Award Winners
Art & Science Award
Wikipedia Award
RCSB PDB Poster Prize
F1000Research Poster Award
Ian Lawson Van Toch Memorial Award for Outstanding Student Paper
Download Award Winner's as PDF
Wikipedia Competition
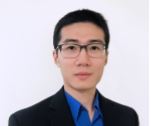
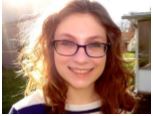
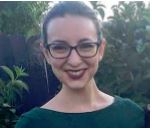
“Pfam”
The Garvan Institute of Medical Research Minard->o: How Napoleon Marched into Phospho-Proteomic Data
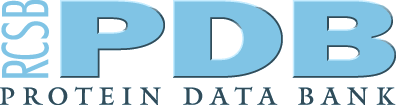
Next generation structure-based antibody drug design with ABodyBuilder and PEARS
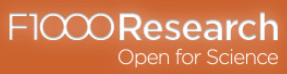
B-192: Kernelized Rank Learning for Personalized Drug Recommendation
Large-scale screenings of cancer cell lines with detailed genomic profiles against libraries of pharmacological compounds are currently being performed in order to gain a better understanding of the genetic component of drug response and to enhance our ability to predict drug sensitivity from genetic profiles. These screens differ from the clinical setting in which (1) medical records only contain the response of a patient to very few drugs, and in which (2) selecting the most promising out of all therapies is more important than accurately predicting the sensitivity to the given drug. Current regression models for drug sensitivity prediction fail to account for these two properties. We present a machine learning approach, named Kernelized Rank Learning (KRL), that ranks drugs based on their predicted effect per patient, circumventing the difficult problem of precisely predicting the sensitivity to the given drug. Our approach outperforms several state-of-the-art predictors in drug recommendation, particularly in a clinically-relevant case where few training data are available.
A-350: Generalized benchmarking of gene prioritization methods
B-276: Selection of Stable Biomarker Signature for Prediction of Metabolic Phenotypes
In biomarker research, the goal is to construct an accurate prediction rule on the basis of a small number of predictors to make it practical in the clinical setting. Such rule typically is used to determine presence of disease/malignancy, to stratify patients into subtypes or to select an optimal treatment option. Advances in high-throughput data generation have dramatically expanded the search space for biomarker discovery, making the selection of an optimal biomarker signature from large and noisy datasets challenging. In this project, we aim to benchmark the procedure of biomarker signature selection, using measurements of 35 murine strains from the BXD genetic reference strain panel [1]. Animals of each strain were respectively exposed to high-fat and chow diets [2], yielding 70 samples in total. We use 2100 liver proteins measured with SWATH-MS to predict seven metabolism-related continuous phenotypic traits: body weight, fat mass, lean mass, blood glucose and insulin levels, body temperature during the cold test, respiration volume. Some of these traits are strongly linked to liver biochemistry (fat, glucose), and some are, to our knowledge, independent (e.g. body temperature). Thus, we select biomarker signature of few proteins to predict the respective phenotype using several feature selection approaches. The ability of the model to generalize shows whether the model derived from the experiment can be ultimately translated to clinical setting. For each trait, we select best features multiple times using cross-validation. If the signatures for each sampling are similar (the signature is stable), the joint signature can be generalized to an independent population. Using various metrics, we estimate the stability of biomarker signatures for each feature selection approach. We show that the stability of the signature heavily depends on the relevance of the molecular profile to the phenotype it aims to predict. For our dataset, biomarker signature derived from liver proteome is stable for fat mass and glucose level, which are traits related to liver metabolism, and is highly unstable for body temperature. Thus, stability can be used to assess whether the biomarker signature is in principle identifiable from the given dataset.
A-124: WL Viewer: The Common Workflow Language Viewer
B-124: Computational Functional Annotation using Hierarchical Orthologous Groups in OMA
When loss-of-function is loss of function: Assessing mutational signatures and impact of loss-of-function genetic variants
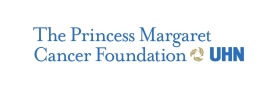
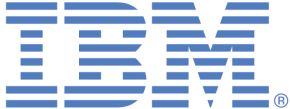