a Struts/JSP web application, PostgreSQL is used as the underlying relational database. The system has been in development for over three years by a team of software engineers and biologists. All software is freely available under the LGPL license.
Poster
Identification of replication origins in archaea using a moving window model along the double strands
Authors:
Yonghong Wang (Department of Physics, Tianjin University, Tianjin, 300072, China; School of Computer Science and I)
Xiaolei Liu (Department of Physics, Tianjin University, Tianjin, 300072, China)
Short Abstract: A new method has been developed to identify replication origins in prokaryotic genomes based on a moving-window model along double strands. Two curves corresponding to base distribution in two DNA strands, intersect at replication origins. The approach can be used to identify single and multiple replication origins in archaea.
Long Abstract:
The DNA replication is one of the fundamental steps in cell division process. The study on replication origin is very important to understand replication mechanism. In the three domains of life, replication process in bacteria starts from a single replication origin. The process in eukarya starts from multiple replication. In archaea, replication process starts from single or multiple replication origins. Almost all proteins involved in archaea replication have their homologous counterparts in eukarya [1-3]. Therefore, it is very important to analyze replication origins of archaea for understanding the replication mechanism in both bacteria and eukarya. With the rapid growth of completely sequenced prokaryotic genomes, identification of replication origins by computational methods becomes more and more important. In the past few years, computational approaches have been applied for identification of replication origin in prokaryotic genomes. Lobry used the DNA walk method to detect the replication origins [4]. Since then, several algorithms have been proposed for the identification of replication origins in prokaryotic genomes [5-9]. In general, the methods are mainly applied for identification of single origins. Recently, the Z curve method has been successfully applied in the identification of the single and multiple replication origins in archaea [10, 11]. With this method, prediction is mainly based on the asymmetry of compositions and oligomers between the leading strands and the lagging strands. Here a simple method is proposed for identification of replication origins in prokaryotic genomes. The method consists of two parts: a moving window model and the wavelet de-nosing technique. A sliding window is moved along the entire sequences. The contents of bases G and T in double strands are respectively calculated within a window. Two curves are formed to describe bases G and T distribution respectively along the main strand and the complementary strand. Taking the asymmetrical distribution of bases G and T around replication origins into account, the two curves intersect at some points close to the replication origins and termini. Moving one curve upwards or downwards makes the two curves completely symmetrical. The locations of the intersection points correspond to the replication origins and termini accurately. As the local composition fluctuates along DNA sequences, wavelet de-noising technique is used to reduce the noise signals. Using the present method, two archaea genomes have been analyzed, which are Halobacterium sp. NRC-1 and Pyrococcus abyssi GE5. In the archaea, the replication origins have been experimentally proven at the sequence level. Their genomic sequences were downloaded from http://www.ncbi.nlm.nih.gov. In order to determine the replication origins, a large window is selected, such as 100kbp. The window moves along the double strands at 10 bp intervals. The wavelet de-noising technique includes two steps: wavelet decomposition and reconstruction. The Haar wavelet is applied to decompose the signal sequences until the 14th level. The signal sequences are reconstructed using the original low frequency coefficients and the high frequency coefficients modified by a threshold Cj. Actually the reconstructed signals are the same even though the signals are decomposed into more than 14 levels. Using the predicted criteria, replication origins for the two archaea are identified. For Pyrococcus abyssi, the two curves intersect at two points, the predicted replication origin lies at the position 1230kb. The prediction is consistent with the experiment evidence [12]. The two curves for Halobacterium sp. NRC-1 interact at four points, two replication origins are predicted. They lie at 910160bp and 1806200 bp, respectively. However, experiment evidence shows that Halobacterium sp. NRC-1 only has one replication origin, located in the range of 1807930-1809486bp. One of the prediction locations is consistent with the range. For the other location, Zcurve method also has provided similar prediction [11]. The characteristics around the location are consistent with those of most known replication origins. For prokaryotic organisms, the single replication origins can be successfully identified by computational methods. However, identification of multiple replication origins still remains a challenge. Clarification of replication mechanism can provide insight into the understanding of replication mechanism of eukarya.
References
[1] D. R. Edgell and W. F. Doolitte. Archaea and the origins of DNA replication proteins. Cell 89: 995-998, 1997.
[2] I. K. Cann and Y. Ishino. Archaeal DNA replication identifying the pieces to solve a puzzle. Genetics 152: 1249-1267, 1999.
[3] K. Bohlke, F. M. Pisani, M. Rosso, and G. Antranikian. Archaeal DNA replication spotlight on a rapidly moving field. Extremophiles 6: 1-14, 2002.
[4] J. R. Lobry. A simple vectorial representation of DNA sequences for detection of replication origins in bacteria. Biochimie 78: 323-326, 1996.
[5] A. Grigoriev. Analyzing genomes with cumulative skew diagrams. Nucleic Acids Res. 26: 2286-2290, 1998.
[6] J. Mrazek and S. Karlin. Strand compositional asymmetry in bacterial and large viral genomes. Proc. Natl. Acad. Sci. USA 95: 3720-3725, 1998.
[7] M. J. Mclean, K. H. Wolfe, and K. M. Devine. Base composition skew, replication orientation, and gene orientation in 12 prokaryote genomes. J. Mol. Evol. 47: 691-696, 1998.
[8] S. L. Salzberg, A. R. Kerlavage, and J. F. Tomb. Skewed oligomers and origins of replication. Gene 217: 57-67, 1998.
[9] P. Lopez, H. Philippe, H. Myllykallio, and P. Forterre. Identification of putative chromosomal origins of replication in archaea. Mol. Microbiol. 32: 883-886, 1999.
[10] R. Zhang and C.T. Zhang. Single replication origin of the archaeon Methanosarcina mazei revealed by the Z curve method. Biochem. Biophys. Res. Commun. 297: 396-400, 2002.
[11] R. Zhang and C. T. Zhang. Multiple replication origins of the archaeon Halobacterium species NRC-1. Biochem. Biophys. Res. Commun. 302: 728-734, 2003..
[12] W. V. Myllykallio, P. Lopez, P. Lopez-Garcia, R. Heilig, W. Saurin, Y. Zivanovic, H. Philippe, and P. Forteere. Bacterial mode of replication with eukaryotic-like machinery in a hyperthermophilic archaeon. Science 288: 2212-2215, 2000.
