ISMB/ECCB 2019 - Distinguished Keynote
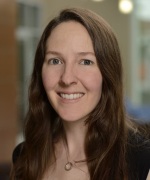
Alexis Battle
Associate Professor, Biomedical Engineering and Computer Science
John Hopkins University
Baltimore, United States
http://battlelab.jhu.edu
Time: Tuesday, July 23, 8:30 am - 9:30 am
Room: San Francisco
Abstract
Non-coding and regulatory genetic variation plays a significant role in human health, but the impact of regulatory variants has proven difficult to predict from sequence alone. Further, genetic effects can be modulated by context, such as cell type and environmental factors. We have developed computational approaches to model the effects of regulatory variation, including predicting the impact of rare regulatory variants on gene expression, modeling the interaction between environmental factors and genetic variation, and detecting regulatory effects that vary over time courses. I will present recent results evaluating the complex impact of both rare and common genetic variation on gene regulation in diverse contexts including different tissue types.
Rare genetic variation is abundant in the human genome and can contribute to disease risk, yet interpreting and predicting its functional effects remains a significant challenge for personal genomics. Recent work has shown that gene expression measurements can aid in evaluation of rare variants, and that rare variants are enriched near genes where an individual exhibits abnormal expression levels. Recently, we significantly expand the use of RNA-sequencing to guide interpretation of rare variation, extracting diverse signals of abnormal transcriptomic patterns including total expression, allele-specific expression, and alternative splicing. I will discuss the use of unsupervised probabilistic graphical models to scores rare variants from a personal genome by integrating information from genomic annotations along with all three signals from the personal transcriptome, ultimately predicting the probability a rare variant has a functional effect on each transcriptional signal. Our method outperforms methods based on genome sequence alone and improves over models where each transcriptomic signal is treated independently. Overall, we provide a comprehensive analysis of the impact of rare variation on gene expression and describe an integrated framework for personal genome interpretation.
Biography:
Alexis Battle is an Associate Professor of Biomedical Engineering and Computer Science at Johns Hopkins University, and a 2016 Searle Scholar. Her research group focuses on understanding the impact of genetic variation on the human body, using machine learning and probabilistic methods to analyze large scale genomic data. She is interested in applications to personal genomics, genetics of gene expression, and gene networks in disease, leveraging diverse data to infer more comprehensive models of genetic effects on the cell. She earned her Ph.D. in Computer Science in 2013 from Stanford University, where she also received her Bachelor’s degree in Symbolic Systems in 2003. Alexis spent several years in industry as a manager and member of the technical staff at Google, Inc.