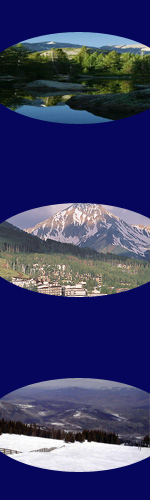 |
 |
|
Judith
Cohn, Ph.D.
Technical Staff Member, Bioscience Division
Los Alamos National Lab
Judith Cohn's CV (.pdf)
Title:
Dynamics Perturbation Analysis of SCOP Domains
We have
developed an algorithm that uses analysis of protein dynamics
to predict functional sites. The algorithm performs an approximate
version of Dynamics Perturbation Analysis (DPA), which can
predict ligand-binding sites in protein structures (D. Ming,
M.E. Wall. 2006. J Mol Biol 358:213). The present
algorithm decorates the surface of the protein with test points,
and uses approximate calculations of entropies to characterize
the degree to which each point perturbs the protein's thermal
vibrations. Residues near points that cause a large change
in entropy are predicted to reside in functional sites. We
used the algorithm to analyze more than 50,000 SCOP domains;
and predictions were integrated with residue-conservation
statistics obtained from the HSSP database. The analysis was
performed using a flexible, distributed software architecture
recently developed for this and other computationally intensive
tasks.
|
 |
|
Peter
Haug, M.D.
Senior Informaticist for Intermountain Health Care
and Professor
Department of Biomedical Informatics, University of Utah
Peter Haug's CV (.pdf)
Title:
Data Mining, Clinical Modeling and the Future of
Healthcare Computing
Abstract:
The evolution of the modern electronic health record (EHR)
has created an environment in which large amounts of medical
data are collected reflecting the character of disease processes
and the response of caregivers to these processes. This data
has historically had an episodic character but now is rapidly
becoming longitudinal in nature, allowing it to reflect the
course of health over time. The presence of this data facilitates
both the study of disease processes over time and the development
of novel, experience-based approaches to support the delivery
of care.
The data
available is captured both as structured, readily-computable
information and as textual reports, which require approaches
base on natural language processing (NLP) paradigms for effective,
computer use. In this presentation we will discuss our ability
to extract this data and to use it to develop computable models
of disease capable of informing care over time. The conjunction
of data mining and artificial intelligence techniques holds
promise to change the way we deliver, document, and evaluate
the clinical care process. |
 |
|
Kirk
E. Jordan,
Ph.D.
Emerging Solutions Executive IBM Strategic Growth Business/Deep
Computing
http://www-304.ibm.com/jct09002c/university/scholars/people/kjordan.html
|
 |
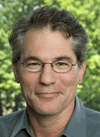 |
Eugene
Myers, Ph.D.
Group
Leader
Howard Hughes Medical Institute, Janelia Farms Research Campus
(HHMI JFRC)
http://research.janelia.org/myers/
|
 |
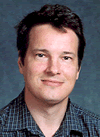 |
Andrey
Ptitsyn, Ph.D.
Assistant
Professor, Colorado State University
Andrey
Ptitsyn's CV (.pdf)
Title:
Life Works on AC Power
Multiple
studies indicate that 10-15% of all genes in the hypothalamus
and multiple peripheral tissues in mammals oscillate in a
daily (circadian) rhythm. In our recently published studies
we have applied three alternative algorithmic approaches to
identify circadian oscillation in metabolically active peripheral
tissues in mice and reported unexpectedly high number of oscillating
genes. Our studies also detect no steady non-oscillation fraction
of actively expressed genes. This leads to the conclusion
that the accepted null- hypothesis in tests for gene expression
periodicity is formulated on the unfounded assumption that
all genes display a default steady-line expression. We propose
a new approach that allows application of Digital Signal Processing
(DSP) algorithms separately to each phase class of genes.
Combined with Kolmogorov-Smirnov test this method identifies
circadian baseline oscillation in almost 100% of all expressed
genes. We conclude that such prominence of circadian oscillation
in gene expression must be taken into account in all studies
related to biological pathways. The importance of oscillation
in signal transduction is demonstrated on the example of insulin
signaling. This suggests that the loss of synchronization
is likely to be among the causes or aggravating factors in
metabolic disorders such as obesity and diabetes.
|
 |
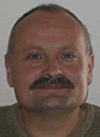 |
Heinrich
Roder, D. Phil.
Chief Technology Officer, Biodesix, Inc., Colorado
Heinrich
Roder's Biography (.pdf)
Title:
Comparative
Mass Spectrometry for Clinical Applications
Abstract:
The direct use of comparative mass spectrometry as
an unlabeled probe for the differentiation of disease states,
for prognostic stratification of patients according to treatments,
and for disease progression monitoring fasces special challenges
intricately connected with the physics of mass spectrometry
of biological molecules. In this presentation I will give an
overview of statistical techniques specific to the comparative
analysis of mass spectrometry data. |
 |
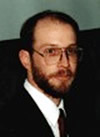 |
David
S. Wishart, Ph.D.
Department of Computing Science and Department of Biological
Science
University of Alberta David
S. Wishart's Biography (.pdf)
Title:
Metabolomics:
The Next Frontier for Bioinformatics?
Abstract:
Metabolomics is a newly emerging field of “omics”
research concerned with the high-throughput identification
and quantification of the small molecule metabolites in the
metabolome. Metabolomics is drawing considerable attention
these days because it has the potential to substantially improve
the speed and accuracy of many clinical tests and diagnoses.
Metabolomics shares many of the same computational needs with
other, much better established “omics” fields
such as genomics, proteomics and transcriptomics. All four
“omics” techniques require electronically accessible
and searchable databases, all of them require software to
handle or process data from their own high-throughput instruments,
all of them require laboratory information management systems
(LIMS) to manage their data, and all require software tools
to predict properties, pathways, relationships or functions.
Unfortunately, for metabolomics specialists, relatively few
of these essential tools or resources exist. Fortunately,
for bioinformaticians, this represents a wonderful opportunity
to apply what they have learned from other “omics”
endeavors to this newly emerging field. In this presentation
I will highlight some of the efforts we, and others are making
to bring modern bioinformatics practices to metabolomics –
including the development of public metabolomic databases,
the development of LIMS and the creation of software to interpret
metabolomic data. |
|
TOP |
| |