Poster
Corresponding human and mouse genomic regions unalignable in primary sequence
contain thousands conserved RNA structures
Authors:
Elfar Torarinsson (The Royal Veterinary and Agricultural University)
Milena Sawera (The Royal Veterinary and Agricultural University)
Jakob H. Havgaard (The Royal Veterinary and Agricultural University)
Merete Fredholm (The Royal Veterinary and Agricultural University)
Jan Gorodkin (The Royal Veterinary and Agricultural University)
Short Abstract: Comparative genomics has been limited by the requirement of a good alignment in
the primary sequences. We scanned corresponding human mouse regions unalignble
in primary sequence and estimate 1800 such human-mouse regions to contain
common RNA structures. RT-PCR and northern blots confirm coexpression in mouse
overlapping human transfrags.
Long Abstract:
A major limitation in comparative genomics is that it requires a good alignment of the primary genomic sequences. If available, the use of multiple organisms can to some extend compensate for this. However, when searching for non-coding RNAs in corresponding genomic regions with sequence conservation typically less than 50% the desired approach should take both sequence and structure conservation into account. Here, we screened the approximately 100,000 human-mouse regions that neighbor corresponding alignable regions, but are unalignable in their primary sequence themselves for local RNA structural alignments using FOLDALIGN [1]. From the screen, we estimate that there are approximately 1800 such human-mouse regions containing common RNA structures. Furthermore, we find that the high scoring candidates are twice as likely to be be found in regions overlapped by transcribed fragments (transfrags) in human [2] than regions that are not overlapped. Co-expression between predicted candidates in human and mouse was verified by conducting expression studies by RT-PCR and Northern blotting on mouse candidates, which overlap with transfrags on human chromosome 20. For expression using RT-PCR 32 out of 36 candidates was confirmed, whereas for Northern blots we confirmed 4 out of 12 candidates. In addition, many RT-PCR results indicate differential expression across different tissues. Hence, our findings suggest that there are corresponding regions between human and mouse, which contain expressed non-coding RNA sequences unalignable in primary sequence. Details are described in [3] and a database for ncRNA candidates can be accessed at http://genome.kvl.dk/resources/hm_ncrna_scan.
References
[1] Havgaard, J.H., Lyngsø, R.B., Stormo, G.D, and Gorodkin, J., Bioinformatics 21: 1815-24, 2005 (http://foldalign.kvl.dk)
[2] Cheng, J., Kapranov, P., Drenkow, J., Dike, S., Brubaker, S., Patel, S., Long, J., Stern, D., Tammana, H., Helt, G., Sementchenko, V., Piccolboni, A., Bekiranov, S., Bailey, D.K., Ganesh, M., Ghosh, S., Bell, I., Gerhard, D.S., Gingeras, T.R., Science 308: 1149-54, 2005.
[3] Torarinsson E., Sawera, M., Havgaard, J. H., Fredholm, M. and Gorodkin, J., Genome Research, Accepted.
Poster
Mr
Authors:
Gasper Tkacik (Princeton University)
Elad Schneidman (Princeton University)
William Bialek (Princeton University)
Short Abstract: We present a maximum-entropy based approach to the reconstruction of interaction networks and apply it to the published data on the signaling cascade in primary human immune system cells1. The approach assumes that the interaction graph can be fully connected, but that each single interaction is ‘low-order’, i.e. occurring only between pairs or perhaps triplets of elements.
Long Abstract:
In the case of single-cell simultaneous measurements of activation levels of interacting proteins we model the data as being drawn from an underlying distribution. Lacking enough samples to estimate it directly, we make the following simplifying assumption: the distribution is as random as possible (hence maximum-entropy) while still preserving all pairwise correlations observed in the experiment. The solution to this problem is equivalent to a generalized Ising model in statistical physics and amounts to finding the corresponding pairwise interaction potential Vij(xi,xj), which fully describes the ‘interaction network’. This procedure has been successfully applied to modeling of the simultaneous spiking activity in the neurons of vertebrate retina2. Here we have extended it to utilize data that combines sets of single-cell measurements taken at different external conditions.
We present a systematic way of increasing the resolution of the method as more data becomes available. For representations in which each protein activation level can be described by two or three discrete states, we find that pairwise interactions capture most of the structure in the analyzed signaling network, and, in the isolated place where they fail, they indicate which higher order interaction terms are missing. We discuss the performance of the maximum entropy reconstruction when a significant number of interacting nodes remains inaccessible to measurements.
1. Sachs K, Perez O, Pe'er D et al. Causal Protein-Signaling Networks Derived from Multiparameter Single-Cell Data. Science 308, 523-29 (2005).
2. Schneidman E et al. Weak pairwise correlations imply strongly correlated network states in neural population. Nature 440, 1007-12 (2006).
Poster
Independent Re-annotation of Affymetrix GeneChips Resulting In Reliable And Expanded Gene Association
Authors:
Yong Yue (Integrative Biology, Eli Lilly and Company)
Ramneek Gupta (Bioinformatics Development, Lilly Systems Biology)
Mahesh Kumar (Bioinformatics Development, Lilly Systems Biology)
Adam West (Integrative Biology, Eli Lilly and Company)
John Calley (Integrative Biology, Eli Lilly and Company)
Short Abstract: Re-annotation of Affymetrix GeneChips by independent methods has resulted in increased reliability and stability of annotation with slightly improved coverage over human chips and a larger gain in coverage over rat chips. Using similar methods on aCGH and siRNA platforms provides consistent annotation that is important for data integration.
Long Abstract:
Affymetrix GeneChips are used widely in transcript profiling, particularly for applied research in the pharmaceutical industry where high throughput and standardization is critical. Gene annotation of the microarrays provided by Affymetrix known as NetAffx, however, does not keep pace with growing knowledge as indicated by a number of gaps uncovered by recent studies. Re-annotation of the Affymetrix chips has been carried out by various laboratories, but many share in large measure the same gene association as NetAffx or depend on Unigene clustering. We have developed an integrated process to re-annotate human HG-U133 Plus2, mouse MOE430A/B, and rat RAE230A/B chips, on the basis of genome mapping and RefSeq transcript matching using probe target sequences provided by Affymetrix. We found that 5067, 2297, and 1824 probe sets, respectively, of the three chips were on the reverse strand of known genes, to which the transcripts will not hybridize. We have also identified 4299, 3052, and 1082 probe sets in these chips as ambiguous, as they each match two or more genes. Removal of the reverse-stranded, ambiguous, and other undesirable probe sets significantly increased the quality of gene association. Based on genome and transcript mapping, the annotated probe sets have been assigned one of the five categories: 1) both genome and transcript, 2) transcript only, 3) genome only, but with EST stack, 4) genome only, without stack, and 5) Unigene only. Probe sets in 4th category, with lower signal intensity or presence call rate from 284 human chip experiments, likely represent either rare transcripts or non-transcribed genomic sequences. Judging from the stability of gene association, our re-annotation is markedly more reliable than those from NetAffx in each of the five annotation categories. With the quality improvements in gene association, there is little change (less than 1%) from NetAffx in the numbers of annotated probe sets in the human and mouse chips, whereas the gene annotation gained by 9.9% in the rat chips.
