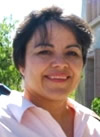 |
Assistant Professor
Arizona State University / Department of Biomedical Informatics
Biography (.pdf)
Title: Next-generation Extraction: Ad-hoc Knowledge Networks
Co-authors: Luis Tari, Jorg Hakenberg, Chitta Baral
Abstract: It takes a large computational effort to load, parse, and analyze (syntactically and semantically) millions of biomedical abstracts for information extraction tasks such as the extraction of relevant associations. Extraction of biomedical associations has received its fair share of interest from the research community in recent years, mostly centered around protein-protein interaction extraction, with numerous authors proposing slight variations of the same theme. All of these approaches perform "early binding" with respect to biomedical entities: documents are scanned for relevant entities ahead of extraction, tagging any that are of interest. Associations between the identified entities are then extracted and stored following the specific approach of each method, awaiting (future) queries from a mostly passive user. However, the knowledge about biological entities is always evolving: new synonyms are added, or new variants are discovered. With the traditional tag-ahead approach, any changes to the set of known entities or to the approach used to identify them or their associations require complete re-processing of the corpus, at a very high cost. Also, intended users (biological researchers) of the extracted associations have little say about what exactly constitutes an association or about what ancillary information is used to define them or needs to be extracted along with the association itself. We propose an alternative approach built around the idea of extracting first and then tagging, understanding "tagging" broadly as the action of identifying entities belonging to a class of interest or matching a specified pattern for an association with specific extractions, akin to binding a variable to a specific value. We call this method "late binding". Late binding allows new knowledge to be dynamically incorporated without having to re-process the corpus and without having to create ad-hoc extraction systems for every new association required. Using late binding, the process of parsing and grammatical analysis of the text is done only once, storing the resulting structural information in a database. For example, consider the following statement: The enzymes RgtA and RgtB, described in the accompanying article, catalyze GalA transfer to the Kdo residue, whereas RgtC is responsible for modification of the core mannose unit (PMID 16497671). While the RgtA, RgtB, and RgtC proteins would likely call the attention of any PPI interaction system, GalA (galacturonic acid) would not, since it is a sugar, not a protein, and the passage will most likely be ignored. With late binding, it would be possible to extract sugar-lectin associations "on the fly" and construct a specialized knowledge network with such associations by specifying the pattern of interest in a user-friendly query language. We have already implemented a prototype of this next-generation extraction system, and have completed processing and storage of all the abstracts in Medline with layered semantic and syntactic information.
|