By applying similar methods to annotate reagents across multiple data platforms, the consistent annotation helps our scientists in the integration of data from aCGH, siRNA and multiple expression platforms.
Poster
Identifying persistent stress response modules in yeast
Authors:
Ydo Wexler (Computer Science Dept., Technion, Israel)
Oleg Rokhlenko (Computer Science Dept., Technion, Israel)
Zohar Yakhini (Agilent Laboratories)
Short Abstract: We study stress response mechanisms in Saccharomyces
cerevisiae by identifying genes that, according to very
stringent criteria, have persistent co-expression under a variety
of stress conditions.
We identify persistent cliques with enriched function as well as enriched
regulation by a small number of TFs.
Long Abstract:
The mechanisms that control and regulate cellular processes in living organisms are complex and involve several types of control, monitoring and activation/de-activation modules. Model systems, such as S. cerevisiae play an important role in this study. Some of the components of the mechanisms that control gene expression in yeast are, known and can even be reproduced or manipulated in the laboratory.
Living organisms and the survival of cells critically depend on their ability to sense alterations in the environment and to then respond promptly and adequately to new situations through the induction of protective stress responses. Yeast, as well as other organisms, employ a concerted response to external stress conditions. The genomics of stress response in S. cerevisiae has been extensively studied using a variety of experimental and computational techniques. Ruis and Schuller (1995) review stress response mechanisms in Saccharomyces cerevisiae and identify yeast genes with a universal stress response as well as genes with a more specific reaction profile. In a breakthrough application of a high-throughput approach, Gasch et al. (2000) use expression profiling with microarrays to measure the changes, as a function of time, of almost all yeast genes, as a result of the exposure to a variety of stress conditions. They study the correlation between the response patterns of genes in single stress conditions by using clustering techniques. Here we study the sets of genes that seem to be persistently and strongly co-ordinated as part of the stress response mechanism, not restricted to a single specific condition.
For every stress condition we define the co-expression graph to be an undirected graph whose vertices correspond to genes, and the vertices of two genes are connected by an edge if their expression profiles are sufficiently correlated. Namely, the p-value of the Pearson correlation between the expression patterns of the two genes is statistically significant ($<0.01$). Two genes are said to be co-co-expressed in stress conditions $A$ and $B$ if their expression patterns in both time-courses correlate; alternatively - if they have an edge connecting them in both co-expression graphs. The $k$-stress persistence graphs are the intersection graphs of sets of $k$ co-expression graphs. By studying cliques in $k$-persistence graphs we impose very stringent conditions of co-ordination on sets of genes. They must all be highly correlated with each other in all conditions under consideration.
We extracted maximal cliques from all $k$-stress persistence graphs for $2\leq k\leq 12$, and studied the enrichment of two important types of features - transcription regulation factors which are known to regulate certain genes, and Gene-Ontology (GO) annotations that associate genes to functions or processes.
In yeast the activation of stress response has been associated with the activity of a small number of transcription factors (TFs) that regulate complex expression patterns of a large set of genes. Pilpel et.al. (2001) study the joint effect of TFs on gene expression in yeast, developing a framework for understanding the combinatorics of transcription regulation, and observe that a small number of TFs regulate response to stress. %Similar observation is made also by Hvidsten et al. (2005) develop a rule-base mechanism for %discovering binding-sites modules. predicting stress related co-expression. In this study we used TF to mRNA association data (Harbison et.al. 2004) to identify persistent cliques with enriched association to very few TFs. Our findings show that cliques are enriched only to six TFs (FHL1, RAP1, ABF1, SFP1, PAC and mRRPE) out of 113 taken from the data of (Hvidsten et.al. 2005, Pilpel et.al. 2001) when setting a statistical threshold of $10^{-6}$, in agreement with previous results. When raising the threshold to $10^{-3}$, only four more TFs (YAP5, SNT2, PDR1 and YDR026c) are enriched in the cliques.
We examined also the enrichment of cliques to pairs of TFs. Significant enrichment (p-value$<10^{-6}$) was observed only for a few pairs: (RAP1,FHL1), (RAP1,SFP1), (FHL1,SFP1), (PAC,ABF1), (PAC,mRRPE), and (ABF1,mRRPE). These pairs consist only of the six TFs reported to be enriched by themselves. Moreover, this set divides into two triplets, which appear to be synergistic. These results partially support the results of Pilpel et al.(2001), where PAC and mRRPE are reported to be synergistic. We suggest that similar relations exist for the rest of the pairs as well.
We also find persistent cliques to be functionally enriched using GO-term analysis. We computed the enrichment of cliques with the different GO-terms, and found that the cliques are enriched only with 19 GO-terms. In several cliques we were able to predict the GO-terms of un-annotated ORFs that reside in cliques highly enriched by a specific GO term.
This significant proximity between genes that are persistently co-expressed under several stress conditions might indicate that the cell is using genomic proximity to facilitate prompt co-activation as response to stress. We observe an interesting relationship between persistent cliques and the genomic location of member genes. Previous works indicated the correlation between genomic proximity and genes that participate in the same metabolic pathways (Overbeek et.al. 1999). We analyze the $k$-stress persistence graphs to study the relationship between co-expression and genomic proximity and show that not only that genes which are co-expressed tend to be closer on the genome, but that their proximity is higher in graphs that represent greater persistence (i.e. large $k$ values).
Finally, we seek stress persistent cliques that are enriched with specific motifs in the 3-UTR. Elements that affect mRNA stability and degradation were previously localized to certain regions in the 3-UTR (Chen and Shyu 1995, Stoecklin et.al. 2005). In addition to searching enriched simple sequence mitfs, we also require the motifs to be energetically accessible to binding when the mRNA is folded. We hypothesize that such motifs have important role in mRNA stability, and compare our findings to known motifs in that region.
Poster
Phospholipase D in Citrus EST database - Structure and function studies
Authors:
Martins, NF (AB3C)
Mehta, A (AB3C)
Short Abstract: Phospholipases D in plants are involved in several cellular responses. The in silico analyze of CitrusESTs database revealed 457 sequences related to phospholipases as 13 contigs and 12 singlets. A theoretical model, validated by Procheck, revealed common domains and therefore functions. It is the first evidence of PLD in Citrus.
Long Abstract:
Phospholipases D in plants are involved in cellular responses, as growth, development, stress and defense. The in silico analyze of Citrus ESTs database revealed 457 sequences related to phospholipases as 13 contigs and 12 singlets. The homology modeling of a PLD from Citrus revealed common structures and therefore functions. The model was validated by Procheck server (http://biotech.ebi.ac.uk:8400/cgi-bin/sendquery). Our results suggest that the phospholipase D shares structure-function relationship and perhaps same reaction mechanism. Structural analysis suggests that the occurs via phosphohistidine intermediate and provide the identification of a catalytic water molecule. It is the first evidence of PLD in Citrus.
Poster
Dr.
Authors:
Paula Regina Kuser Falcão (Embrapa-CNPTIA- Bioinformatics Lab)
Goran Neshich (Embrapa-CNPTIA-Bioinformatics Lab)
Anete Pereira de Souza (Universidade Estadual de Campinas, Inst. de Biologia, Dept Genética)
Short Abstract: A structural analysis of the stress-related protein (or chaperonin) smHSP17,9 kDa from the orange phytopathogen Xylella fastidiosa (9a5c strain) was performed and it revealed us the ability of this protein to form dodecameric complexes.
Long Abstract:
Molecular Modeling Of A Small Heat-Shock Protein From The Phytopathogen Xylella Fastidiosa.
Susely F. S. Tada1, Paula R. Kuser-Falcão2, Goran Neshich2, Anete P. Souza1.
1CBMEG, State University of Campinas, P.O. Box 6010, 13083-970, Campinas, SP, Brazil. 2EMBRAPA Informatics for Agropecuary. P.O. Box 6041, 13083-970, Campinas, SP, Brazil.
E-mail: susy_fs@yahoo.com.br
Protein smHSP 17,9 kDa (or orf XF2234) belongs to the class of small heat-shock proteins (smHSP's), which is overexpressed in cellular environment during episodes of stress or still during the sporulation of Xylella fastidiosa bacterium. The complete genomic sequence from this phytopathogen was elucidated by a Brazilian Consortium (ONSA) [1] and at this time, several approaches related to understanding of its pathogenicity mechanisms in order to fight this bacteria are in course. This protein possess in its extremity C-terminal a domain known as -crystalline, of about 132 amino acids, which possess similarity with others smHSPs. Homologous proteins had been crystallized and they had their structure 3-D resolved [2,3,4]. The most similar homologous (35% identity) to protein 17,9 is the smHSP 16,4 kDa of the archeobacteria Methanococcus jannaschii [2] which forms multimeric complexes. Perhaps such arrangement is the steadiest conformation for this protein. The -crystallin domain is important for the association of proteins, for termotolerance and the chaperone activity. The analyses using circular dicroism indicate that its secondary structures are composed mainly of beta sheets and that its C-terminal is highly flexible [5]. Despite the interesting properties of smHSPs and its important role in the folding mechanism of proteins, it has little structural information of this protein family. This work elucidated a structural model of a new smHSP of the phytopathogen Xylella fastidiosa by molecular modeling. The model was constructed by comparative modeling with the crystallographic structure of smHSP from Methanococcus jannaschii [2].
A preliminary analysis of the model, with the module Java Protein Dossier (JPD) [7] of STING software [6], showed that some amino acids have high energies of contact and could present some function in the functionality of association with other proteins. Some of these amino acids (Asp-23 and Glu-27) found in the first helix of the N-terminal, are in accessible areas of proteins and they are not part of the characteristic alpha-crystalline domain of this protein family. Amino acids Asp-65, Asp-68, Arg-84, Glu-87, Arg-100, His-106, Arg-108, Asp-116, and Arg-136 are in the central part of the protein and they vary with respect to the accessibility. Besides the molde obtained by comparative modeling, it was possible to generate a model for the multimeric complex formed by smHSP 17,9. In accordance to other resolved structures of chaperonins, this molecule exhibits a dodecameric array of its subunits (or monomers) characterized like two superimposed rings that forms a central pore (Figure 1).
Finally, this work aimed to construct a model of the three-dimensional structure of the new small heat shock protein (smHSP 17,9 kDa) from Xylella fastidiosa. An important observation can be drawn about the oligomeric model is that the central pore formed by the complex of the 12 subunits: probably, during the episode of cellular stress, the protein to be protected of a possible denaturation advances inside this pore, being involved for the complex of heat-shock proteins, which will play its protective function inside the cell. Obtaining structural information on this protein, one of the several approaches resulted of the Brazilian Consortium for Xylella fastidiosa Sequencing Program, may help in the process that aims the combat to this phytopathogen, which still causes innumerable damages in the of orange plantations and great economic losses in Brazil, the major world-wide producer of this fruit.
References:
[1] Simpson AJG et al (2000) The genome sequence of the plant pathogen Xylella fastidiosa. Nature 406:151-157.
[2] Kim KK, Kim R, Kim S-H (1998) Crystal structure of a small heat-shock protein. Nature (letters) 394:595-599
[3] van Montfort RLM, Basha E, Friederich KL, Slingsby C, Vierling E (2001) Crystal structure and assemby of a eukaryotic small heat shock protein. Nature Struct. Biol. (letters) 8:1025-1030
[4] Kennaway CK, Benesch JLP, Gohlke U, Wang L, Robinson CV, Orlova EV, Saibil HR, Keep NH (2005) Dodecameric structure of the small heat shock protein ACR1 from Mycobacterium tuberculosis J. Biol. Chem. 280:33419-25
[5] Neshich G et al (2003) STING Millennium: a Web based suite of programs for comprehensive and simultaneous analysis of protein structure and sequence. Nucl. Acids Res. 31:3386 - 3392
[6] Neshich G et al (2004) JavaProtein Dossier: a novel web-based data visualization tool for comprehensive analysis of protein structure. Nucl. Acids Res. 32 (Web Server issue):W595-W601.
Poster
Molecular Modeling Of A Small Heat-Shock Protein From The Phytopathogen Xylella Fastidiosa.
Authors:
Paula Regina Kuser Falcão (Embrapa-CNPTIA- Bioinformatics Lab)
Goran Neshich (Embrapa-CNPTIA-Bioinformatics Lab)
Anete Pereira de Souza (Universidade Estadual de Campinas, Inst. de Biologia, Dept Genética)
Short Abstract: A structural analysis of the stress-related protein (or chaperonin) smHSP17,9 kDa from the orange phytopathogen Xylella fastidiosa (9a5c strain) was performed and it revealed us the ability of this protein to form dodecameric complexes.
Long Abstract:
Protein smHSP 17,9 kDa (or orf XF2234) belongs to the class of small heat-shock proteins (smHSP's), which is overexpressed in cellular environment during episodes of stress or still during the sporulation of Xylella fastidiosa bacterium. The complete genomic sequence from this phytopathogen was elucidated by a Brazilian Consortium (ONSA) [1] and at this time, several approaches related to understanding of its pathogenicity mechanisms in order to fight this bacteria are in course. This protein possess in its extremity C-terminal a domain known as -crystalline, of about 132 amino acids, which possess similarity with others smHSPs. Homologous proteins had been crystallized and they had their structure 3-D resolved [2,3,4]. The most similar homologous (35% identity) to protein 17,9 is the smHSP 16,4 kDa of the archeobacteria Methanococcus jannaschii [2] which forms multimeric complexes. Perhaps such arrangement is the steadiest conformation for this protein. The -crystallin domain is important for the association of proteins, for termotolerance and the chaperone activity. The analyses using circular dicroism indicate that its secondary structures are composed mainly of beta sheets and that its C-terminal is highly flexible [5]. Despite the interesting properties of smHSPs and its important role in the folding mechanism of proteins, it has little structural information of this protein family. This work elucidated a structural model of a new smHSP of the phytopathogen Xylella fastidiosa by molecular modeling. The model was constructed by comparative modeling with the crystallographic structure of smHSP from Methanococcus jannaschii [2].
A preliminary analysis of the model, with the module Java Protein Dossier (JPD) [5] of STING software [6], showed that some amino acids have high energies of contact and could present some function in the functionality of association with other proteins. Some of these amino acids (Asp-23 and Glu-27) found in the first helix of the N-terminal, are in accessible areas of proteins and they are not part of the characteristic alpha-crystalline domain of this protein family. Amino acids Asp-65, Asp-68, Arg-84, Glu-87, Arg-100, His-106, Arg-108, Asp-116, and Arg-136 are in the central part of the protein and they vary with respect to the accessibility. Besides the molde obtained by comparative modeling, it was possible to generate a model for the multimeric complex formed by smHSP 17,9. In accordance to other resolved structures of chaperonins, this molecule exhibits a dodecameric array of its subunits (or monomers) characterized like two superimposed rings that forms a central pore.
Finally, this work aimed to construct a model of the three-dimensional structure of the new small heat shock protein (smHSP 17,9 kDa) from Xylella fastidiosa. An important observation can be drawn about the oligomeric model is that the central pore formed by the complex of the 12 subunits: probably, during the episode of cellular stress, the protein to be protected of a possible denaturation advances inside this pore, being involved for the complex of heat-shock proteins, which will play its protective function inside the cell. Obtaining structural information on this protein, one of the several approaches resulted of the Brazilian Consortium for Xylella fastidiosa Sequencing Program, may help in the process that aims the combat to this phytopathogen, which still causes innumerable damages in the of orange plantations and great economic losses in Brazil, the major world-wide producer of this fruit.
References:
[1] Simpson AJG et al (2000) The genome sequence of the plant pathogen Xylella fastidiosa. Nature 406:151-157.
[2] Kim KK, Kim R, Kim S-H (1998) Crystal structure of a small heat-shock protein. Nature (letters) 394:595-599
[3] van Montfort RLM, Basha E, Friederich KL, Slingsby C, Vierling E (2001) Crystal structure and assemby of a eukaryotic small heat shock protein. Nature Struct Biol (letters) 8:1025-1030
[4] Kennaway CK, Benesch JLP, Gohlke U, Wang L, Robinson CV, Orlova EV, Saibil HR, Keep NH (2005) Dodecameric structure of the small heat shock protein ACR1 from Mycobacterium tuberculosis J Biol Chem 280:33419-25
[5] Neshich G et al (2003) STING Millennium: a Web based suite of programs for comprehensive and simultaneous analysis of protein structure and sequence. Nucl Acids Res 31:3386 - 3392
[6] Neshich G et al (2004) JavaProtein Dossier: a novel web-based data visualization tool for comprehensive analysis of protein structure. Nucl Acids Res 32 (Web Server issue):W595-W601.
Poster
Characterization of gene expression during erytrhoid differentiation by Serial Analysis of Gene Expression (SAGE)
Authors:
Anderson F. Cunha (Universidade Estadual de Campinas- Hemocentro)
Ana Flávia Brugnerotto (Universidade Estadual de Campinas- Hemocentro)
Adriana SS Duarte (Universidade Estadual de Campinas- Hemocentro)
Gustavo GL Costa (Universidade Estadual de Campinas- Hemocentro)
Marcelo F Carazolle (Universidade Estadual de Campinas- Instituto de Biologia)
Gonçalo A G Pereira (Universidade Estadual de Campinas- Instituto de Biologia)
Fernando Ferreira Costa (Universidade Estadual de Campinas- Hemocentro)
Short Abstract: SAGE enables the analysis of thousands of genes and a total quantification of each transcript. We used SAGE to quantify expression profiles during the differentiation of human erythroid cells. Results may contribute to the comprehension of erythroid differentiation and identification of new targets genes involved in some erythroid diseases.
Long Abstract:
Erythroid differentiation is a dynamic and complex process in which a pluripotent stem cell undergoes a series of developmental changes that commit it to a specific lineage. These alterations involve changes in gene expression profiles. Extensive studies have led to a considerable understanding of the cellular and molecular control of hemoglobin production during red blood cell differentiation, however, a complete understanding of human erythropoiesis will require a robust description of the entire transcriptome of these cells during differentiation. From a global point of view of cell metabolic regulation, where genomic information could be complemented with gene expression, the use of methods that enable quantification of the entire transcriptome of red blood cell during the differentiation is of great importance. Serial analysis of gene expression (SAGE), a technique developed by Velculescu et al. (1995) [1] enables the analysis of thousands of expressed genes and a total quantification of each transcript. In this study, we used SAGE to quantify the gene expression profiles during differentiation of Human erythroid cells in a two phase liquid culture. To do this, we performed SAGE experiments in cells collected at the beginning, immediately before the addition of erythropoeitin (0 hour), during the culture and at the end of the second phase of culture, i.e., 192 hours and 336 hours after the addition of this hormone, respectively. We generated, after automatic sequencing, a total of 19328 tags at 0 hour, 19783 tags at 192 hours and 19562 tags at 336 hours, representing 8497, 8482 and 8175 unique tags, respectively. In the 0 hour library, a high expression of ferritin genes and CD74 antigen gene was observed. The beta globin, gamma globin and ribosomal genes were the most expressed genes at 192 hours and at 336 hours library the most expressed genes were basically globin genes. To identify the genes differentially expressed between the libraries, we considered a P value < 0.01 and fold ≥ 5 as statistically significant. In the comparison of the 0 hour and 192 hours libraries, 179 differentially expressed transcripts were identified. From these genes, we found in addition to the globin genes, an up-regulation of several genes such as GATA-1, TPSB1, GSTM3, TRIP6, PRDX2. Genes such as CSTB, CAPG, PLA2G7 and IFI30In were found to be down-regulated. Comparing the 192 hour and 336 hour libraries, 103 differentially expressed transcripts were identified. The up-regulated genes were generally genes related to hemoglobin synthesis, such as ALAS2, a gene involved in the biosynthesis of the heme group and related to sideroblastic anemias and genes related to intracellular transport such as MSCP and NUDT4 genes. The functional classification of genes was performed according to the Gene Ontology Consortium using the GOLIAS (Gene Ontology Library Analyser for SAGE), a program developed in our laboratory that uses the Gene Ontology structure to provide integration and to automate the SAGE analysis. GOLIAS reports quantitative data regarding the sequenced tags, statistics and graph charts. The results showed that the global aspects of the transcriptome were similar during the differentiation for most of the genes and that a set of genes involved in the modification of erythroid cells during differentiation. Among these, we found genes involved in signal transducer activity, transporter activity, proteic activity and transcription regulator activity.
To the best of our knowledge, this is the first report where the entire transcriptome of the red blood cell is quantitatively assessed during differentiation. The results found in this study will contribute to the comprehension of erythroid differentiation and identification of new target genes involved in some erythroid diseases.
Supported by FAPESP
[1] Velculescu VE, Zhang L, Vogelstein B, Kinzler KW (1995) Serial analysis of gene expression. Science 270:484
Poster
Protein Structure Prediction Aided Text Mining for Functional Inference
Authors:
Charlie Strauss (Los Alamos National Lab)
Andreas Rechtsteiner (Los Alamos National Lab)
Short Abstract: Transitive annotation by sequence comparison fails for highly divereged. Instead one can predict the protein structure and then look for structural simmilarity to protiens of known function. We show that ambiguous transitive annotations can be clarified by comparison of the text mined from sequence and structure.
Long Abstract:
Each newly sequenced genome is, in due course, principally annotated by comparison of its sequences to previously annotated genomes. Typically 40 to 60% of a new genome can be reliably annotated in this fashion. However, this method is most successful for the genes we often care least about, placing a premium on methods that can annotate unusual or highly diverged sequences. That is, if an organism was chosen for sequencing based on it's unique characteristics, those special features are likely to have elements that are highly specialized and hence highly diverged from other genomes and only possessing weak, ambiguous, sequence similarity.
In this twilight recognition realm, structure based annotation can be useful. By prediction of a protein's approximate structure we can compare it's structure to proteins of known function. It turns out that the process is highly insensitive to the accuracy of structure prediction allowing for recognition even for sequences with large deletions and variations in their sequences. It has been shown previously that ab initio modeling and comparison methods like Rosetta and Mammoth are highly effective on structural variations beyond the limits of homology modeling and threading approaches.
Because it is less specific than sequence based annotation, it is useful to confirm putative transitive annotations by other means. We have been developing methods to screen genome-scale predictions in an automated fashion to selected candidates for human curation effort.
To approach this we compare sequence and structure based similarity measures for common features. The comparison measure we describe here is text based. We generate a body of text from sequence-based comparison to the non-redundant sequence database by selecting the literature associated with the top BLAST hits and extracting keywords. Then we do the same with structure comparison to SCOP families. We then apply a text key word similarity measure between each SCOP match and the ensemble of sequence-based key-words.
We report that putative annotation rankings based on this approach dramatically improve the annotation accuracy over either sequence or structure based annotation alone. This is demonstrated on large benchmark sets that are carefully screened for homolog removal to simulate highly diverged sequences.
Poster
Text Mining in Life Sciences – Examples from the Pharmaceutical Industry
Authors:
Romacker (CKM&TM Novartis Pharma AG)
Parisot (CKM&TM Novartis Pharma AG)
Vachon (CKM&TM Novartis Pharma AG)
Kreim (CKM&TM Novartis Pharma AG)
Grandjean (CKM&TM Novartis Pharma AG)
Short Abstract: Text Mining technologies are in daily use at Novartis Pharma AG. In our Poster, we present solutions that are requested by scientists from research. All requests share common requirements. We will show how these requirements are met and how we apply text mining technologies in custom-tailored solutions at Novartis.
Long Abstract:
Introduction
Text Mining technologies have matured in the recent years and reliable solutions are now in place. With Text Mining, we are able to automatically analyze and access the content of huge sets of unstructured data, i.e. usually free text, as provided by patents, data base entries, full text articles from scientific journals. In our company that works in the area of Life Sciences, Text Mining technologies are in daily use since about 6 years. There are several solutions in place for scientists who work in research (e.g. biologists, chemists, patent specialists). Our objective is to facilitate and accelerate the access to information that is spread over disparate internal and external knowledge repositories.
.
Methods
Our approach to Text Mining is based on a entity recognition for diseases, targets, products, genes, companies, people and geographic locations. We are applying a canonical workflow consisting of a sentence splitter, a tokenizer and entity recognition. Additionally, we are able to parametrize the entity recognition process in order to cope with particular problems in the biomedical domain (e.g. part of speech - “a” and “not” are genes). We are also using zoning, i.e. the identification of structures in unstructured texts, on our data. Zoning enables us to improve precision and to recognize other entities such as RefSeq IDs, UniProt IDs, Affymetrix Codes, patent numbers etc.
Entity recognition is usually performed on the fly and we do not store annotations together with the documents. The reason is that our terminology changes frequently. In order to extract information we maintain a huge terminology with more than 1,500,000 terms. Especially for genes where science is still evolving we have to adapt our terminology frequently.
Discussion
For our collaborators, Text Mining is a promising means to gather valuable information when the number of relevant documents exceeds the human reading capacity. For example, recurrent questions relate to our research document management system (RDS) where research related information is stored and valuable information is locked. How do I retrieve important information in almost 70,000 RDS documents with sometime more than 600 pages without having to read a large subset of them? How do I, for example, find colleagues working on a specific target and a related disease ?
We apply Text Mining in two major areas: dynamic concept extraction in a comprehensive knowledge portal called the GPS Navigator and custom-tailored solutions for specific information needs. The GPS Navigator comprises more than 50 different data sources that can be searched, navigated and accessed in a unique manner. Furthermore, the GPS Navigator offers tools for analysis (e.g. clustering, business intelligence). In our poster presentation we will focus on the second area that we call solution offerings.
It is a widely acknowledged hypothesis that Text Mining should be applied on a large scale to document collections such as PubMed to extract entities and relations between them. There exist a lot of applications that extract medical, chemical or biological concepts and – sometimes – relations between them. Interestingly, when we have meetings with scientists from research who have urgent information needs they are not really attracted by these kinds of annotations, with respect to their domain of expertise. There are mainly two reasons. First of all, the scientists know their domain of expertise very well and they to not find many novelties in the annotations. Secondly, they do not really trust annotations (both intellectual and from Text Mining). However, they are very much interested in the automatic annotations on data from research areas in which they are not expert. If we really look closer to what we extract it is quite poor compared to the rich statements in the text. With Text Mining, we expect to find novelties in the quantity of data and not in the quality of the extraction.
From our experience in various meetings with people from different disease areas, discovery technology, patent office etc, the information needs of scientists differ from the before mentioned large scale approach. Usually, they are centered around their domain of expertise and based on a detailed information model. We therefore need to adapt our applications to be responsive to such needs.
In the requests for text mining we encounter very similar settings. First of all, we have a focused information need related to a smaller set of terms or concepts – usually about 500 - (e.g. targets, compounds, diseases) and relations between them, (e.g. a target related to a disease). Secondly, we use these terms as input for retrieving relevant documents (patents, journal articles but also internal documents from RDS and others). However, identifying a term in a text is not the only indicator for relevance. It is even possible that a relevant term is not mentioned but derivable from a context which is, for example, the case for mutations in kinases. Finally, we apply our text mining tools and extract relevant facts and/or relations. A highly requested feature is the compilation of the results in a lean report or the provision of annotated texts since the scientists want to integrate the extracted knowledge into their application.
Another interesting feature is to apply data analysis/mining techniques on automatic annotations, thus allowing to discover trends in a large collection of data. Scientists express an interest in discovering things that they don’t know yet. Annotations as such may be not a surprise for an expert in the field, but connections between annotations may be and this brings often an added-value like e.g. find research areas connected to other research areas.
Conclusions
In our poster, we want to expose the processing pipeline and some successful examples where we can illustrate the paradigmatic information requests from our collaborators / internal partners. Additionally, we intend to demonstrate some business benefits for scientists working in the Life Sciences.
Poster
DISPARE: a DIScriminative PAttern REfinement algorithm using PWMs
Authors:
Isabelle da Piedade (University of Copenhagen, IMBF)
Dorota Retelska (University of Copenhagen, IMBF, Bioinformatics Center)
Peter Arctander (University of Copenhagen, IMBF)
Anders Krogh (University of Copenhagen, IMBF,Bioinformatics Center)
Short Abstract: We describe a novel algorithm: DIScriminative PAttern REfinement algorithm (DISPARE). It is an iterative weight matrix optimization method that aims to distinguish more efficiently the true TFBS sites from a negative control set. The main new feature of the method is the discriminative nature of the method. DISPARE will be applied to the increasing collection of known true positives set obtained by ChIP-chip experiments
Long Abstract:
Gene expression is controlled by combinatorial interaction of transcription factors (TFs) and their binding sites (TFBS) in DNA. They play a central role in most biological processes. Promoters contain multiple binding sites for different transcription factors that cooperate for controlling the initiation (or inhibition) of gene expression. TFs bind to short (typically 6-8 bp), degenerated DNA sequence patterns (or motifs) that make them difficult to identify. Understanding the regulation of transcription in higher eukaryotes is still a major challenge and the current algorithms are not able to clearly distinguish real TFBS from random look-alike sequences.
Position-weight matrices (PWMs) are widely used to represent transcription factor binding sites (TFBS) in gene promoter regions [1-5]. Although many give a high number of false positives due to their low information content, they are commonly used because no better alternative is known. The PWMs are calculated from position frequency matrices (PFMs) that contain the observed nucleotide frequencies at each position of the profile alignment of binding sites for a TF of interest. Most of the known TF PWMs are collected in the Transfac [6] and Jaspar [7] databases accompanied by the information of their genomic binding sites. However, redundant profiles in those databases complicate large scale study of transcription factor binding sites. Moreover, the matrices are often derived from a limited number of experimentally verified sequences, which leads to inaccurate representations. Today wet-lab technologies like chromatin immunoprecipitation (ChIP-chip) are available to identify true novel positives binding sites. ChIP-chip provides a large amount of data and therefore will allow the estimation of more precised PWMs.
We describe a novel algorithm: DIScriminative PAttern REfinement algorithm (DISPARE) for refinement of PWMs based on e.g. ChIP-chip data. It is an iterative weight matrix optimization method that aims to distinguish more efficiently the true TFBSs from a negative control set. The initial PWM may come from Transfac or Jaspar or any other source. In each iteration, the sequences are scanned with the PWM using a low cut-off. To determine the optimal cut-off, we score all sequences with the PWM and calculate a measure of over-representation for each threshold of the score. Then, we pick the cut-off with the highest over-representation of the positive versus the negative set and use all matches of the positive set above the cut-off to estimate a new PWM. Using this iterative method of finding optimal matrices, we converge on an improved PWM for the sites of interest or we keep the initial one in case of non-improvement. We are testing several possible measures of overrepresentation, such as chi squared and a binomial model. We are also investigating additional ways of optimizing the width of the PWM.
The main new feature is the discriminative nature of the method. DISPARE will be applied to the increasing collection of known true positives set obtained by ChIP-chip experiments [8]. Generating accurate PWMs will ultimately contribute to better understanding of eukaryotic transcriptional regulation.
[1]. Hertz G, Stormo G: Identifying DNA and protein patterns with statistically significant alignments of multiple sequences.
Bioinformatics 1999, 15 (7–8):563-77.
[2].Stormo G: DNA binding sites: representation and discovery.
Bioinformatics 2000, 16:16-23.
[3]. Staden, R. (1984) Computer methods to locate signals in nucleic acid sequences Nucleic Acids Res., 12, 505–519
[4]. Bucher, P. (1990) Weight matrix descriptions of four eukaryotic RNA polymerase II promoter elements derived from 502 unrelated promoter sequences J. Mol. Biol., 212, 563–578
[5]. Tsunoda, T. and Takagi, T. (1999) Estimating transcription factor bindability on DNA Bioinformatics, 15, 622–630
[6]. Matys V, Fricke E, Geffers R, Gossling E, Haubrock M, Hehl R, Hornischer K, Karas D, Kel A, Kel-Margoulis O, Kloos D, Land S, Lewicki-Potapov B, Michael H, Munch R, Reuter I, Rotert S, Saxel H, Scheer M, Thiele S, Wingender E. (2003). TRANSFAC: transcriptional regulation, from patterns to profiles.
Nucleic Acids Res, 31:374-8.
[7]. Sandelin A, Alkema W, Engstrom P, Wasserman W, Lenhard B.(2004). JASPAR: an open-access database for eukaryotic transcription factor binding profiles.
Nucleic Acids Res, 32:91-4.
[8]. Kuo, M. H., and C. D. Allis. (1999). In vivo cross-linking and immunoprecipitation for studying dynamic protein:DNA associations in a chromatin environment. Methods 19:425-433
Poster
WebCell: a web-based integrated environment for building, managing and analyzing cellular network models
Authors:
Dong-Yup Lee (Department of Chemical & Biomolecular Engineering, National University of Singapore)
Choamun Yun (Department of Chemical & Biomolecular Engineering, Korea Advanced Institute of Science and Technolog)
Ayoun Cho (Department of Chemical & Biomolecular Engineering, Korea Advanced Institute of Science and Technolog)
Sunwon Park (Department of Chemical & Biomolecular Engineering, Korea Advanced Institute of Science and Technolog)
Sang Yup Lee (Department of Chemical & Biomolecular Engineering, Korea Advanced Institute of Science and Technolog)
Short Abstract: We developed WebCell which is an integrated environment for managing quantitative and qualitative information on cellular networks, and for interactively exploring their steady-state and dynamic behaviors over the web. WebCell is accessible at http://webcell.org or http://webcell.kaist.ac.kr.
Long Abstract:
Kinetic modeling and simulation of biological systems are now widely employed by researchers with diverse backgrounds: the functions and characteristics of the living system can be elucidated in detail. For the researchers who are less familiar with the underlying computational methods, various software and computational environments have been developed for such modeling and simulation of biological systems as listed in the systems biology community (http://sbml.org). Nevertheless, only a handful of projects adopt the platform-independent web-based approach which is a desirable direction for simulation research and development. Towards this end, we developed a WebCell system which is an integrated environment for managing quantitative and qualitative information on cellular networks, and for interactively exploring their steady-state and dynamic behaviors over the web. WebCell is interfaced to provide a simple and comprehensive modeling environment, allowing users to efficiently create, visualize, simulate and store their reaction network models. The modeling capability is further enhanced by SBML support. Provided methods for analyzing the resulting models include, but not limited to, structural pathway analysis, metabolic control analysis (MCA), conservation analysis and time course simulation. For the tutorial purposes, a variety of model collections publicly available have been compiled. Thus, this comprehensive, web-accessible and integrative system not only serves as an educational system for revisiting publicly available kinetic models, but also provides the customized modeling environment for quantitatively and dynamically analyzing the cellular network. WebCell is accessible at http://webcell.org or http://webcell.kaist.ac.kr.
Poster
Integrated semantic web system for metabolome data analysis and dynamic modeling
Authors:
Wonjun Park (Bioprocessing Technology Institute)
Dong-Yup Lee (Bioprocessing Technology Institute & Department of Chemical & Biomolecular Engineering, National Uni)
Short Abstract: The proposed herein is an integrative semantic web system for metabolomic data management and kinetic modeling of biological systems. The current framework can be realized in the application where three major functional parts including semantic layer, data management, and modeling with parameter estimation are organized.
Long Abstract:
Recent advances in high-throughput experimental techniques are now allowing us to study various omics data sets for the global understanding of biological systems. Concurrently with the high-throughput experiments, it is increasingly accepted that in silico modeling and simulation improve our capability to elucidate the functions and characteristics of complex cellular systems: The cellular behavior of the systems can be analyzed and predicted under any perturbations. Thus, it is highly desirable to establish a systems biology platform for integrating both wet-experiments and in silico models at the systems level. Towards this end, we propose an integrative framework for defining, sharing and evaluating kinetic models and metabolomic data of the cellular systems by resorting to the semantic web which is an emerging technology for effective exchange of knowledge through explicit description of the structured data semantics. Metabolome data is one of front-end omics data, thus directly describing the phenotypic cellular behavior. In this framework, the metabolome data and constructed kinetic models are standardized by XML-based data formats. To describe their clear relationship, we propose resource description framework (RDF) and RDF scheme from the semantic point of view. Thus, such a semantic association of XML-based knowledge renders both metabolomic data and kinetic models interoperable. Based on the interoperable system proposed, kinetic parameters in the model can be efficiently and precisely estimated by fitting with targeted metabolite profiles which are quantified by metabolic target analysis after data acquisition and preprocessing. The current framework can be realized in the application where three major functional parts including semantic layer, data management, and modeling with parameter estimation are organized. In conclusion, a proposed semantic web system is the practical solution for the effective management of metabolome data and dynamic modeling of biological systems as a novel paradigm. The main focus of the current study was on the metabolome data, but the procedures similar to those adopted for managing and integrating heterogeneous biological data and for describing their semantic association with in silico models can be extended to any omics data through data standards and their relations.
|
|
Back |
|
|
|
 |
| | | | | | | | | | | | | | | | | | | | | | | | | | | | | | | | | |